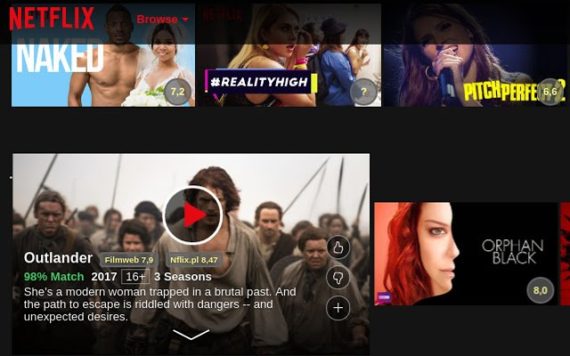
Real-world examples of AI analytics in action
With the help of artificial intelligence (AI) technologies, businesses today can easily predict what customers want and respond accordingly. A huge benefit of using AI is just this ability for more accurate prediction of customer needs.
What’s sophisticated AI look like in the real world?
As Kindzierski writes for PR Newswire, “Thanks to the rise of digital channels and the advancement of AI and machine learning technology, we now have the ability to predict (with a high level of confidence) the right answer to a customer’s inquiry in any digital channel: email, SMS, chat, or social. This advancement in technology will make our agents much more efficient. No more looking up answers, spell checking, tagging or categorizing conversations.” (PR Newswire).
There’s no question that advanced data analytics tools help businesses reveal important insights and gain a competitive edge. As customer experience analytics matures, it’s becoming increasingly predictive and focused on personalization.
A sophisticated data analytics tool can make predictions, or generate recommendations based on information gathered, thereby giving human support reps and chatbots more background and context on each individual customer they’re servicing. In addition, analytics play a significant role in increasing productivity. For example, speech analytics can detect long conversational pauses or points in the conversation when the customer has to repeat responses (PR Newswire).
Predictive Analytics to Reduce Churn and Increase NPS
Predictive analysis is used in companies all over the world. For example, Sprint uses AI to create better customer experiences. In 2014, they had a customer churn rate of 2.3%, double that of its biggest competitors. As for customer service strategies, the company was relying on CX agents to analyze their data, thereby allowing for human error and trusting in the agent’s own judgment.
Before employing AI such as predictive analytics, the CX agent would sift through numerous offers, picking the best offer while the customer was waiting on the phone. As we know, customers are loathed to wait, and the longer they wait the more aggravating it can be.
Sprint realized this was not ideal so, they implemented a data solution where predictive and self-learning analytics helped find customers at risk of churn. They also proactively provided personalized retention offers to customers. The results were staggering. Sprint reduced customer churn by 10% to “historic lows”, and increased its Net Promoter Score (NPS) by 40%. Additionally, Sprint boosted customer upgrades by eight times, convincing 40% more customers to add a new product line, and improving overall customer service agent satisfaction (Forbes, 2017).
Another example of real-world AI in action is one telecom company which found that when a customer defected, others in that customer’s calling circle, friends and family, were at elevated risk of defection also. Their predictive analytics model helped them flag those situations in real-time and trigger relevant actions based on these predictions (Bain, 2017).
Netflix and Spotify autonomously figure out customer’s likes and dislikes
AI algorithms underpin Netflix services, where if a customer is browsing a different movie category on Netflix than they were before, new recommendations need to be served up to entice the customer to watch a particular movie and continue being engaged with Netflix (Bain, 2017).
Content services get better and better at figuring out what the customer likes and dislikes through algorithms, such as Discover Weekly playlists on Spotify.
Personalization through AI
Raconteur brings us an interesting example from US software company Dynamic Yield, which uses an advanced machine-learning engine to build actionable customer segments to personalize the customer experiences and services.
Using AI, marketers can to talk to each of their customers in a tailored and relevant way and thus build stronger relationships, which will yield more ROI over time. “Dynamic Yield is working towards being the leader in AI-based personalization”, says founder and chief technology officer Omri Mendellevich.
Currently, the technology is used to empower the decision-making process for marketing purposes.
“We understand how specific segments within your customer base interact with specific variations of the web experience,” says Mr. Mendellevich.
“You can try different designs of a purchase stage on your website. Since we have a lot of audiences in the system, we can see through our machine-learning how each segment interacts and engages with the different content. We learn with every user response. This means you actually get traffic directed to the right segment with the right variation in real time.”
In this way, behavioral data reflecting real customer actions trumps traditional consumer panels, where customers tell you how they think they might behave in the future (Raconteur, 2017).
In a similar vein, ContentSquare, a user experience analytics, and optimization business added an AI layer in the form of a bot named Arti. This bot captures website user engagement data to help them ensure every section of content on their sites is as potent and profitable as possible (Raconteur, 2017).
Watch this space for our upcoming blog post as a great example of prescriptive analytics.
Stay up to date with the latest
Join the newsletter to receive the latest updates in your inbox.