How to validate your AI-driven insights
The ultimate guide to using AI-driven insights for business growth
More and more analysts are finally embracing using artificial intelligence. With it, they are becoming more effective in uncovering insights for their organization.
AI-driven insights are a powerful tool to enable analysts to extract value at scale from (tens of) thousands of unstructured data points such as customer feedback.
Looking for key takeaways or frequently asked questions around validating AI-driven insights? Skip ahead to the end of this post!
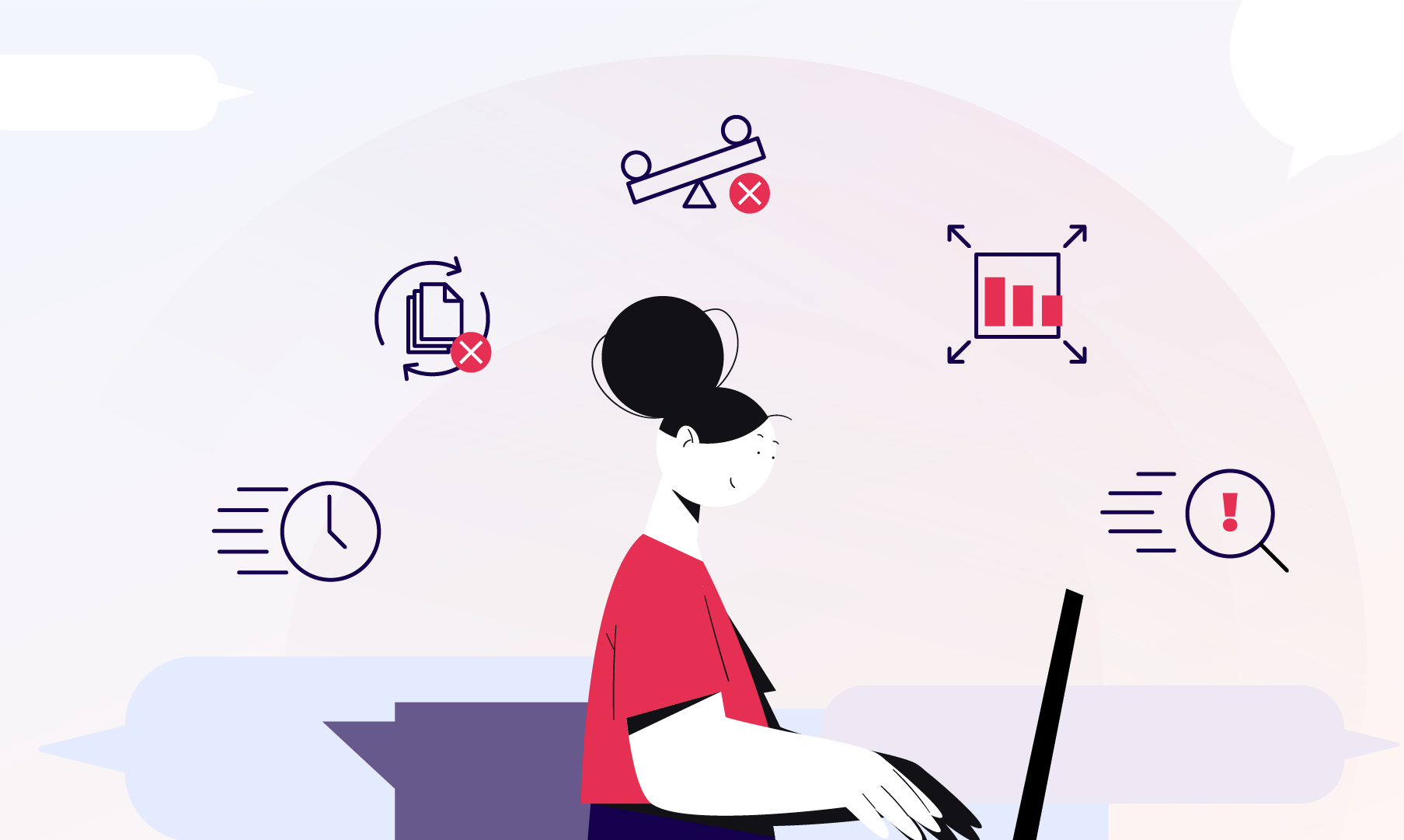
Analysts leverage AI to:
- Save time: Manual analysis is hugely time consuming, the reason why many companies leave their valuable qualitative data untouched.
- Reduce repetitive work: AI can take on some of the most hated tasks in data analysis, such as data cleaning.
- Reduce bias: Algorithms unbiasedly - and more consistently - interpret feedback data.
- Scale analysis: AI can handle large quantities of feedback effortlessly.
- Get insights in real-time: Feedback analytics software can monitor and communicate issues in real time.
While AI does give you an edge, empowering you to do so much more, and work a lot smarter, it does bring its own challenges. For example, how do you know if you can trust what the data is telling you? How do you know the AI isn’t hallucinating (the technical term for when AI makes things up)?
Thematic lives and breathes AI-driven insight. They've done so for years. So I've worked with them to compile best practice advice on how to thrive with using AI, condensed in one handy guide.
This guide will enable you to make the most of AI-driven insights to propel your organisation forward and turn customer feedback into a powerful growth engine.
Read on to discover:
- How you can validate and become confident your results are reliable
- The path to improving the quality and reliability of your insights
- How you can dig deeper and unlock transformational insights
- The path to convincingly sell the insights to stakeholders
Validating your AI-driven insights
The first person that you need to convince that your AI-driven insights are valid is you. So, how do you do that? The way in which you approach this is dependent on the AI tool you are using to reach your insights.
Most AI tools guard their processes very jealously and are, to all intents and purposes, a black box. You put data in, you get something back, but you don’t know how it got there.
This is one of the major ways Thematic goes against the grain. Thematic's platform builds trust through transparency. It’s easy to see how results have been obtained, and you can always map results back to your real customer verbatims. Not only that, Thematic offers you the opportunity to finetune the model so it learns to think like you, and is more accurate and specific to your business case.
But what if you aren’t with Thematic, but with a solution that is less forthcoming with its workings? It’s going to be more work for you to validate that the results are robust, but not impossible.
You will need to:
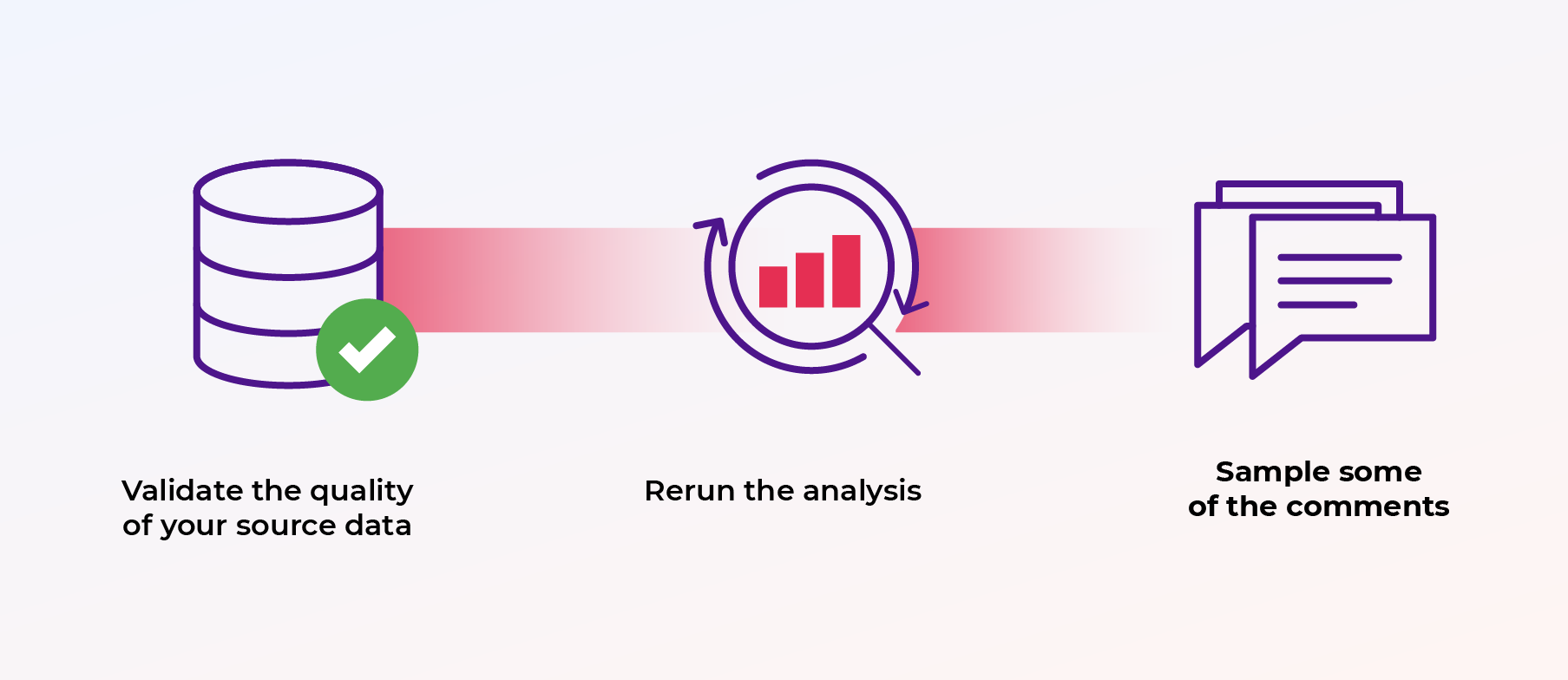
1. Validate the quality of the source data
The adage “garbage in, garbage out” has always rung true, but the impact of ignoring it is increasing rapidly with the explosion of AI (which feeds on data).
This means that your very first step (even if you use Thematic) is to look at the source material.
- Do the customer comments actually make sense?
- Is the data valid - are the comments answering the question you wanted them to answer?
- Is the data recorded correctly?
If your data is lacking in quality, don’t even put it through any form of analysis. Go back and fix this issue with more throughout data cleaning. How much data cleaning you need to do will depend on the tools you use.
For example, Thematic automatically filters out unnecessary noise such as “Hi! how are you?” that could muddy the analysis. Other tools may put a greater burden on the analyst to clean the data properly.
Crucially, make sure to be extremely hands on with data cleaning, as it does play a big part in building confidence in the results.
2. Rerun the analysis
Let’s assume your data is robust and reliable, and you’re comfortable with its quality. Now what?
Put the exact same dataset and parameters (or prompt) into the AI tool or software you use. Does it output something completely different?
While some minor differences are to be expected when using Generative AI (less so with other solutions), the result should be consistent across attempts. If it doesn’t, raise that internal alarm bell, and dig deeper to understand what is happening and what parameters need changing.
3. Sample some of the comments
Most tools give you examples of customer comments that align with the themes identified in the data. Sanity check if these actually make sense. Do you agree that these comments are properly categorised? Are the strengths genuine strengths?
Then, regardless of whether you managed to do the above, go a step further, and conduct your own analysis.
Select a random sample of comments to get a feel for whether it feels like the analysis is accurate. If you see a big discrepancy between the two, add some more comments to the pot to get further validation.
By the time this exercise is complete, you will have analyzed a fair few comments - how many depends on the size of your data set but this is one place you probably don’t want to cut corners. From your first-hand review of the data, does it feel like the AI output is aligned with the data?
If the insights that the AI has given you survive those two tests, you can be confident it is indeed representative of your data.
It may feel like this process defeats the purpose of using AI. However, after a few instances of your due diligence confirming the outputs, you will be able to dial the validation down to some extent. Even then, we’d recommend you always keep a certain degree of vigilance.
Having said that, the best way to make sure your findings are valid is to actually get your hands dirty and input into it.
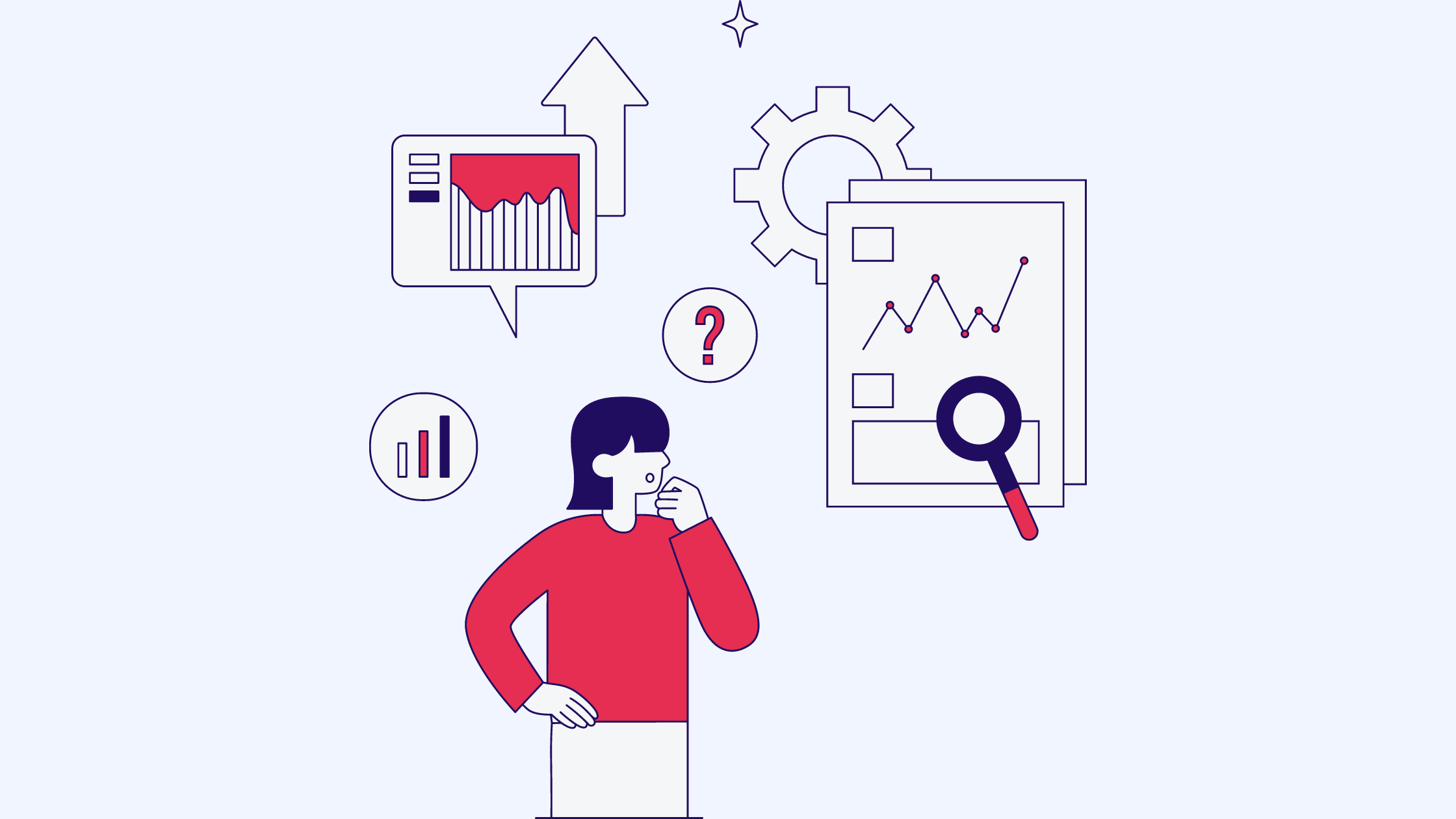
How to further strengthen the quality of your insights
Here, garbage in, garbage out, also applies. You validated that your data is of sufficient quality, but what about the AI you fed it to?
Text analytics solutions rely on a taxonomy.
A taxonomy is essentially a list of categories (or themes) that the data can be classified into.
For example, if I am a telecoms provider, my taxonomy would include themes such as “broadband speed”, “engineer visit”, or “contract renewal”. The AI looks at the comments one by one and tries to answer the question “to which category does this particular comment relate?”. Once identified, it adds that comment to the right theme(s) and keeps going until it is done categorizing.
This means that if you don’t have a taxonomy that fits your industry and circumstances, the results are unlikely to prove insightful.
Except in exceptional cases, you will be able to finetune and improve upon your taxonomy over time. It may be that your taxonomy was created based on your data, or you may have taken advantage of an existing one for your industry. Either way, dig deep into that taxonomy. Get to know it intimately. Give yourself the confidence that it is built appropriately to meet your needs.
Speaking of finetuning the taxonomy, it’s important to note here that not all tools are created equal: with some providers, you will be able to edit extensively and take complete ownership of the taxonomy (this is where Thematic sits). Others however are less permissive and will require you to put in a ticket to the provider for them to advise and make changes on your behalf. Make sure to account for the delays this would cause.
Whatever the outcome of your deep dive: set out to improve your taxonomy.
The better the taxonomy, the stronger the insights.
Crucially, the better the taxonomy, the more scalable your work becomes. Additionally, if you are involved in shaping your taxonomy, it will be stronger than something off-the-shelf, and you will know the parameters are fit for purpose.
What if you have been using a Large Language Model (LLM) such as GPT4?
In this case, there isn’t a taxonomy for you to assess, as the model is far broader than this. In this case, the quality of your outputs is largely dependent on the quality of the context you provide in your prompt (the input you give the LLM). Here, you need foundational skills in prompt design or engineering. Mastering the fundamentals of prompting will enable you to turn water into wine in terms of AI-driven insights.
It’s worth mentioning here that it doesn’t necessarily need to be an either/or situation with a traditional taxonomy versus LLMs. Thematic utilizes LLMs within the platform to deliver instant summaries and enhance the quality of the analysis provided. Thematic feeds in high-quality, themed, and curated data through to LLMs so it has a stronger context to work against rather than “all the data in the dataset”.
Whichever tool you use, the key message here is that the more hands-on you are in improving the inputs, the more reliable your results become. This will also give you the confidence to better articulate and defend the insights.
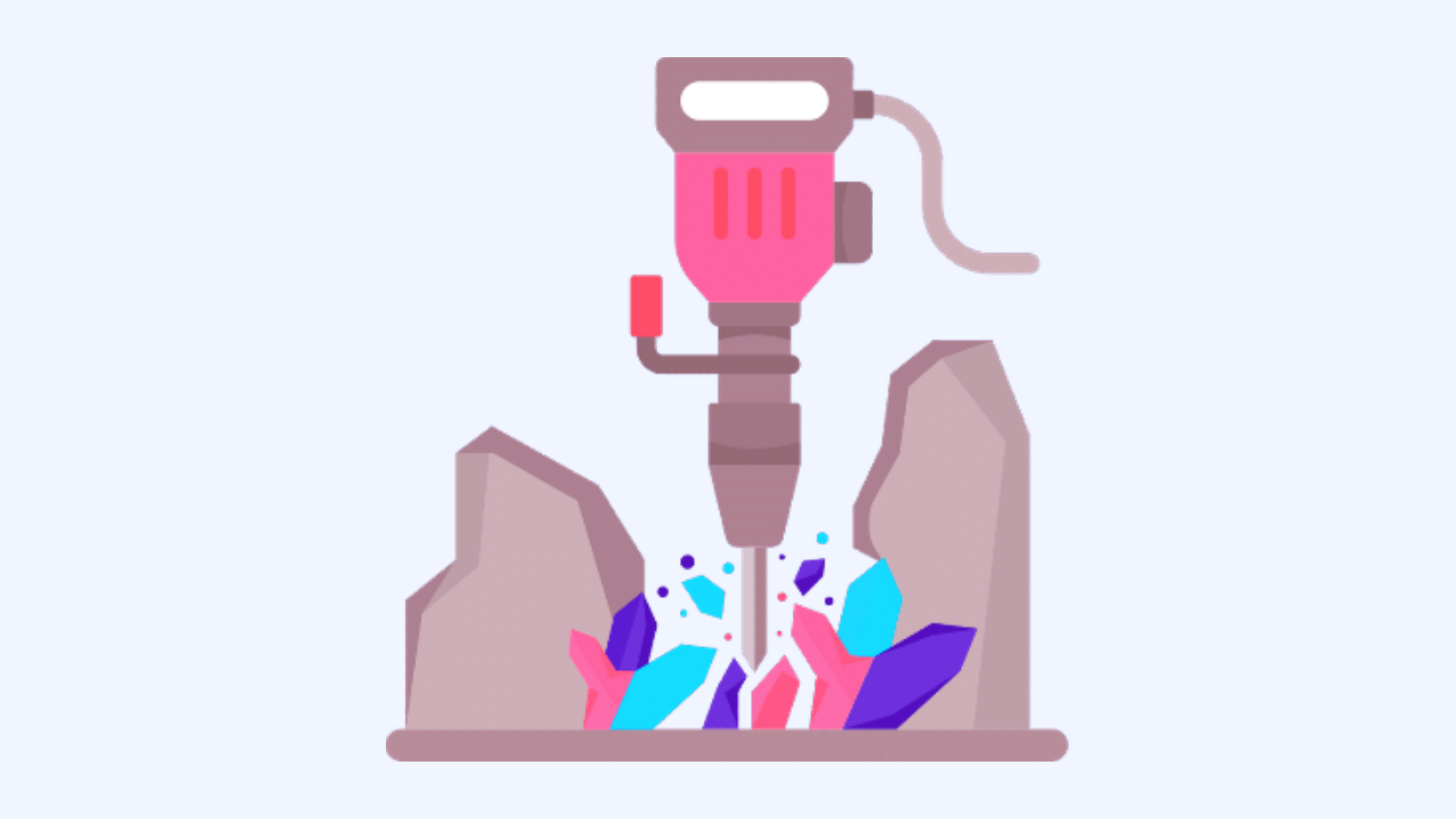
Dig deeper for transformational insights
Using AI should only be a step in the process, and never the finished product.
Structured data such as a score offers a bird’s eye view on how customers view their experience with an organisation. Unstructured data (like customer comments) provides the next layer of richness, as it gives us a stronger understanding of “why?” that drives and colours the perception the customer has of an organization.
When unstructured data is coupled with a powerful AI solution, analysts are able to get very granular at scale and understand precisely what the strengths and opportunities are for an organization to grow.
Using an AI-enabled platform, analysts can surface particular themes and promising insights, answering many business questions.
But the analysis will also spark many more questions the data just cannot answer in its current state. This is where conducting follow-up conversations around what the AI surfaced becomes incredibly valuable. Indeed, you will find that by following up, a deeper level of insight can be discovered.
This step beyond the AI is fundamental for three key reasons:
- It validates whether there is indeed an opportunity and how meaningful it is - is this something customers actually care about?
- You gain a better understanding of the opportunity, so you can start to shape the solution you will design
- Conversations reveal tangible anecdotes, genuine stories that you can play back to stakeholders
This is where the magic happens, this is where the Eureka! moments appear. And this is how you will be able to drive meaningful change.
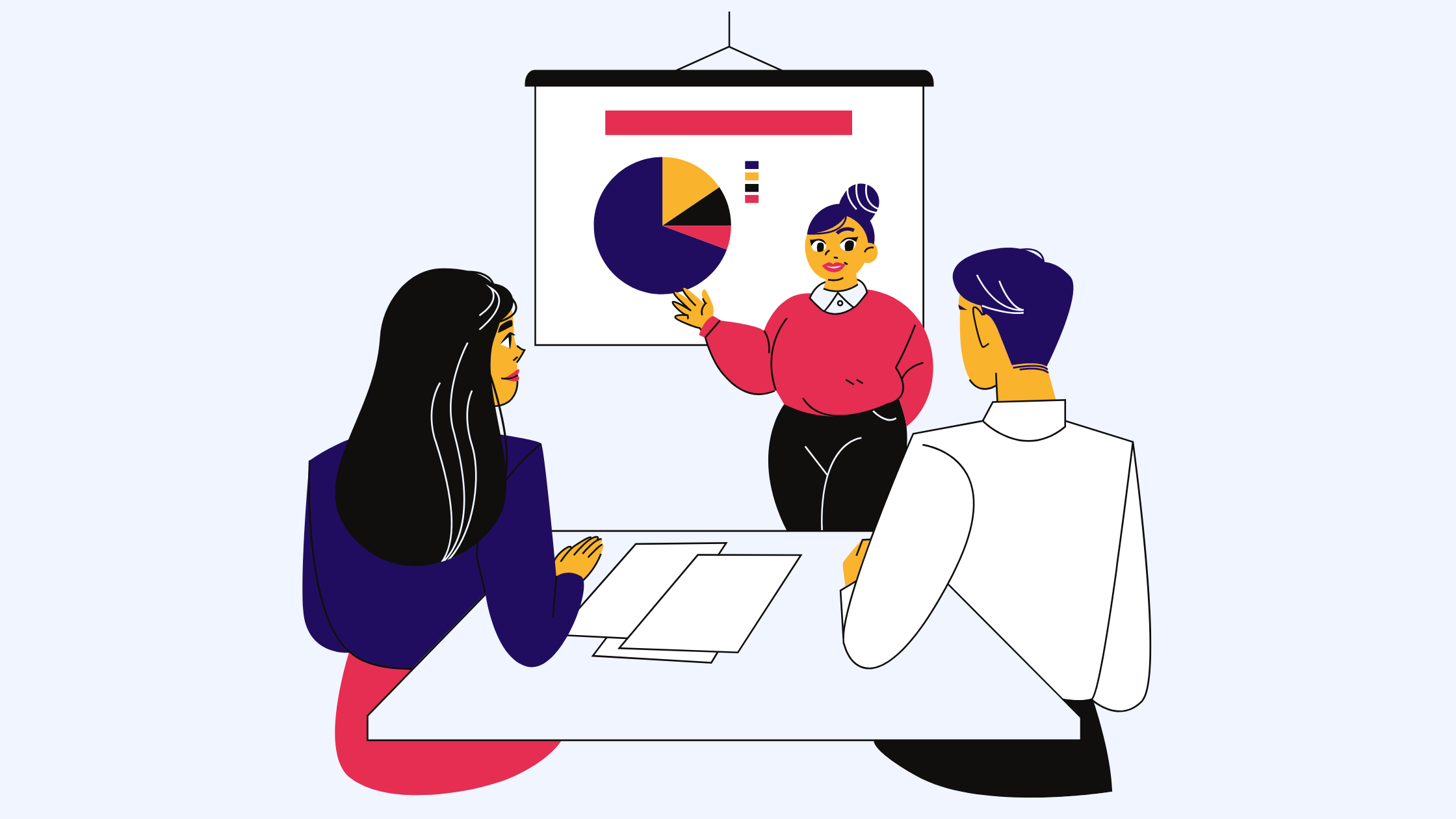
Getting buy-in for change from stakeholders with storytelling
You are now comfortable with the robustness of your AI-driven insights. You have also dug deeper and spoken to your customers about that insight.
This has enabled you to shape a solution that is very likely to hit the mark and improve your customers’ experiences.
Next up, you need your stakeholders to buy into your idea so you get the necessary resources to implement it.
It is undeniable that pitching to stakeholders and getting them on board is an art, and one that shouldn’t be underestimated. How do you get people excited about your idea? How do you get them to see the value the change you are proposing would bring to the organisation?
To truly succeed you need two assets: your data-driven insights, and your human stories you collected from your comments and follow-up conversations. The reality is that AI-driven insights and narratives go hand in hand, each representing one side of the same coin.
Use the story of a particular customer to frame the recommendation you are making and use their own words via transcripts, comments on surveys or even recordings to ensure you relay the situation in the customers’ own words.
Don’t be shy about telling the story. The more tangible and relatable you make the struggle, and the more your stakeholders can relate to it, the more likely you are to gain their support at an emotional level.
And to tackle their rational side, provide strength to your narrative by using your AI-driven insights to introduce the wider context: what’s at stake, the size of the opportunity (or pain) and why your stakeholders should care. While presenting your case, layer in specific comments of how your customers talk around the issue you are attempting to address, in their own words to provide a richer picture.
By combining the analytics, the anecdote, and several examples of other customers talking around the proposal you are puting forward, you will be able to present a strong case to get buy-in and drive change for customers.
As you approach your stakeholders, be aware people are still reluctant around AI. This means that when you do speak to them, you want to ensure you convey the role played by AI.
Articulate that the insight isn’t driven by AI but instead enabled, or facilitated, by it.
- You started the process by identifying what question you wanted to answer.
- You then took the output from the AI, challenged it, and then confirmed it with customers who provided you with tangible examples and further depth on the problem you are trying to solve.
The process doesn’t start, nor does it end, with AI, and it’s important that your stakeholders are aware of this when you pitch to them.
Ultimately, remember what it is all about
AI-driven insight, whether through well-established taxonomy-based solutions, or the newer advent of Generative AI , or a tool that utilizes both, can be incredibly powerful in enabling you to dedicate your time to higher value activities. You can dig far deeper than you could otherwise, especially if you operate at a large scale.
Insights are never the goal, but rather a means to an end. Data for the sake of data is of limited value. It only becomes powerful once it is used to drive improvements.
And that’s exactly what we all must bear in mind when we analyze customer feedback: unless it drives genuine, measurable change, it is all done in vain. The value of your insight, whether aided by AI or not, is dependent on what you do with it and how it impacts business growth.
Now you’ve completed this guide, you have a clear step-by-step you can follow to transform customer feedback into genuine business growth with AI. And don’t forget: AI is an enabler, only you, as the expert, can drive the change that will take your business forward.
Key takeaways
- Analysts leverage AI to help make sense of large volumes of feedback. AI help to save time, reduce bias and repetitive work, scale analysis, and monitor real-time issues.
- There can be a struggle with trusting the results from AI. It's hard to trust results when you can't see the process. This is where validation comes in.
- Crucial steps to validate AI-driven insights are checking the quality of your data, re-running the analysis, and sanity checking a sample of comments against the AI's analysis.
- Your insights are only as good as the quality of data and the categorization list, known as a taxonomy, you're feeding the AI. So, get to know your taxonomy like the back of your hand and don’t be shy about making improvements.
- The use of AI should only be one step of your process, not the end goal. Pair it with follow-up conversations or additional research based on what the AI revealed to get richer insights.
- You've got great insights and now you want your team to buy into them. Mix data-driven insights with real-life customer stories to create a convincing narrative. Make it tangible and relatable to win your stakeholders on an emotional and rational level.
- Finally, while AI is a game-changer, remember it's just a helper in the process. The value of your insight depends on how it's used to drive improvements and business growth. AI is an enabler, but only you can steer your business in the direction of growth.
Frequently asked questions
1. What are AI-driven insights and how can they help businesses?
- AI-driven insights are results obtained from using artificial intelligence to analyze data. AI massively speeds up the time to insights, which in turn makes it faster and easier for companies to improve products and services.
2. What challenges can arise when using AI to analyze large volumes of data?
- It's hard to trust AI-driven insights when you can't see inside the analysis process. Not all AI solutions are equal, which is why it's a good idea to build trust by validating your results.
3. How can I validate AI derived results?
- Validation can be done by checking the quality of your data, rerunning the analysis, and analyzing a sample of comments compared to the AI's results.
4. What is a 'taxonomy' and why does it matter?
- A taxonomy is a list of categories used to classify data. Having a comprehensive taxonomy ensures your AI tool is more accurate in analyzing data, which in turn makes for more accurate insights.
5. How can I improve the quality of AI-driven insights?
- Improving your taxonomy is one way to boost the quality of insights. The better the taxonomy, the stronger the insights and the more scalable your work becomes.
6. Will AI replace me as an analyst?
- No. AI is a tool to help you work faster and more efficiently. With feedback analysis, human intervention is crucial for extra validation, conducting follow-up research, and digging deeper into insights.
7. How can I get stakeholder buy-in for data-driven recommendations?
- Combining robust AI-driven insights with compelling human stories helps sell ideas to stakeholders. Make issues tangible and relatable for emotional buy-in, and back up your statements with quantifiable data points, like 'x number of customers are affected'.
8. Why should I bother with additional research or customer conversations when using AI-driven insights?
- Following up allows for validation and deeper understanding of the AI-produced insights. This additional layer helps uncover more details and can lead to more profound, transformational insights.
---
About the author: Gustavo Imhof is a leading customer experience expert. Not only is he a co-author of the international best-seller Customer Experience 3, but he also was named one of 30 rising stars under the age of 30 in customer experience worldwide.
Gustavo splits his time between professional academy Avado, where he is a product director, and his own consultancy CXAhead, where he offers customer experience advisory, coaching and content creation services.