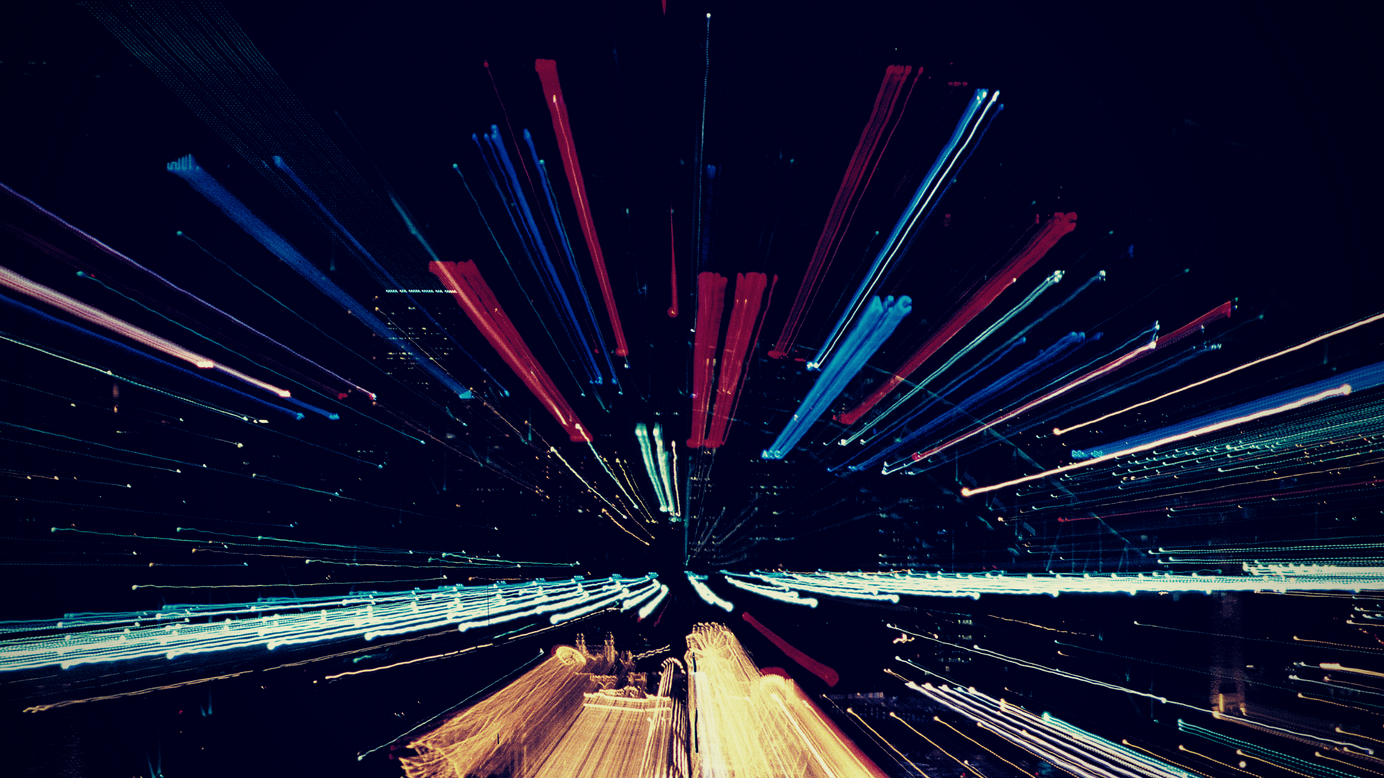
How we use our own platform and Chrome extension to centralize & analyze feedback
Customer feedback doesn't have all the answers. But it has critical insights for strategy and prioritization.
Thematic is a B2B SaaS company. We aren't swimming in feedback. Every piece of feedback counts. Collecting and analyzing this feedback requires a different approach.
We receive feedback from many places:
- our in-product NPS survey
- customer surveys
- customer interviews
- public reviews on G2 and Capterra
- sales & customer conversations via email or calls
Different teams collect this feedback, which opens questions:
So, how do we bring all this feedback together? How do we paint a single picture of what customers want and share it across the company? How do we identify issues at different points of customer journey and act on them?
You might be asking yourself the same questions. So, here's what helped us.
For one part of this process, we are using our own software Thematic. Yes, we are dogfooding! For the other part, we use a new tool we created called Product Feedback Collector. It's a free Chrome extension, which you also might find useful!
1. Why you need a central feedback repository
Customer feedback management platforms help push surveys to customers and get them to respond. But most platforms only provide analytics for the data they collect, e.g. surveys. This makes getting a unified view of what customers are saying impossible.
For example, we have a dashboard summarizing our NPS feedback. But how do we reconcile these insights with those from unsolicited online reviews? Or how do we align the team on priorities, if sales conversations tell a different story?
A central repository of feedback is the answer. It could be a data warehouse. But we've seen other companies use many work arounds. Some use giant spreadsheets, others product roadmap tools. Some use adapted wikis/workspace tools like Airtable or Notion to become feedback repositories. The problem with these solutions is that they don't analyze feedback for you. You have to tag feedback yourself. But worst of all, you need to manage tags by hand.
Our own solution Thematic can not only centralize feedback, but also analyze it. We use it in combination with a spreadsheet that helps us gather feedback from everyone on our team.
2. How we bring all feedback into one repository
Some of the feedback sources are easy to bring into Thematic. You connect via one of our integrations, and pull feedback daily for analysis.
To capture tidbits of feedback mentioned on calls and in emails, we use a tool we built in-house. It's called Product Feedback Collector and is a Chrome extension. It's connected to a Google Form, which is in turn collected to a spreadsheet.
With the chrome extension installed, when a customer shares feedback via email, one of us highlights the text, right-click, and 'Send Feedback to Team'.

This opens a form with the highlighted text already inserted, and then you add any other necessary information into the form. E.g. we collect; company name, how critical is the feedback, and what is the type of feedback.
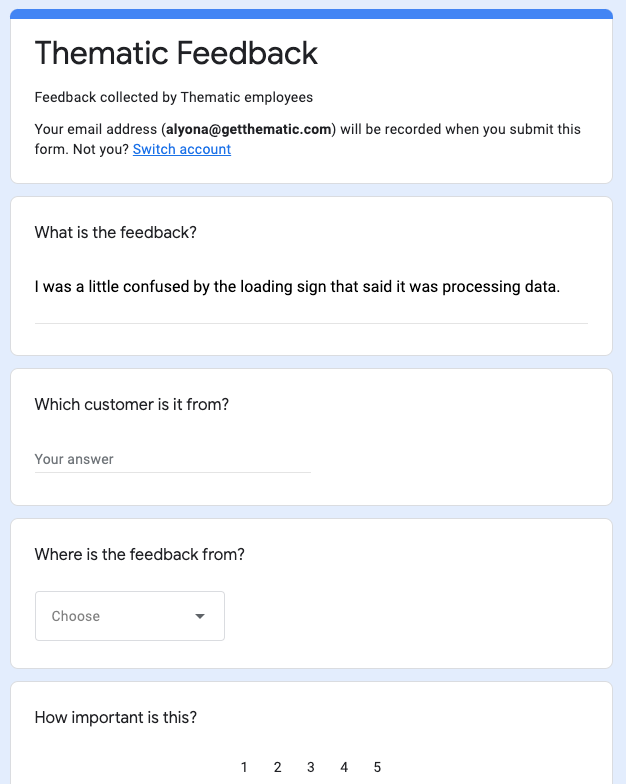
Thematic pulls the daily contents of the connected Google Sheet.
We could combine this feedback with our NPS and survey feedback via a workflow. But instead, we use the same Chrome extension to collect feedback from other sources, e.g. our G2 reviews.
This way, we can mark who the customer is and the importance of their feedback. Their identity might not be obvious but we can guess it.
For larger companies, this wouldn't be workable. But for a company of our size, it works well.
3. Getting the insights from the feedback
Our next step is to analyze the feedback and discover insights.
Step 1 is to find themes in feedback.
The recommended approach here is coding each piece of feedback based on what it is about. We use our own solution Thematic for this.
For example, here is a piece of feedback tagged with themes & sentiment in Thematic:
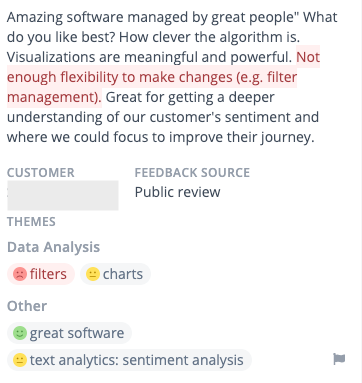
Thematic's self-supervising AI discovered these themes as they emerged from the data. Then our team has adjusted them in our Themes Editor. They include core features of the product, e.g. Text analytics and Data analysis. And general themes of interest to our users, e.g. Insightfulness or Interface.
Step 2 is to segment feedback by importance and look at the most common issues our core users are facing.
Core users are those who love our solution. We applied the idea from Sean Ellis' product market fit survey to understand which users would be disappointed if they no longer could use Thematic. In some cases, we asked via a survey. In other cases, we asked on a call or observed customer behavior. We value feedback of our core users more, and so we segment them out in Thematic.
Here you can immediately see the difference in high-level themes between core and non-core users, e.g. difference in mentions of Pricing.
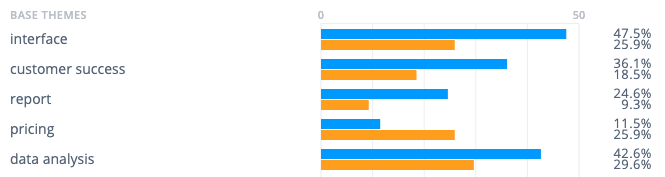
Step 3 is to identify what to focus on and track progress over time.
Two months ago, we used Thematic to identify our filtering feature as one of the important issues. The initiative that followed was a re-design of the UI.
Next question is clear: Has our work made a difference?
If people would keep mentioning filters in a negative way, we would need to keep iterating. But so far we see a promising trend. Only one person commented on filters this month.
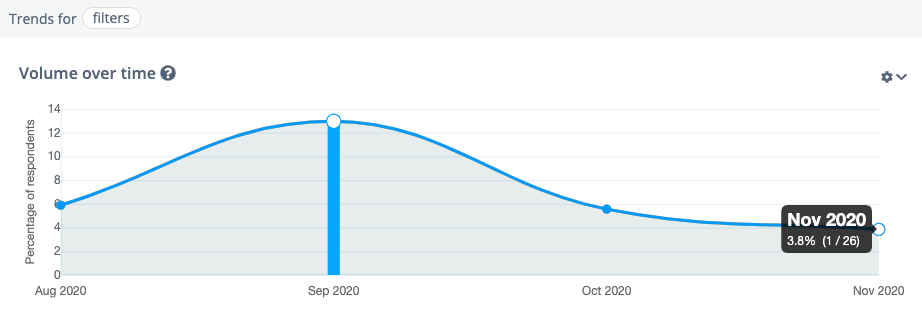
This is our approach, but we'd love to hear from you. What worked well and what didn't work at your company?
Stay up to date with the latest
Join the newsletter to receive the latest updates in your inbox.