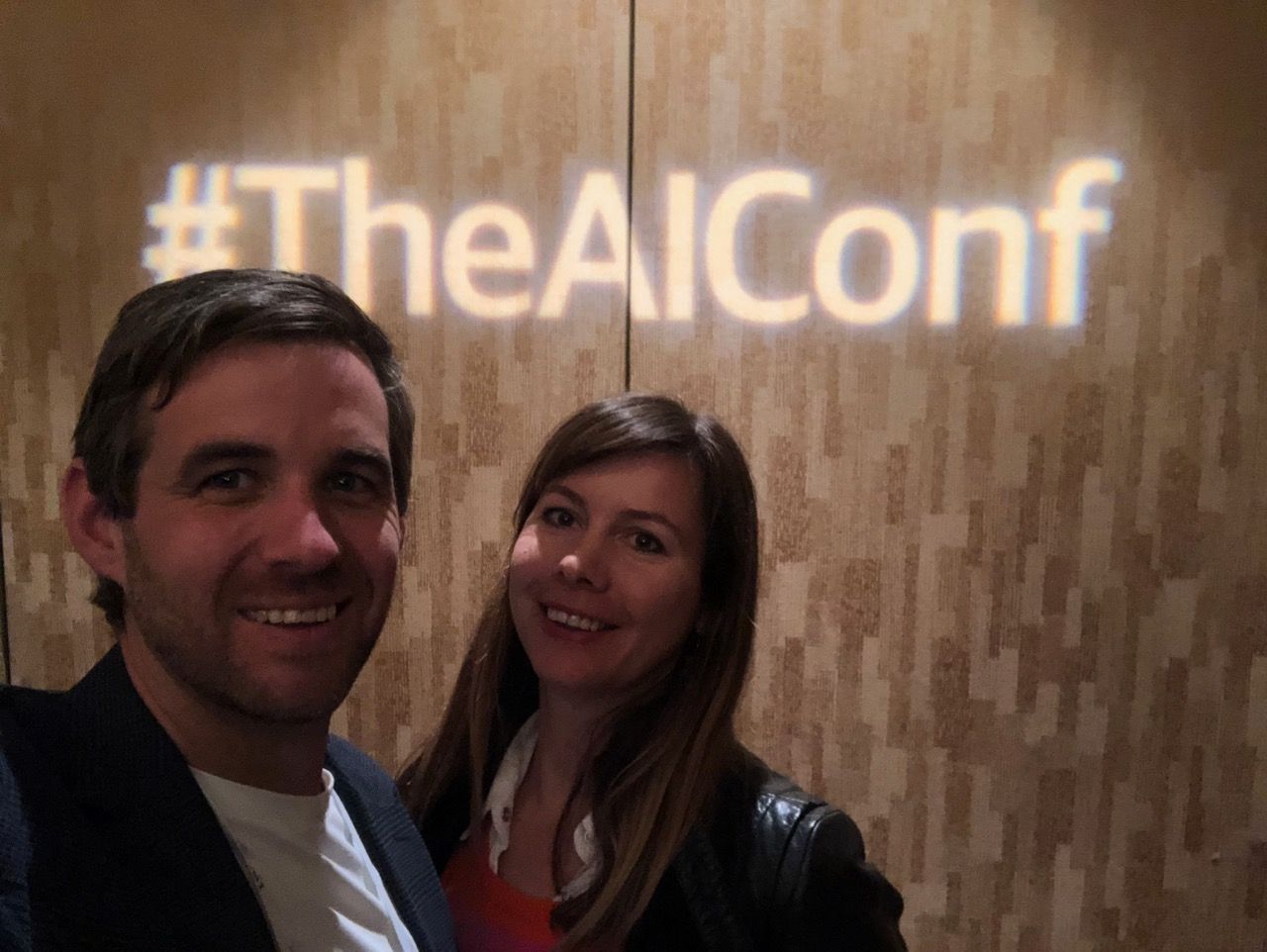
Commercializing AI research: Lessons learned and Thematic's journey
– “Hey, aren’t you the creator of Maui?”– A few months ago, someone I didn’t know approached me at O’Reilly’s AI conference.
– “Yes, I am”, I said, excited that someone still remembered my project, and a little sad too… It’s been almost 10 years since I’ve published Maui’s open-source code repository at the end of my PhD, and approximately 5 years since I stopped participating in its community forum.
This is a story of how instrumental Maui still was in creating Thematic, and how my co-founder Nathan Holmberg and I have commercialized AI research and built a SaaS company selling an AI solution over the past few years.
How NATO wanted to buy my software
Maui (named after New Zealand’s dolphin) really made waves. This open-source solution could find keywords in documents and match them to entries in controlled vocabularies like industry-specific taxonomies, or even Wikipedia. My PhD thesis proved that Maui could be as accurate as humans. Some people still use it, and some are even making money by using it.
I often thought about how to commercialize Maui myself. I even tried doing so by putting up a website and seeing who will reach out to buy. Several organizations reached out. The craziest request was from NATO. Turns out they have hundreds of scientists, and they needed to connect these scientists based on common topics in their research.
But Maui never became a real commercial project. Why?
Commercializing academic research is hard for many reasons. One of the reasons is that it is hard to find a link between technology and real-world business problems. When you start with technology, there are dozens of options for how one might use it, and you need to methodically discount each one of them until you find the right one.
How one meeting made me stop commercializing technology
I was in the process of exploring options for commercializing Maui when I stumbled upon an insight that urged me to start Thematic. I encountered a problem that I couldn’t solve with Maui, but I could solve it with my specialist knowledge in AI, and more specifically, Natural Language Processing and making sense of text.
I was in a meeting with a large company, who invited me to pitch Maui. There were three people in the room. Two men, who invited me, and a woman who came along because Maui sounded useful for her problem.
After I gave a demo, the men brainstormed about how Maui could be applied at that company. All great ideas.
– “When do you think you might start implementing something like this at your company?”, I asked.
– “In January or February”, one of the men said. It was June!
I turned around to the woman and asked her about her problem. She explained that the company receives a lot of Net Promoter Score (NPS) feedback that gauges how people feel about their brand and a recent interaction with their customer services. NPS is a KPI and she is looking for tools that glean insights into what’s driving NPS.
I knew straightaway that Maui couldn’t solve that problem, but there was another coding project I was working on at the same time. So, I asked her:
– “I might be able to help. When would you need this by?”
– “Ideally, last month! I’ve been looking for a solution for a long time. I reached out to an US company, but they never got back to us. Perhaps we are too small for them to matter.”
Needless to say that the contrast in these responses made me drop Maui altogether the following week. I decided to focus on my “other coding project”.
How we searched for patterns and gotchas
Because I run the NLP Meetup in my city, several other companies reached out to me around the same time asking whether there is a good solution for finding themes in customer feedback. They also used NPS surveys. Coincidence or a pattern? Anyway, I already started to code up a possible solution and my PhD knowledge was instrumental in finding the right approach.
By that time I also enlisted my co-founder Nathan to join this quest, and we both started working part-time on this project to continue validate our ideas further.
An important thing we did was to speak to as many people as we could connect with about what the right solution might look like. We spoke with marketing researchers, qualitative researchers, insights professionals, and quizzed them all on what are some of the gotchas, what do we need to consider to make this work and to sell it.
How commercial world is different from academic world, and how it’s also similar
These initial conversations were important, because you don’t usually talk to potential customers when you are working in academia. This just doesn’t matter. In academia, what matters is publications. And you get published when you beat state-of-the-art results with a novel approach. In academia, instead of talking to potential customers, you read papers and re-use other people’s data. Even if you improve the performance by 2-3% over the previous approach, it’s an achievement.
But in commercial world, usually, these 2-3% don’t matter. What matters is whether your solution solves a real company’s problem. Are companies aware of other solutions that exist in the market? Why are they not using them? How is your solutions different? Why should they buy from you?
We built Thematic from ground-up by commercializing AI research, but we started with a problem many companies were prepared to talk to us about. They talked to us because they struggled to find a viable solution. To find the right approach, we focused on recent advances of many academics in Natural Language Processing, not just mine.
Whereas a PhD is a lonely venture, starting Thematic meant that we were able to quickly get support from many people who believed in our vision and in us as founders. The support of our employees who are just as passionate about what we are creating is what I love about this journey the most.
And yet, surprisingly, the journey of starting a company has not been that dissimilar to doing a PhD. I often tell people: Having a PhD only proves that you are boring enough to work on an extremely narrow problem for 3 to 5 years. When you start a startup you also end up working on an extremely narrow problem:
how to create a solution to a specific problem that others will want to buy and how to reach these buyers in a cost-efficient and repeatable way.
Like a PhD researcher, you are also running experiments and testing hypothesis all the time. And it also usually takes 3 to 5 years to turn this startup into a company.
We are now midway in year 4 and it’s been one of the most exciting things I’ve done in life.
Stay up to date with the latest
Join the newsletter to receive the latest updates in your inbox.