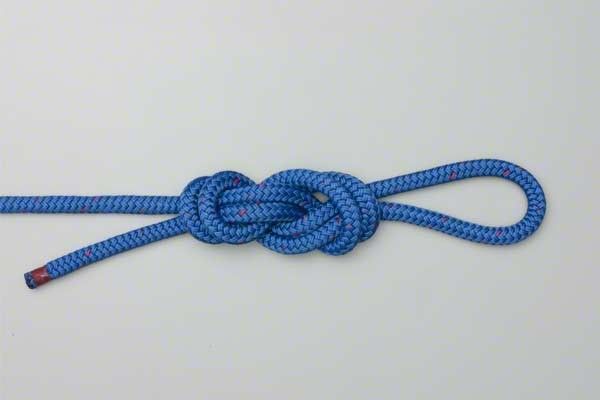
Thematic Analysis for Feedback: The Secret Weapon Most Companies Miss (Part 5/5)
Discover Thematic Analysis, the ONLY text analysis approach that's accurate, fast, and transparent. Learn how it outperforms other methods and uncovers hidden insights in your feedback.
Over the past few weeks, we’ve reviewed several Text Analytics approaches to feedback analysis: word spotting in Excel, manual rules, text categorization, and topic modelling. Now, it’s time for Thematic Analysis.
All of the former approaches mentioned have disadvantages. In the best case, you’ll get OK results only after spending many months setting things up. And you may miss out on the unknown unknowns.
The cost of acting late or missing out on crucial insights is huge! It can lead to losing customers and stagnant growth. This is why, according to YCombinator (the startup accelerator that produced more billion dollar companies than any other), “whenever you aren’t working on your product you should be speaking to your users”.
After Thematic participated in their programme, we’ve been asked for advice three times via a survey, once via a personal email, and also in person.YCombinator also use Thematic to make sense of all the feedback they collect.
1.Word Spotting for Text Analytics: Quick & Dirty (But When Does It Fail?)
2. Manual Rules for Text Analytics: Why They Often Miss the Mark
3. Text Categorization Demystified: Does It Really Deliver on Advanced Analytics?
4. Topic Modeling for Text Analysis: The Hype vs. Reality
👉 Thematic Analysis for Feedback: The Secret Weapon Most Companies Miss
When it comes to customer feedback, three things matter:
- Accurate, specific and actionable analysis
- Ability to see emerging themes fast, without the need of setting things up
- Transparency in how results are created, to bring in domain expertise and common sense knowledge
In my research, I’ve learned that the only approach that can achieve all three requirements is Thematic Analysis, combined with an interface for easily editing the results.
Thematic Analysis: How it works
Thematic Analysis approaches extract themes from text, rather than categorize text. In other words, it’s a bottom-up analysis. Given a piece of feedback such as “The flight attendant was helpful when I asked to set up a baby cot”, they would extract themes such as “flight attendant”, “flight attendant was helpful”, “helpful”, “asked to set up a baby cot”, and “baby cot”.

These are all meaningful phrases that can potentially be insightful when analyzing the entire dataset.
However, the most crucial step in a Thematic Analysis approach is merging phrases that are similar into themes and organizing them in a way that’s easy for people to review and edit. We achieve this by using our custom word embeddings implementation, but there are different ways to achieve this.
For example, here is how three people talk about the same thing, and how we at Thematic group the results into themes and sub-themes:
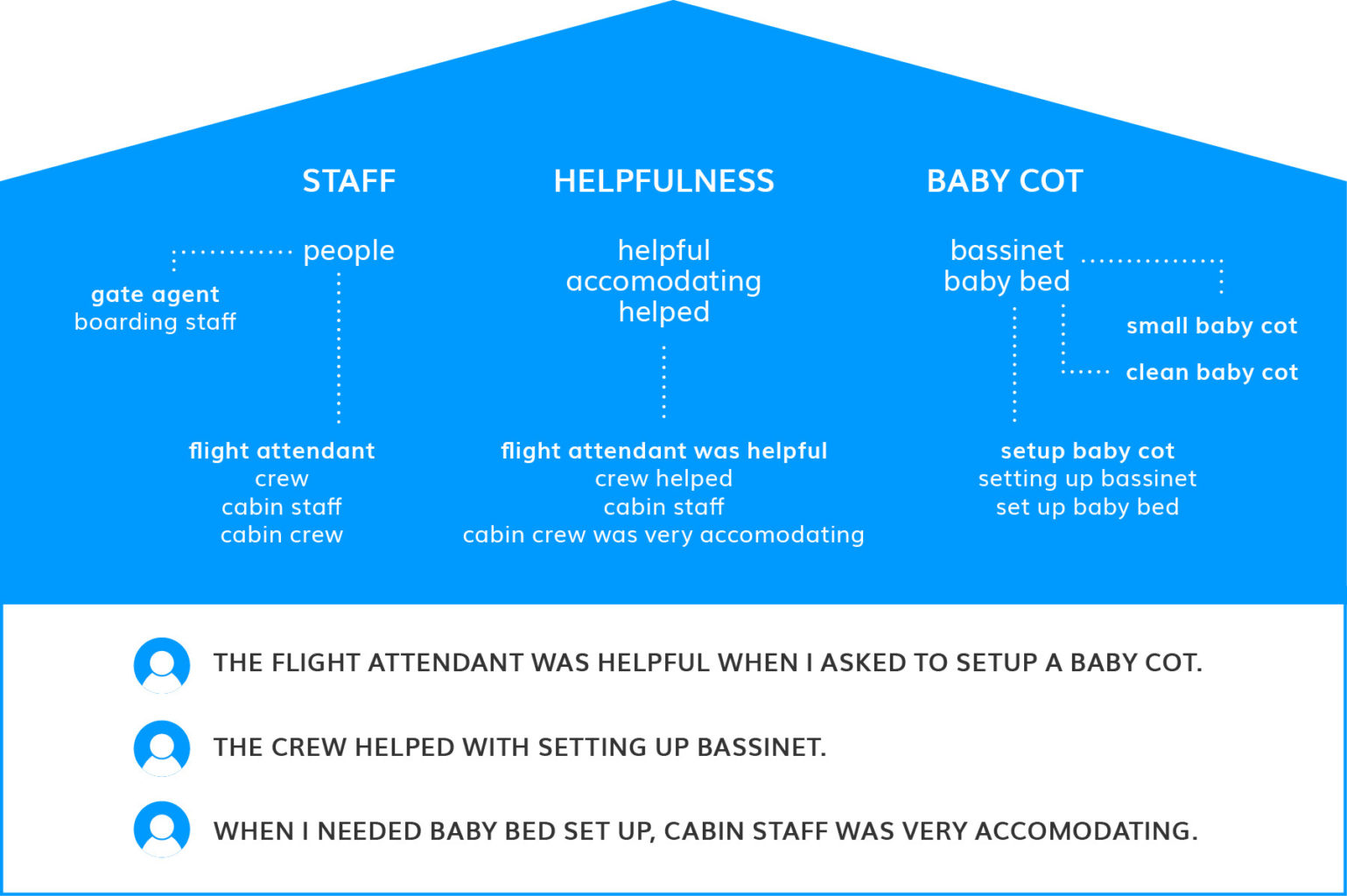
Advantages and disadvantages of Thematic Analysis
The advantage of Thematic Analysis is that this approach is unsupervised, meaning that you don’t need to set up these categories in advance, don’t need to train the algorithm, and therefore can easily capture the unknown unknowns.
The disadvantage of this approach is that it is phrase-based. Sometimes phrases cannot capture the meaning correctly. For example, when there is a complex narrative. For example, let's say the piece of feedback says: "It's the last time I'm calling you about this issue. No more!". This feedback indicates that the customer is likely to stop using the service, but capturing it is difficult. In supervized categorization, you could train a model that could recognize this theme. But it is still tricky to build. The good news is that Thematic Analysis works exceptionally well in 95% of feedback cases since most people try to be clear when they provide feedback.
Thematic Analysis is difficult to implement correctly from scratch. A perfect approach must be able to merge and organize themes in a meaningful way, producing a set of themes that are not too generic and not too large. Ideally, the themes must capture at least 80% of verbatims (people’s comments). And the themes extraction must handle complex negation clauses, e.g. “I did not think this was a good coffee”.
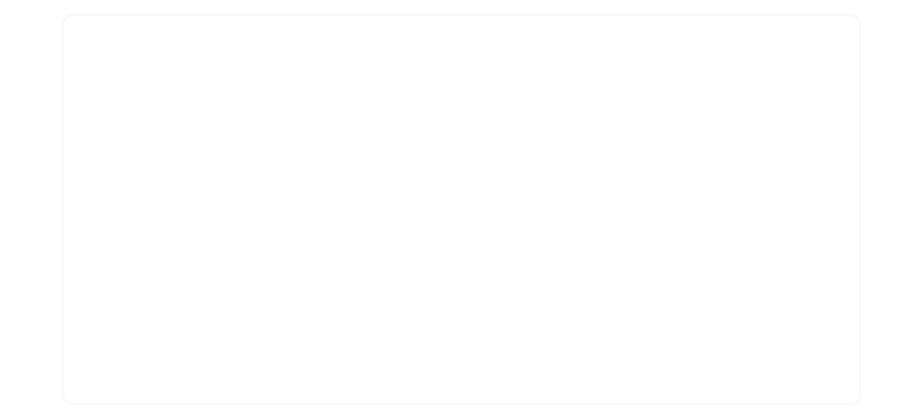
Thematic
AI-powered thematic analysis software to transform qualitative data at scale into valuable & shareable business insights
Who does Thematic Analysis?
Some of the established bigger players have implemented Thematic Analysis to enhance their Manual Rules approaches but tend to produce a laundry list of terms that are hard to review.
Traditional Text Analytics APIs designed by NLP experts also use this approach. However, they are rarely designed with customer feedback in mind and try to solve this problem in a generic way. For example, when we tested Google and Microsoft’s APIs we found that they aren’t grouping themes out of the box.
As a result, only 20 to 40% of feedback is linked to top 10 themes: only when there are strong similarities in how people talk about specific things. The vast majority of feedback is uncategorized meaning that you can’t slice the data for deeper insights.
At Thematic, we have developed a Thematic Analysis approach that can easily analyze feedback from customers of pizza delivery services, music app creators, real estate brokers and many more. We achieved this by focusing on a specific type of text: customer feedback, unlike NLP APIs that are designed to work on any type of text. We have implemented complex negation algorithms that separate positive from negative themes, to provide better insight.
Our secret sauce: Human in the loop
Each dataset, and sometimes even each survey question, gets its own set of themes, and by using our Themes Editor, insights professionals can refine the themes to suit their business. For example, Thematic might find themes such as “fast delivery”, “quick and easy”, “an hour wait”, “slow service”, “delays in delivery” and group them under “speed of service”. One insight professional might re-group these into “slow” and “fast” under “speed of service”, another into “fast service” > “quick and easy”, and “slow service” -> “an hour wait”, “delays in delivery”. It’s a subjective task.
I believe more and more companies will discover Thematic Analysis, because unlike all other approaches, it’s a transparent and deep analysis that does not require training data or time for crafting manual rules.
What are your thoughts?
Stay up to date with the latest
Join the newsletter to receive the latest updates in your inbox.