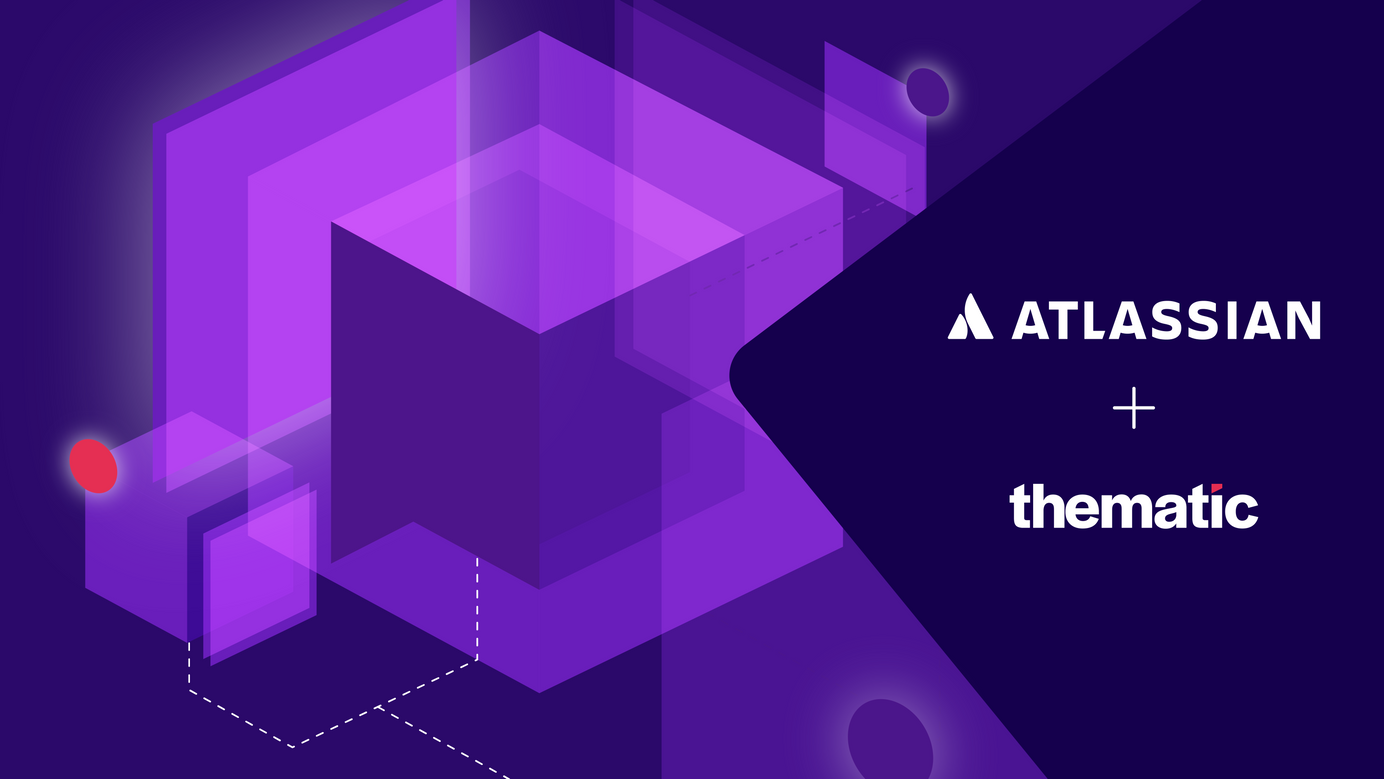
Harnessing NLP to address customer needs: Munna Ahaduzzaman at Atlassian
Atlassian has to make sense of disparate customer datasets across all of its products. With Thematic, they can drill down into the detail, finding exactly where a problem originates and what needs to be fixed.
Software engineer Munna Ahaduzzaman is chasing in-house solutions to a global problem. He is determined to help Atlassian - one of the world’s biggest software companies - better harness insights from a sprawling array of customer feedback.
Fortunately, the multilingual Bangladesh-born Texan resident seems to tackle challenges as efficiently as he traverses international borders. Munna collected his first computer science master’s degree while in Japan, where he also became a fluent Japanese speaker. His second master’s, in artificial intelligence (AI) and natural language processing, came courtesy of the prestigious Carnegie Mellon University in Pennsylvania. Eventually, he found his way to the Australian software development corporation Atlassian.
As principal software engineer within Atlassian’s technology innovation team, Munna is constantly hunting for new technology solutions to business process problems. That’s how he came to head the NLP vendor assessment project. His project aims to help Atlassians better understand what their customers want, where they are having problems, what could be done to improve any pain points.
Although Atlassian was already gathering customer insights when Munna started investigating, he knew there was room for improvement. He and his team wanted to help the company – and its software engineers in particular - harness NLP to delve far more deeply into customers’ needs and wants.
“We had a solution but we were looking for something better,” he says. “We are a very customer-focussed company so we wanted to be able to really hear and understand and identify those insights. We wanted to make sure the solution we had was best in class.”
The issue, Munna says, is that Atlassian needs to make sense of wildly disparate customer datasets collected from a range of sources, across all of its products and from an ever-growing list of newly-acquired companies.
Feedback can include comments from a dropdown feedback box, assorted rating and ranking methods, information from company-generated surveys and public forums as well as product-specific support tickets.
Some questionnaires require a few short emotive responses while others are structured to automatically generate successive questions, depending on the answers supplied.
“If you have a star rating from one product and a feedback form from another saying you are happy, angry or sad, how do you quantify the overall result? The problem in any company is, since we have so many insights and so many different types of data, how do you aggregate the insights to gain clear direction from them?”
So the hunt began. Extensive market research netted a long list of hopefuls, interviews led to a shortlist and eventually three vendors were invited to participate in a ‘proof of concept’ process that would span 10 weeks.
In the end, after finalists had completed a proof of concept (POC) process, Thematic emerged the clear winner.
“The way it themes the data, it makes sense,” he says of Thematic’s rise to the top.
“It relates to real life, it nicely captures the feedback, it identifies themes and names that are very close to the way customers express themselves in feedback. And the accuracy is good.”
Munna and his team built a data pipeline to facilitate the POC process. Once Thematic was on board, there was plenty of to-ing and fro-ing on both sides to make changes, add features, improve integration and transform the trial into a practical, production-ready solution.
He likes the way Thematic drills down into the detail, to show exactly where a problem originates and what needs to be fixed. For example, it can show negative sentiment emerging in relation to navigation within a particular product, then pinpoint whether customers are annoyed by a scrolling feature and whether it’s in the top right or lower left corner of the screen.
“We can quickly see, what do we lack? What are the features we need to improve? Why should we put more focus or energy into one area? How should we prioritise?"
“Without these insights, it’s impossible. It’s hard to build something the customers want and without insights, we don’t know what they want.”
Munna also likes the Thematic augmented AI, which means a hierarchy of themes are rapidly, automatically generated by AI. With augmentation capabilities, Atlassian employees can make alterations to the way themes are structured. The Thematic AI system learns from the changes and incorporates these into any future analysis.
“There’s not so much human involvement as part of the constant modification. So slowly, slowly, we integrated our systems with them. And we improved the processing pipeline because we made it scalable.”
While the initial scoping project has focussed on analysing just three data sources, the full roll-out will add many more.
“We built a platform that means it is now easier to add new kinds of feedback data, we don’t have to write another integration every time. ”
Munna says his own background in language processing and artificial intelligence has helped ensure a cutting edge solution.
His previous role at Atlassian, building customer support software, has also proved beneficial. “It’s the perfect project for me, personally. I have a lot of attachment to it.”
These are still early days in a long-running project but so far, he says, it’s looking good. The next step will involve feeding community-sourced data into Thematic and to continue adding yet more types of community feedback into the system.
“Once you have themes, likes and scores, once the data is there, you have the freedom and power as a product manager to slice and dice the data. You can look at it in various ways, it’s easy for them to analyse it from various angles.”
Thematic is already showing product managers what to focus on. Ultimately, it will enable them to reach out to customers with updates on particular issues and solutions that are in the works.
“It’s closing the loop, saying we heard you. We got your feedback and we’re working on it. Give them an idea when the bug is going to be fixed.”
“Our research and insight team and product managers, they’re learning things they didn’t know before. The features, the bugs, the improvements, all these things they’re finding out in a structured way. They’re excited about it, which is very inspiring. We’re on the right track.”
Thematic Newsletter
Join the newsletter to receive the latest updates in your inbox.