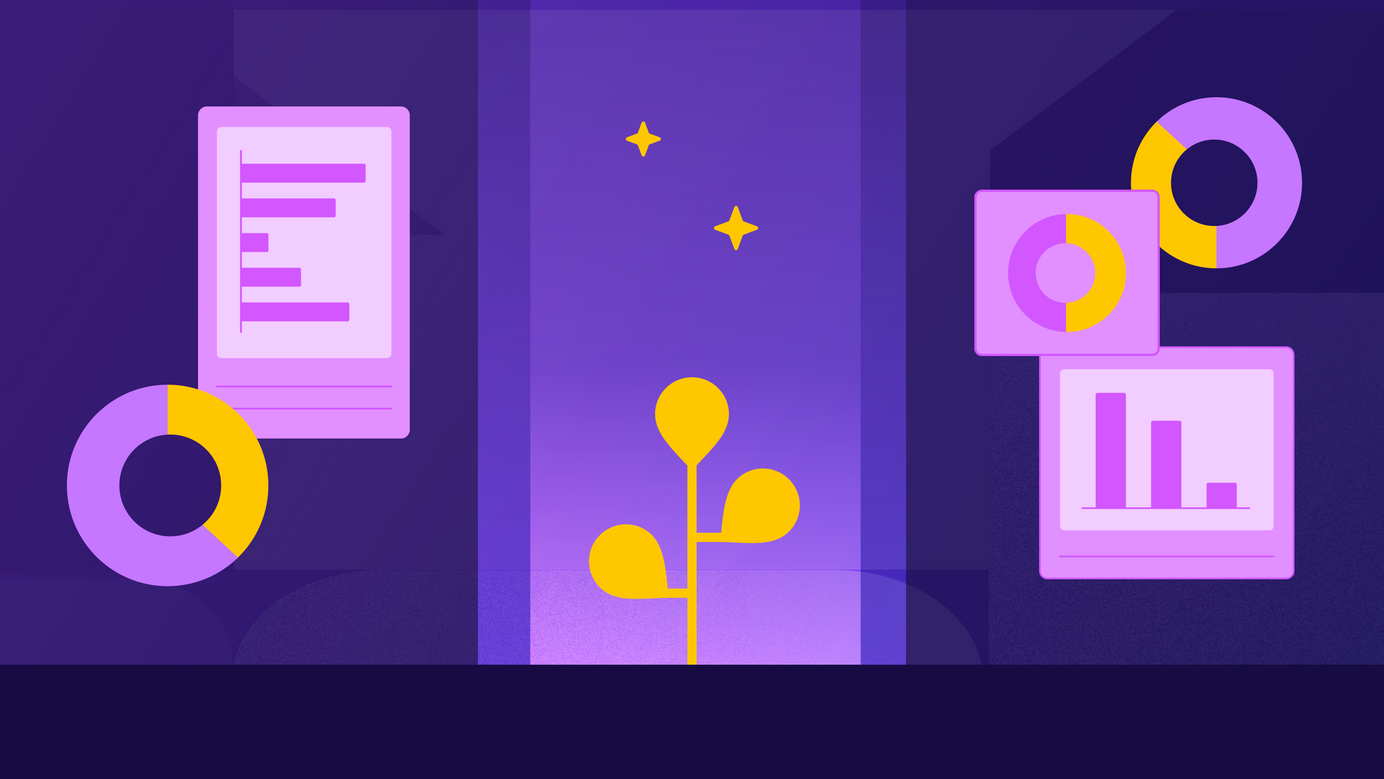
The Explosive Growth of the Text Analytics Market: Trends, Opportunities, and Forecast
Explore the booming text analytics market—key growth trends, drivers, industry insights, and forecasts reaching $78B+ by 2032.
By 2027, 87% of CX leaders plan to use AI-driven text analytics to power their customer interactions. Why? Because customers are tired of slow responses, generic replies, and unresolved issues.
Text analytics—especially when powered by AI—is changing that. It helps businesses make sense of messy, unstructured feedback and turn it into something that actually improves service, products, and customer relationships.
And the momentum behind it is real. The text analytics market is expected to skyrocket from around $29 billion to over $78 billion in the next few years.
In this article, we’ll explore
- what’s fueling that growth,
- where the biggest opportunities lie, and
- why now is the time to start paying attention.
Let’s start.
Understanding the Text Analytics Market
At its core, text analytics is all about turning unstructured text—like customer feedback, emails, support tickets, and social media posts—into something useful. It uses technologies like natural language processing (NLP), sentiment analysis, content categorization, and entity extraction, among others, to make sense of language at scale. That means identifying patterns, emotions, topics, and even specific mentions of products or features hidden within text data.
What makes this so powerful is that around 80% of business data today is unstructured. Traditional tools can’t handle it, but text analytics can. For example, a company might use it to quickly spot that customers are frustrated with shipping delays or that a recent product update triggered a spike in negative sentiment.
As adoption grows, the text analytics market is evolving rapidly. According to Mordor Intelligence, the global market is projected to reach $78.65 billion by 2030. This growth is fueled by businesses wanting to extract deeper insights from massive volumes of text and react faster to customer needs.
Similarly, other market reports note steady double-digit growth, showing that this is more than a passing trend—it’s becoming essential.
In short, the top benefit of text analytics is helping businesses stop guessing and start knowing what their customers really think.
Market Growth and Key Statistics
If the numbers are any indication, the text analytics market isn’t just growing—it’s exploding. As mentioned previously, the global market is set to hit a staggering $78.65 billion by 2030, growing at a CAGR of 39.9%. That’s not a small bump—it’s a massive leap driven by AI innovation, rising data volumes, and the push for real-time decision-making.
Other industry reports echo this upward trend, though the specific figures vary:
- Allied Market Research forecasts the market will reach $29.42 billion by 2030, growing at 17.8% CAGR.
- SNS Insider via GlobeNewswire projects $41.2 billion by 2032, with 17.65% CAGR.
Why the variation? It comes down to how each firm defines the market. Some include adjacent tech like machine learning and speech analytics, while others focus solely on pure-play text analytics platforms. There are also differences in how sectors, regions, and deployment models (like cloud vs. on-premise) are factored in.
No matter which figure you lean on, the consensus is clear: text analytics is becoming a must-have.
Whether you’re trying to improve customer service, understand social media sentiment, or enhance product experiences, now’s the time to dive in.
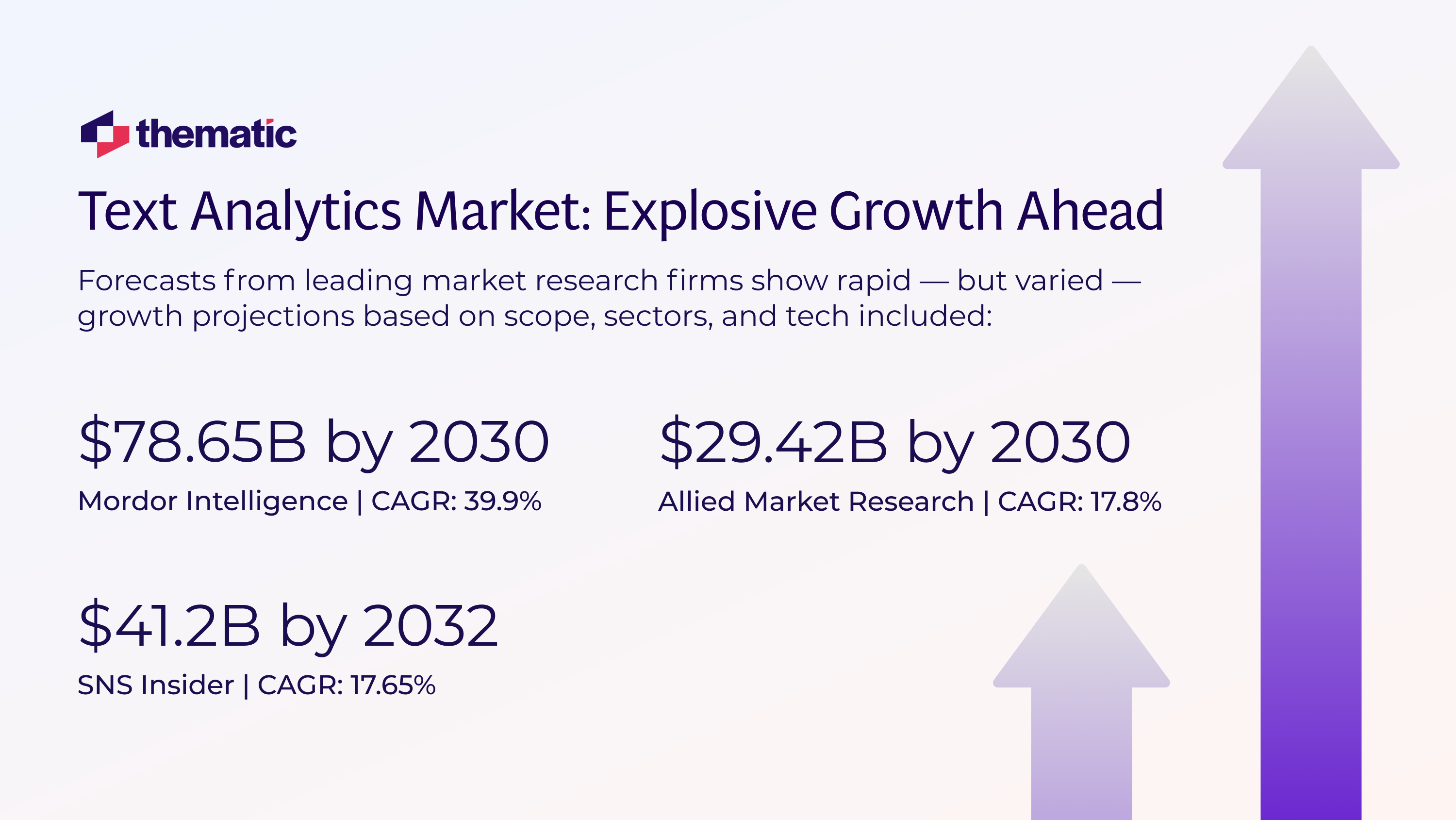
4 Major Drivers Fueling the Text Analytics Market
So, what’s behind the rapid rise of the text analytics market? In short, a perfect storm of data overload, smarter tech, and rising expectations.
The drivers we cover in this section are central to the market’s projected multi-billion dollar growth over the next decade.
- Exponential growth in unstructured data (social media, emails, customer feedback).
- Increased demand for advanced AI and NLP technologies.
- Widespread adoption of cloud-based solutions.
- Rise of predictive analytics and customized industry-specific applications.
1. Unstructured data is growing—fast.
We’ve mentioned this earlier: Over 80% of enterprise data now is unstructured. Businesses are sitting on a goldmine of insights hidden in open-ended survey responses, emails, support chats, and social media posts.
The challenge? This kind of text data is messy and inconsistent, and qualitative data analysis has become taxing to do at scale.
Just look at the case of LendingTree. Their team was flooded with over 20,000 customer comments in just three months—a volume too big to handle through traditional means. What did they do? They used Thematic, which automatically analyzed feedback and pinpointed a specific issue: customers were getting calls at odd hours due to time zone mismatches. This wasn’t just an annoyance—it was costing them leads and potential revenue. With Thematic’s insights, they sized the impact and took immediate action, improving both customer experience and business performance.
Such a challenge with massive amounts of unstructured data is not unique to LendingTree. Many businesses are experiencing this, and they want to make sense of the data to get insights that can help them stay ahead of their game. As such, it makes sense why the text analytics market is growing at a rapid pace.
2. AI and NLP are getting better—and more accessible.
What’s really fueling the next wave of growth in the text analytics market is the evolution of AI itself—particularly in NLP. For years, traditional NLP helped businesses classify text and identify sentiment using rule-based systems. But these models depend on pattern recognition over true understanding, which often leads to errors when interpreting sarcasm, layered emotions, or nuanced context.
Now, Large Language Models (LLMs) are shifting that paradigm. Unlike earlier systems, LLMs don’t just match patterns—they understand context, nuance, and intent. And with the rise of generative AI applications built on LLMs, businesses can now go beyond keyword tagging into a world where machines can summarize, explain, and even predict emerging themes in their data.
AI has dramatically reduced the need for manual work like coding qualitative data, which has traditionally been one of the most time-consuming tasks in customer research.
Instead of manually labeling thousands of survey responses, teams can now use AI-powered text analytics to automatically identify new trends, understand the emotion behind them, and surface insights in real time.
Take Atlassian, for example. The company was overwhelmed by huge volumes of feedback coming from multiple channels across products like Jira and Trello. Their teams were spending weeks manually categorizing feedback in whiteboards, only to produce quarterly reports that were too slow to act on.
By adopting Thematic, an advanced feedback analytics platform powered by LLM-based Text Analytics, they automated the theming process, significantly reduced time spent on analysis, and built a real-time feedback loop that helped them identify issues, prioritize product fixes, and respond to customer needs faster than ever.
“Thematic is your own personal analyst, only your analyst works 1000 times faster than you,” said Mick Stapleton, Atlassian’s CX leader.
This shift—powered by AI— is unlocking insights that would otherwise go unnoticed and making them available to every team that needs them, in real time.
Beyond NLP: How LLMs Transform Text Analytics
Is your Text Analytics solution still relying on B-Grade NLP? Discover how large language models are revolutionizing text analytics, offering deeper insights than traditional NLP approaches.
- Understand key NLP limitations and LLM advantages
- View real-world results of AI-driven text analytics
- Learn how self-learning AI eliminates manual updates
- Cut analysis time from weeks to minutes
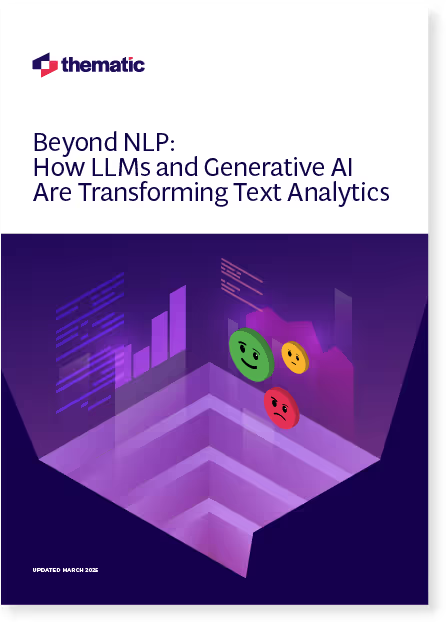
3. Cloud-based solutions are everywhere.
One of the biggest reasons the text analytics market is growing so rapidly? The cloud.
Cloud computing has made advanced analytics tools far more accessible to businesses of all sizes. Instead of building expensive infrastructure or hiring dedicated data teams, organizations can now deploy text analytics platforms on demand, scale them easily, and integrate them seamlessly into their workflows.
This shift isn’t slowing down. As of 2024, over 98% of organizations worldwide use cloud services in some capacity, and public cloud service revenue is expected to surpass $526 billion. Software-as-a-Service (SaaS)—the model many text analytics tools rely on—makes up a major portion of this spend, offering companies flexibility, automatic updates, and fast deployment.
The benefits go beyond cost and convenience. Cloud platforms support real-time collaboration and cross-functional access, which means marketing, product, and CX teams can all tap into customer insights without waiting for weekly reports. And with support for hybrid and multi-cloud environments—now used by 89% of businesses—companies can avoid vendor lock-in and choose the best-fit solutions.
This is especially game-changing in text analytics, where new data is constantly flowing in from multiple sources. Cloud-native platforms can handle these dynamic workloads, delivering near-instant insights from qualitative data—something that simply isn’t possible with traditional on-premise setups.
As businesses continue to digitize, the cloud isn’t just enabling text analytics—it’s supercharging it.
4. There's a growing demand for predictive and industry-specific insights.
Text analytics is no longer just about looking backward. More and more businesses are using it to predict what’s next—from forecasting customer churn to proactively improving products and services. That shift is one of the key forces driving the text analytics market forward.
According to a recent study, predictive analytics plays a vital role in helping companies stay competitive:
“Without leveraging predictive analytics, businesses risk missing out on opportunities, failing to anticipate market trends, and being unprepared for potential disruptions.”
Industries like retail, healthcare, finance, and SaaS are leading the charge. For example, retailers now use predictive models to identify product trends by region and season, optimize supply chains, and tailor promotions to individual customer behaviors. In healthcare, predictive analytics is being applied to monitor patient risk, predict medication needs, and guide care pathways.
This move toward forward-looking insights, powered by machine learning and AI, is what separates modern text analytics from the rule-based systems of the past. As applications become more tailored to specific industries—and more intuitive for cross-functional teams—the market is expected to continue its rapid climb over the next decade.
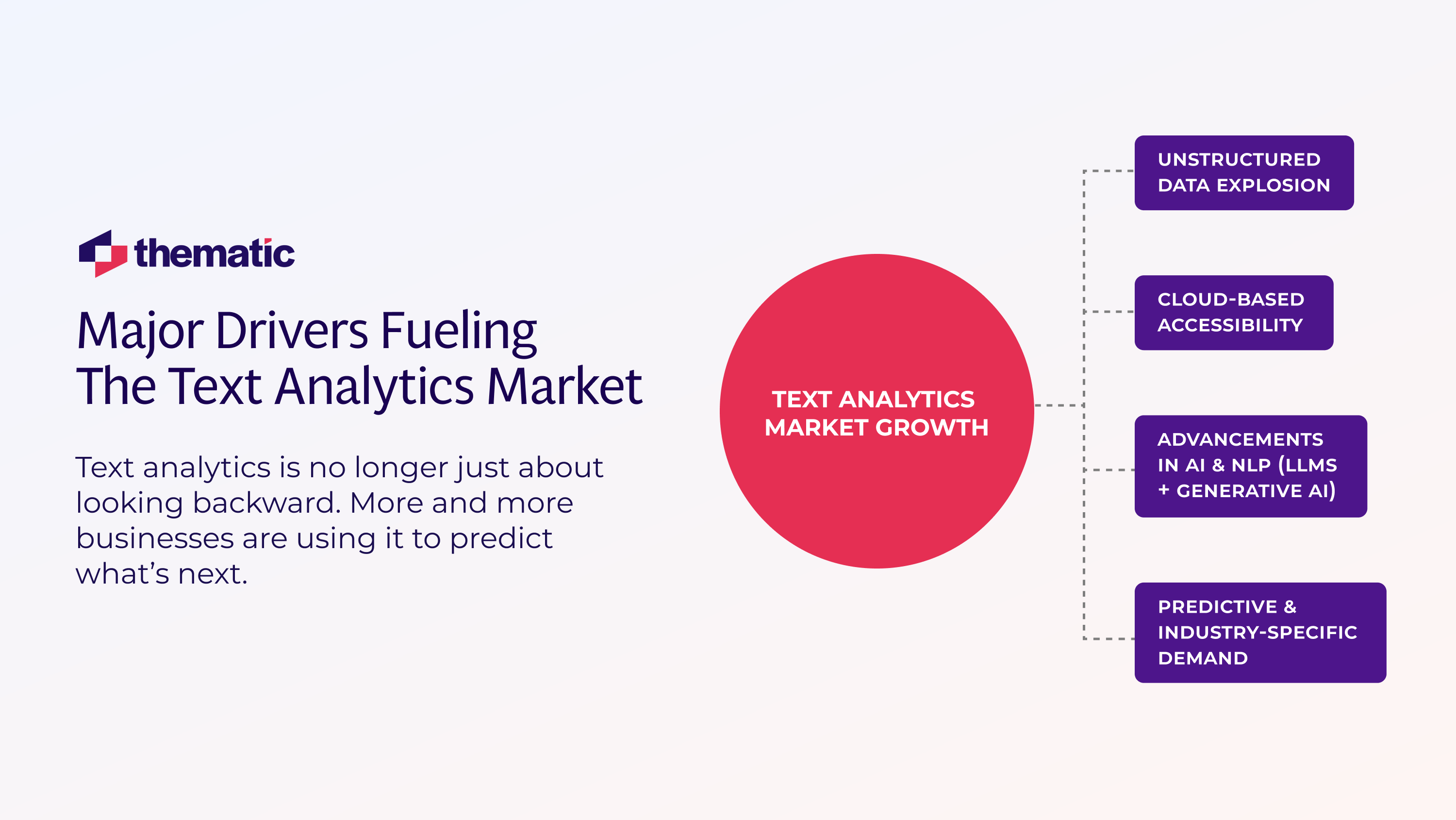
Industry-Specific Applications
One of the key reasons the text analytics market continues to grow at such a rapid pace is its versatility across industries. From healthcare to finance, organizations are turning to text analytics to unlock insights from their most valuable—yet most chaotic—data sources: customer conversations, open-ended survey responses, support tickets, and more.
Healthcare
In the healthcare space, text analytics is being used to improve patient outcomes by analyzing qualitative feedback, electronic health records, and support logs. Hospitals and insurance providers are applying AI-driven tools to understand pain points in patient journeys, manage medical data more efficiently, and even predict readmission risks.
One case in point is the deployment of a text analytics platform at University College London Hospitals. It enabled the processing of over 18 million records, enhancing clinical research and patient management.
Moreover, text analytics aids in fraud detection within healthcare systems. By analyzing textual data, organizations can identify inappropriate prescriptions, questionable referrals, and fraudulent insurance claims, thereby safeguarding against deceitful practices.
Retail & E-commerce
Retailers are using customer sentiment analysis to tailor product offerings and deliver personalized marketing at scale. Text analytics helps identify which product features matter most to customers and why certain promotions succeed—or flop. With real-time feedback analysis, e-commerce brands can adjust pricing strategies, optimize recommendations, and boost customer loyalty.
Old Navy, for example, implemented a new system across 1,200 stores to use RFID, AI, and computer vision to track inventory in real-time. With it, employees were able to quickly locate products, restock shelves, and fulfill online orders, thereby enhancing the overall shopping experience.
Furthermore, sentiment analysis enables retailers to gauge customer opinions from reviews and social media, informing product development and marketing strategies. By analyzing feedback from various sources, businesses can gather valuable information to enhance product offerings and tailor marketing strategies.
Finance & Banking
Banks and fintech companies use text analytics to monitor compliance, detect fraud, improve customer experience, and drive strategic decisions.
One real-world example is Atom Bank, which struggled to unify these insights across teams and touchpoints. They turned to Thematic, which, as mentioned earlier, is a feedback analytics solution using LLM to analyze unstructured customer data at scale.
With Thematic, Atom Bank was able to standardize analysis, eliminate data silos, and uncover which themes were driving—or hurting—their customer experience metrics. The insights led to clear, measurable impact:
- A 40% reduction in calls related to device issues
- A 69% drop in calls about unaccepted mortgage requests
- A 110% growth in customer base
Text analytics didn’t just help Atom Bank interpret feedback—it helped them prioritize product improvements, align cross-functional teams, and support the C-suite with strategic insights. It’s a prime example of how modern financial institutions are leveraging AI to transform unstructured data into clear, growth-driving action.
Social Media & Brand Monitoring
In the digital age, public sentiment changes by the minute—and brands that fail to track it risk falling behind. Brands use text analytics for social media to monitor, interpret, and act on consumer opinions in real time, especially across social media platforms.
One of the most common applications of text analytics in this space is sentiment analysis, which uses NLP to detect the emotional tone behind social media posts, reviews, and online conversations.
A standout example is Starbucks. This famous company applies sentiment analysis in near real time to evaluate how products are being received and quickly address potential issues. For example, if negative sentiment spikes, they can immediately tweak elements like pricing or even the coffee blend itself. This fast feedback loop allows them to stay on top of consumer expectations and protect their brand experience.
This is the power of social media sentiment analysis. With modern text analytics tools—capable of analyzing thousands of tweets per second—companies can transform noisy data streams into strategic brand insights. It’s a powerful example of how brands can “listen” at scale and respond with speed.
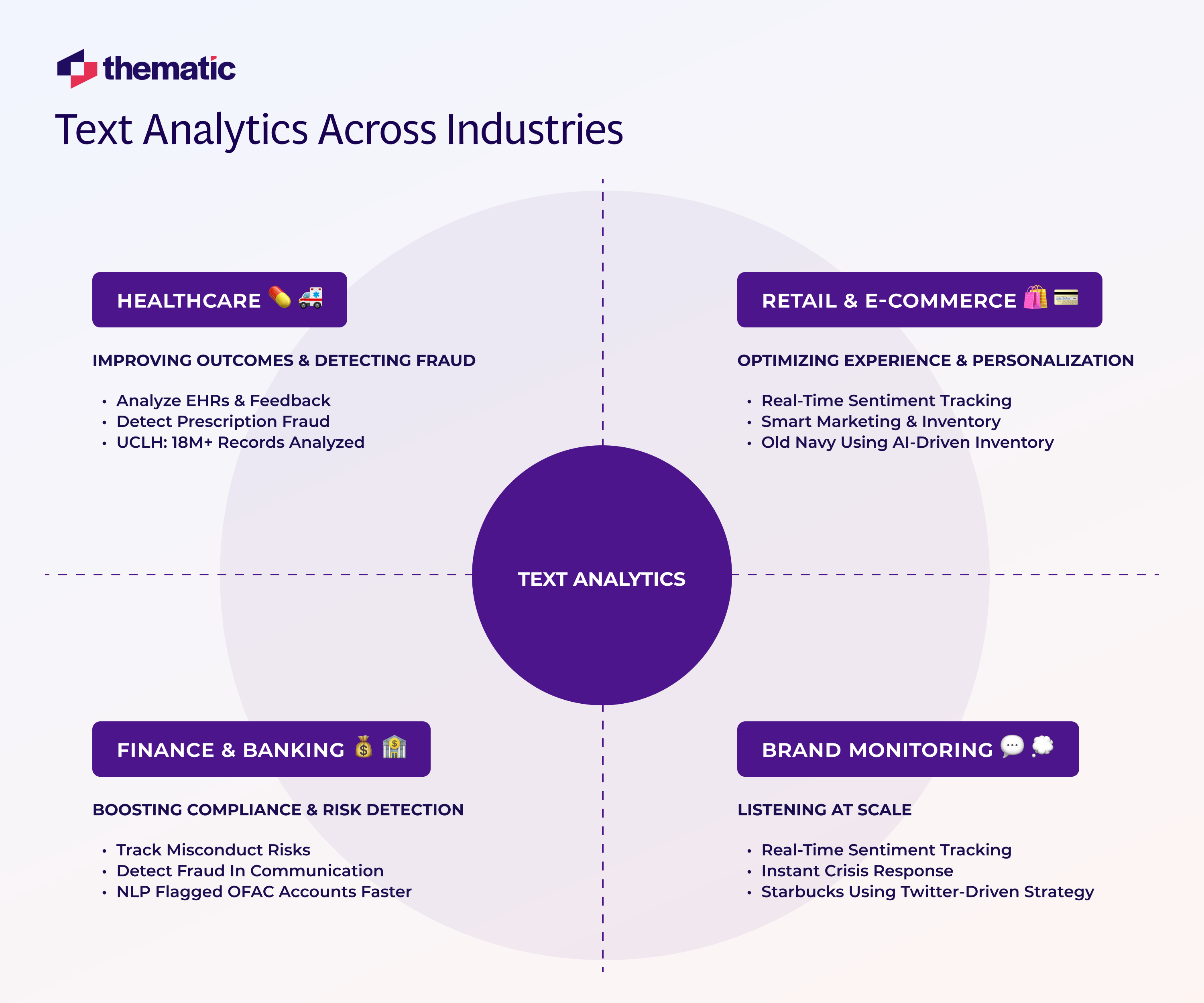
Regional Insights: Where Is the Market Growing Fastest?
The growth of the text analytics market isn’t evenly distributed—it’s driven by specific regions with distinct advantages in technology, infrastructure, and digital adoption.
North America
North America, particularly the United States, still dominates the market. As early adopters of AI, NLP, and enterprise SaaS solutions, U.S.-based organizations benefit from advanced infrastructure and a high concentration of tech-savvy businesses. The region also boasts a strong presence of leading text analytics providers and a high demand for customer-centric platforms across industries.
Asia-Pacific (APAC)
The APAC region is experiencing rapid growth, driven by accelerating digital transformation across sectors. China and South Korea are seeing surging demand for text analytics solutions in banking, e-commerce, healthcare, and government. As companies in these regions embrace cloud technology and invest in smarter tools for consumer insight, adoption is expected to skyrocket. In fact, APAC is projected to be the fastest-growing region throughout the forecast period.
Emerging markets are also making notable strides. In India, a rising startup ecosystem and widespread mobile penetration are creating new opportunities for scalable, cloud-based analytics platforms.
As demand spreads globally, businesses looking to expand their reach must not only adopt the right tools—but also consider the regional dynamics shaping how text analytics is used.
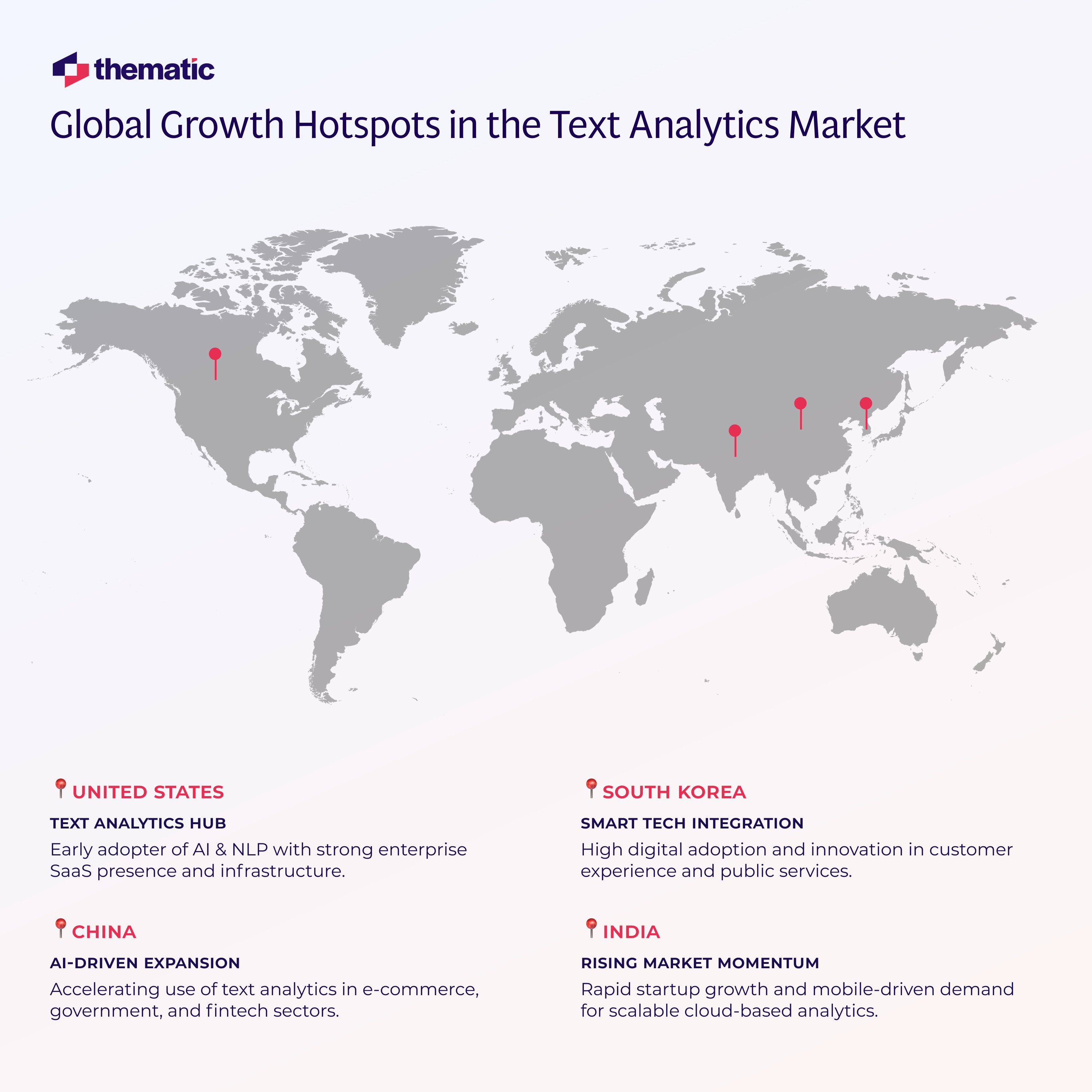
Key Challenges Facing the Text Analytics Market
Despite its rapid growth, the text analytics market still faces several hurdles that can slow adoption and impact outcomes.
One of the biggest challenges is data privacy and security. Text analytics often involves analyzing sensitive information—like customer complaints, healthcare records, or financial interactions—which raises serious concerns about how data is stored, processed, and anonymized. Companies must keep up and comply with evolving regulations like GDPR and CCPA. That also means they must choose tools that prioritize secure data handling.
Another roadblock is the complexity of multilingual data analysis. As businesses expand globally, they face the challenge of analyzing customer feedback in dozens of languages, each with its own nuances, slang, and cultural context. While LLMs and AI help close the gap, accurate interpretation across languages remains a key barrier—highlighted in text analytics challenges.
Finally, there's a growing skills gap. The field requires professionals who understand both data science and natural language processing, and there's still a shortage of experts who can implement, manage, and optimize these systems. Companies looking to scale their analytics need not just tools but teams trained to use them effectively. To address this, many turn to no-code or low-code platforms and follow text analytics best practices to get up and running quickly.
These challenges won’t stop the market’s momentum—but they are important to acknowledge as businesses seek to build more intelligent, human-centric analytics strategies.
That’s why security, privacy, and compliance should be top of mind when selecting a provider. Thematic is SOC 2 Type II certified, meaning we’ve been independently audited and proven to maintain strong, consistent controls for protecting customer data.
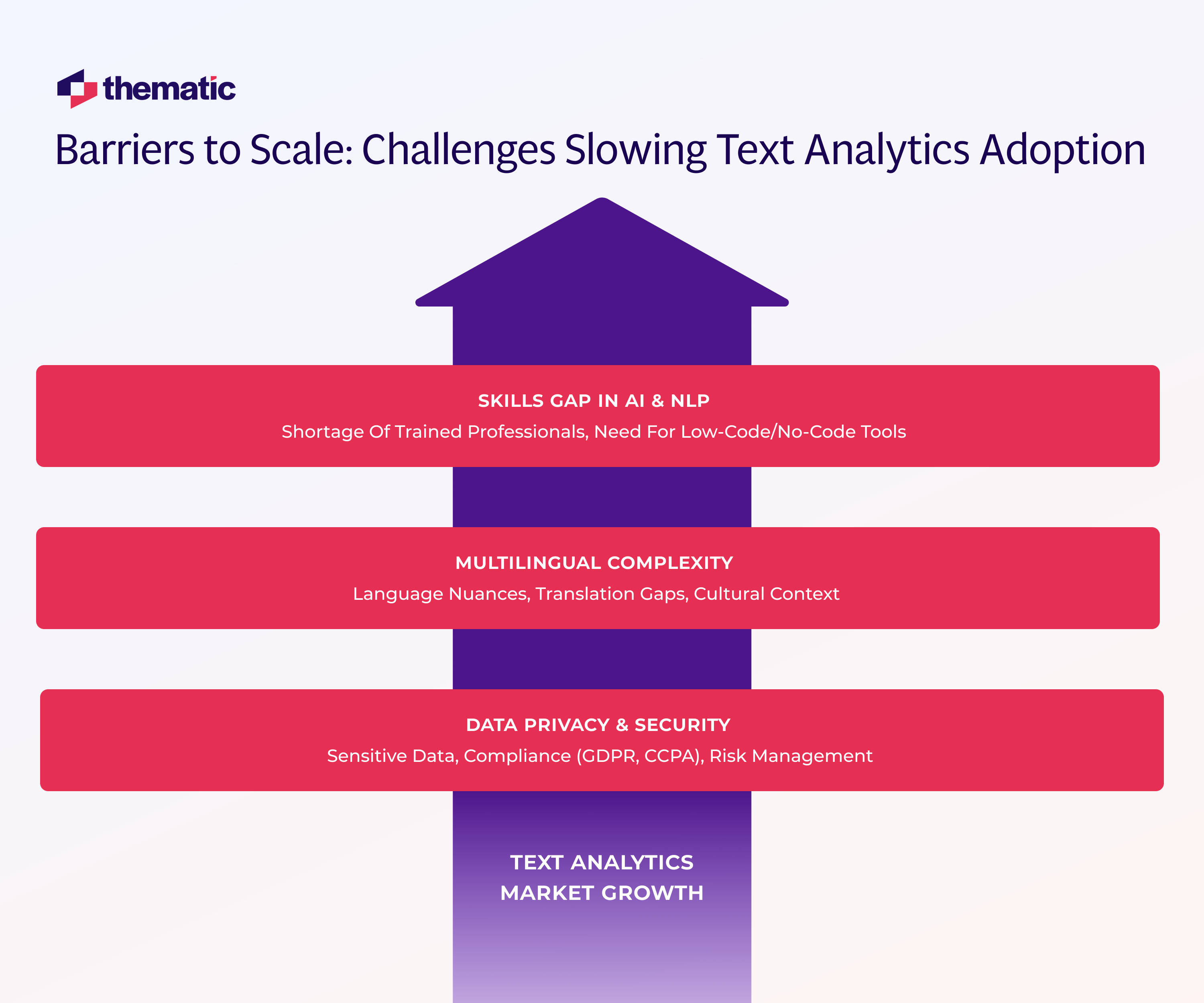
Future Opportunities and Trends
The text analytics market is poised for significant advancements, driven by several emerging trends:
1. Integration of Generative AI
GenAI is revolutionizing text analytics by enabling the creation of new content and insights from existing data. This technology enhances the ability to understand and generate human-like text, facilitating more nuanced analysis and content creation. The global GenAI market is projected to grow from USD 20.9 billion in 2024 to USD 136.7 billion by 2030, reflecting its expanding role across industries.
2. Expansion into Voice-Based Applications and Chatbots
The proliferation of voice-activated technologies has led to increased adoption of text analytics in voice-based applications and chatbots. Businesses can extract valuable insights, improve customer interactions, and enhance user experiences by analyzing spoken language. The AI voice generators market, valued at USD 4.9 billion in 2024, is expected to reach USD 54.54 billion by 2033, underscoring the growing significance of voice analytics.
3. Real-Time Analytics and Automated Decision-Making
The demand for real-time data processing has led to the integration of text analytics with automated decision-making systems. This synergy enables organizations to analyze data streams instantaneously and make informed decisions promptly. Advancements in edge computing facilitate this trend, allowing for immediate responses in dynamic environments.
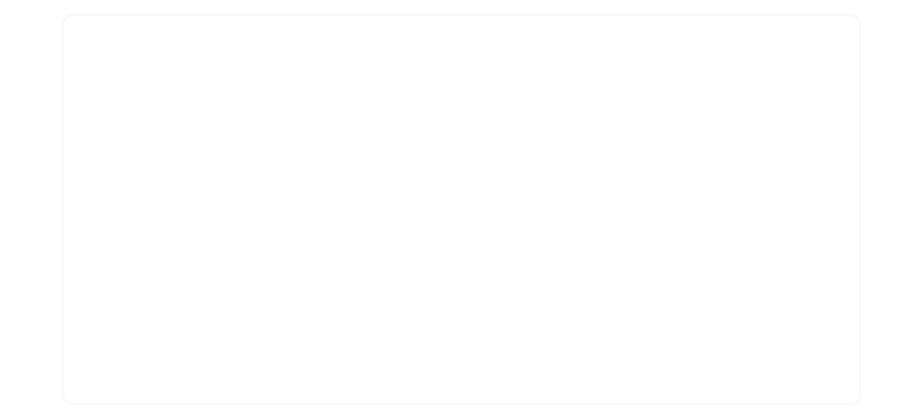
Thematic
AI-powered software to transform qualitative data into powerful insights that drive decision making.
The Bottom Line
The text analytics market is growing—fast. It offers businesses massive opportunities to harness unstructured data for strategic insights.
At this time, it makes sense for businesses to keep up with the trend if they want to stay competitive (and ahead). Of course, this also means finding the right partner—text analytics tools.
Thematic is an AI-powered platform that transforms open-ended text into accurate, layered insights, allowing teams to make informed, customer-led decisions.
Experience first-hand how it would work on your data. Try Thematic now!
Stay up to date with the latest
Join the newsletter to receive the latest updates in your inbox.