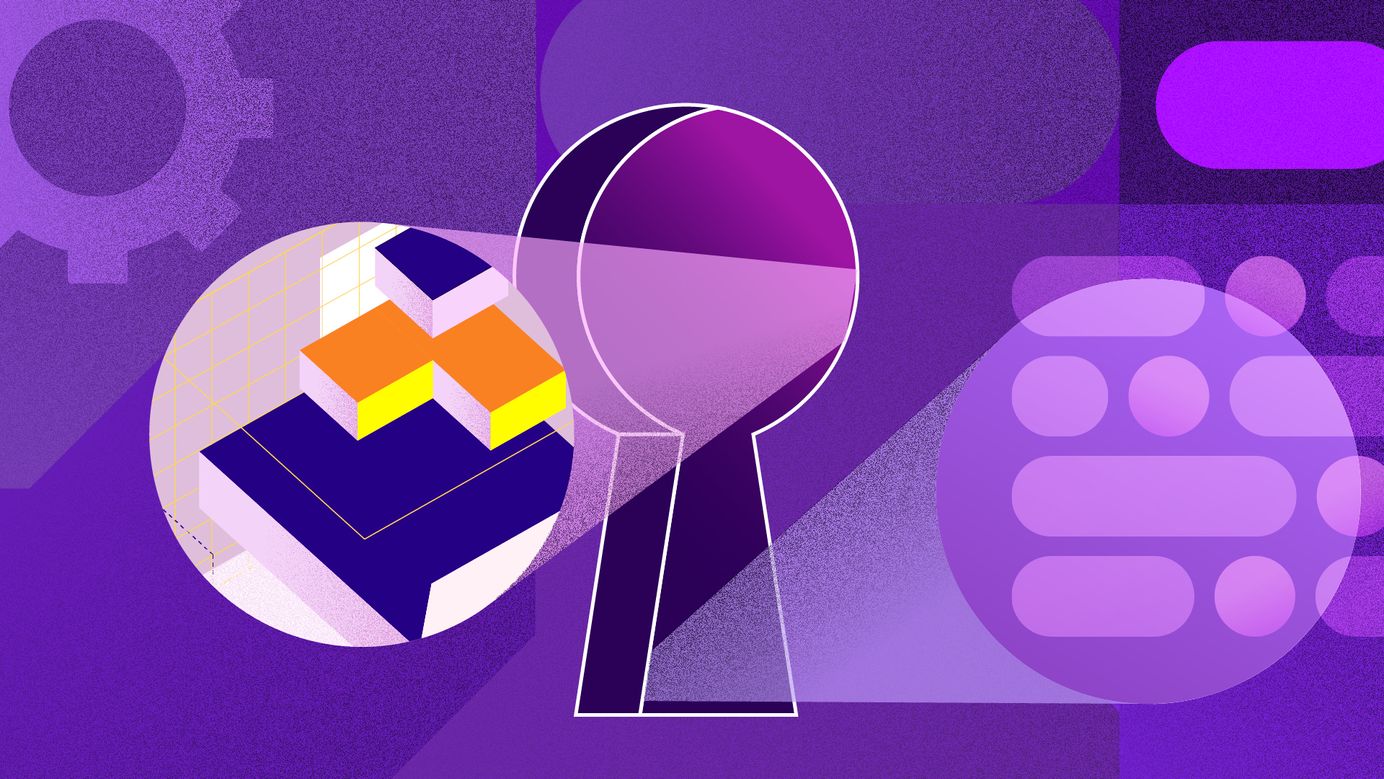
Mastering Text Analytics: Overcoming Challenges to Unlock Game-Changing Insights
Explore the top challenges in text analytics and discover strategies to overcome them effectively.
If you feel like you are drowning in a sea of customer feedback and struggling to pinpoint the real concerns, then you are experiencing text analytics challenges.
Same struggles, different faces—you're just one of the many in this crowd. Sifting through endless feedback can feel like an impossible task. You're sitting on a customer insight goldmine if only you dig deep enough. Within that data sprawl lies the clues to understanding what makes your customers happy, angry, and everything in between.
Did you know that approximately 80% to 90% of big data is unstructured, encompassing emails, social media posts, and customer reviews? This vast amount of unstructured data presents significant challenges for businesses extracting meaningful insights.
Insights can easily slip through the cracks without the right tools, which means missing out on chances to improve and grow. That’s why text analytics is important.
Let’s take a look at some of the common text analytics challenges.
Unstructured Data and Its Problems
One of the most significant text analytics challenges involves handling unstructured data. Customer feedback comes in diverse forms—emails, chat logs, social media posts, and product reviews—making it difficult to standardize and analyze effectively.
Unlike structured data that fits neatly into databases, unstructured text requires extensive pre-processing to clean, organize, and prepare it for analysis. This is why qualitative data analysis is tricky. The complexity even increases as the volume and variety of data sources grow, making it harder to derive consistent and reliable insights.
Solution:
- Employ advanced natural language processing (NLP) techniques to clean and standardize data.
- Implement machine learning (ML) algorithms to categorize and structure different data types.
- Automate the pre-processing stage using data pipelines to ensure data consistency and accuracy.
A case in point is Vodafone New Zealand’s use of Thematic. The company streamlined its feedback analysis process by using the NLP-powered thematic analysis software. They transformed messy, unstructured customer reviews and chat logs into clear categories, uncovering trends like recurring frustrations and positive experiences that needed immediate attention. This approach significantly improved their customer satisfaction metrics.
NLP Models and ML Algorithm Biases
Biases in NLP models and ML algorithms can distort analytical outcomes and lead to misleading insights. These biases often arise from imbalanced training data that fails to capture the full spectrum of customer perspectives. As a result, certain groups or sentiments may be underrepresented or misinterpreted, leading to flawed business decisions.
Solution:
- Train models on diverse and representative datasets to minimize inherent biases.
- Conduct regular audits and retraining of models to detect and correct biases.
- Incorporate explainable AI frameworks to understand and monitor how models make decisions.
Atom Bank reduced bias in their sentiment analysis by using Thematic's explainable AI framework. This allowed them to identify gaps in their training data and adjust their approach, ensuring feedback from underrepresented customer groups was accurately captured and used to improve their services.
Beyond NLP: How LLMs Transform Text Analytics
Is your Text Analytics solution still relying on B-Grade NLP? Discover how large language models are revolutionizing text analytics, offering deeper insights than traditional NLP approaches.
- Understand key NLP limitations and LLM advantages
- View real-world results of AI-driven text analytics
- Learn how self-learning AI eliminates manual updates
- Cut analysis time from weeks to minutes
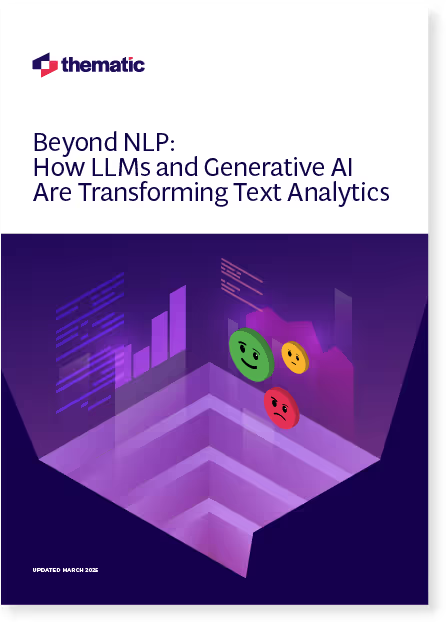
Processing Multilingual Text Data
For global businesses, customer feedback often spans multiple languages, dialects, and cultural contexts. Processing multilingual data introduces complexities because direct translations can alter meaning, tone, and sentiment. This challenge of text reduces the accuracy of sentiment text analysis, topic modeling, and entity recognition.
Solution:
- Utilize multilingual NLP models capable of handling diverse languages.
- Implement context-aware translation models to preserve original sentiment and context.
- Customize models to recognize region-specific language nuances, slang, and expressions.
Imagine translating a heartfelt message into another language, only to realize the translation loses its emotional impact. Multilingual NLP tools in text analytics tools act like a skilled interpreter who not only translates words but also preserves the sentiment behind them, ensuring your customers’ true feelings are understood.
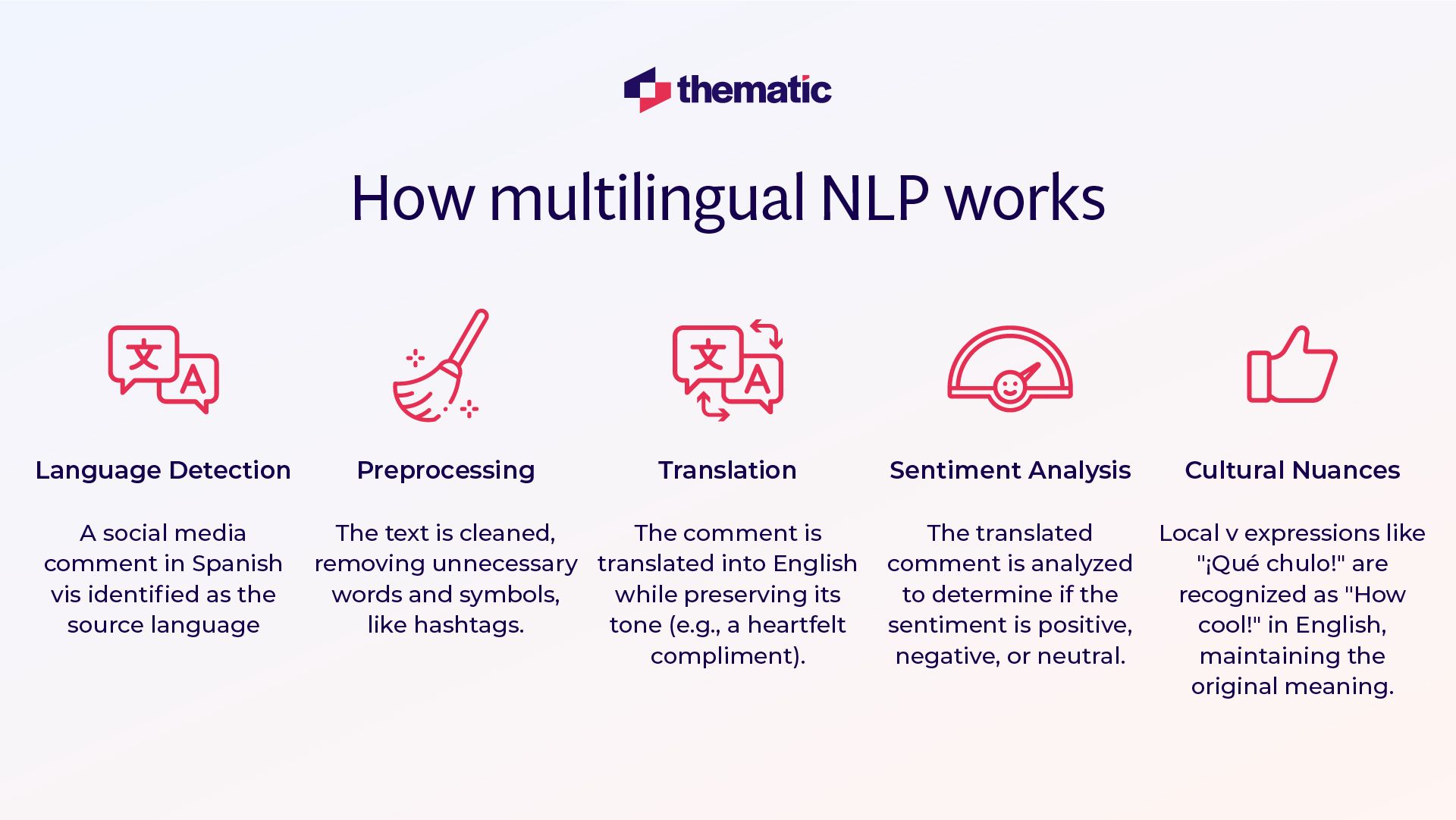
Resource Constraints: Cost and Skilled Talent
Implementing and maintaining robust systems that analyze text can be expensive, and sourcing skilled data scientists and NLP experts is often challenging. Smaller organizations, in particular, may struggle with the high costs associated with infrastructure and specialized talent acquisition.
Solution:
- Adopt cloud-based solutions that analyze text to reduce infrastructure and operational costs.
- Invest in upskilling existing teams through training in NLP and ML technologies.
- Partner with specialized vendors like Thematic to gain expertise without expanding headcount.
Melodics, a music-learning platform, partnered with Thematic to comprehensively analyze customer feedback without the need to expand their team. By leveraging Thematic's cloud-based platform, they streamlined their feedback analysis, ensured no customer comments were overlooked, and stayed within budget constraints.
Data Privacy and Regulation Compliance
Handling customer data responsibly involves navigating stringent data privacy laws and industry regulations. Failure to comply with frameworks such as the General Data Protection Regulation (GDPR) or the California Consumer Privacy Act (CCPA) can result in severe financial penalties and reputational harm.
Solution:
- Anonymize information extraction to safeguard personally identifiable customer data.
- Stay updated on evolving data protection regulations and integrate compliance measures.
- Use platforms that offer built-in compliance features for data storage and processing.
Watercare utilized Thematic's text analytics platform to manage and process customer feedback while adhering to stringent data privacy regulations. By implementing compliance-focused workflows, they ensured sensitive customer data was anonymized and processed securely. This not only helped them navigate legal requirements but also strengthened customer trust during a critical period.
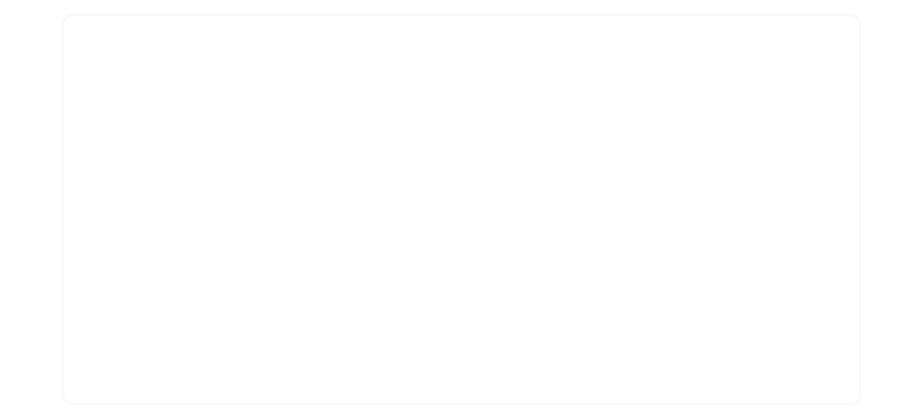
Thematic
AI-powered software to transform qualitative data into powerful insights that drive decision making.
Integration with Existing Business Intelligence Tools
Many organizations face difficulties integrating tools used in text analysis with existing business intelligence (BI) systems. Poor integration leads to fragmented insights, inefficiencies, and decision-making silos that limit the effectiveness of analytics efforts.
Solution:
- Select text analytics platforms with seamless API integration capabilities for popular BI tools.
- Standardize data formats to enable smooth data exchange between systems.
- Collaborate closely with IT teams to simplify and streamline integration workflows.
Think of BI systems and analytics tools as two puzzle pieces that need to fit perfectly for the bigger picture to emerge. Without proper integration, you’re left with scattered pieces that don’t make sense. Thematic ensures those pieces align, delivering a complete and actionable view of your customer data.
The good news: Thematic integrates with Power BI and many other solutions.
Real-Time Data Processing and Scalability Issues
In fast-paced markets, businesses must process customer feedback in real time to address emerging issues and trends. Scaling text analytics systems to manage real-time data streams poses significant challenges to text due to data volume, velocity, and variety.
Solution:
- Implement cloud-based platforms that support real-time data processing and analysis.
- Utilize event-driven architectures for efficient data ingestion and rapid analytics.
- Optimize algorithms for low-latency performance to deliver timely insights.
This is like trying to catch water from a waterfall with a small bucket. As more water flows, your bucket quickly overflows, and you can’t keep up. Real-time data processing is like upgrading from a small bucket to a powerful irrigation system that channels the water (data) efficiently, no matter the volume. Tools like Thematic act as that system, ensuring businesses can manage the influx of customer feedback without missing important details.
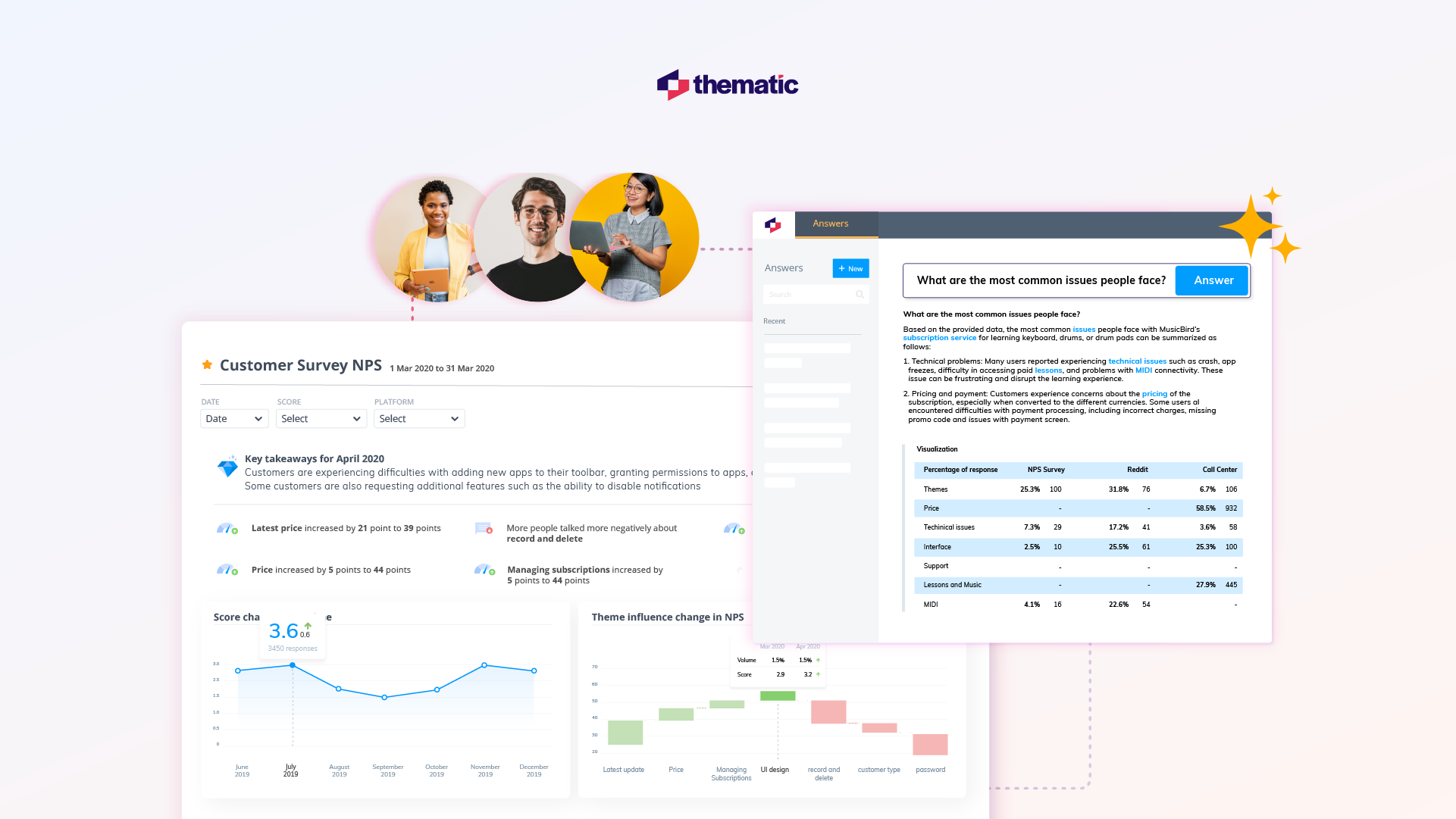
Sentiment Analysis and Topic Modeling Inaccuracy
Sentiment analysis and topic modeling are vital for understanding customer emotions and identifying key discussion themes. However, inaccuracies in these models can lead to misinterpretation of feedback and misguided business strategies.
Solution:
- Train sentiment analysis models using domain-specific datasets for greater accuracy.
- Leverage advanced topic modeling techniques to improve thematic detection.
- Continuously validate and update models to adapt to evolving language trends and customer behavior.
Managing real-time data as trying to navigate rush hour traffic with a small, single-lane bridge creates bottlenecks that delay progress. Thematic's tools act like an upgraded multi-lane highway, designed to handle large volumes of traffic smoothly and quickly, ensuring that no valuable insights are delayed or lost in the process.
Overcome Text Analytics Challenges
In any business, customer review analysis, which requires text analytics, is important in making sure every decision made matters. But as we’ve discussed here, text analytics has challenges—from managing unstructured data to information extraction to ensuring regulatory compliance—that can hinder organizations from fully leveraging customer feedback.
The best text analytics software can easily address these challenges. There are many solutions out there indeed, but why choose Thematic? Thematic does not only do the heavy lifting for you. It maps out the actions you ought to take to seize opportunities.
Try Thematic now on your own data to see how it can help you address text analytics challenges and get valuable insights for better decision-making.
Stay up to date with the latest
Join the newsletter to receive the latest updates in your inbox.