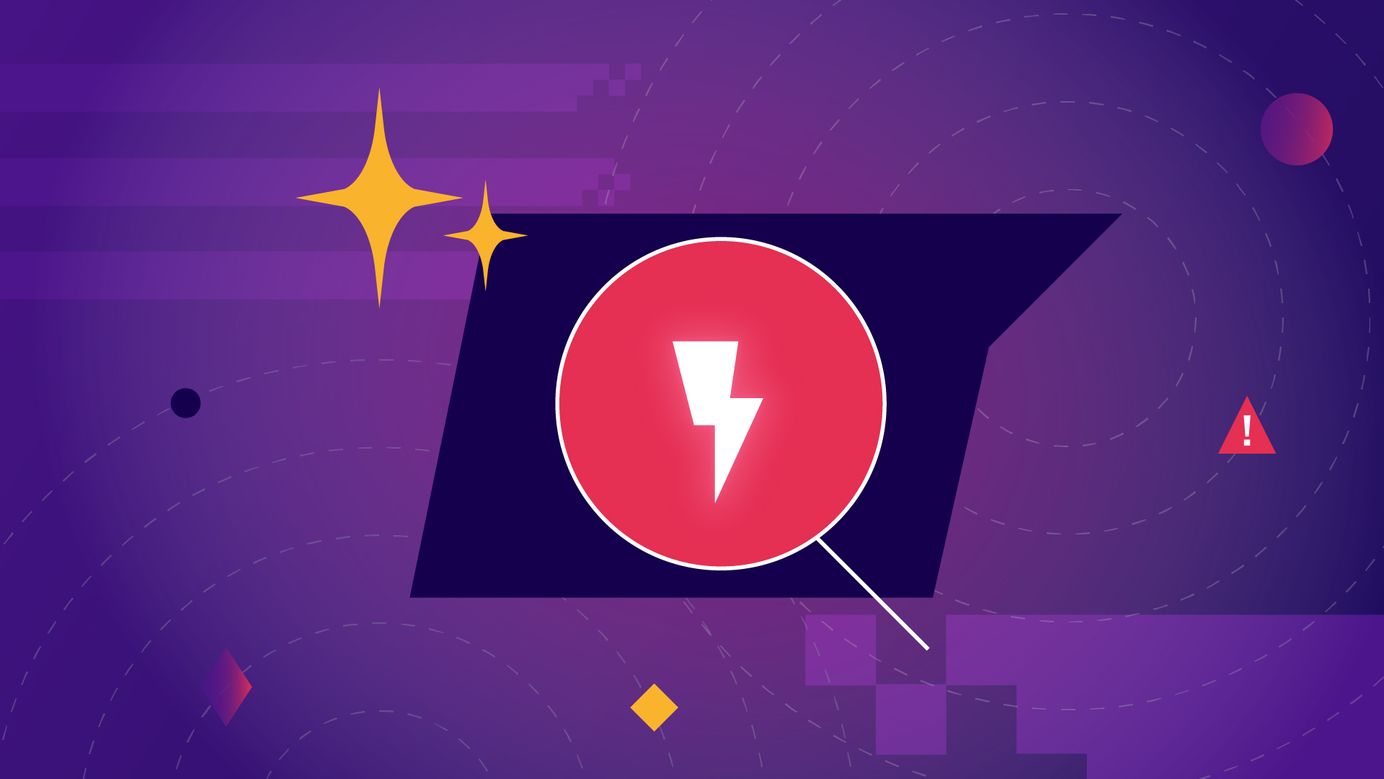
How accurate is Thematic at analyzing customer feedback?
In discussions with potential customers, we often hear the question, "How accurate is Thematic?
This usually comes up for two reasons:
“I’ve been doing the analysis myself. I don’t want to compromise the accuracy when handing this task over to an automated solution.”
“I realize that doing things manually isn’t the best approach. I tried different solutions. They all produced generic results that aren’t useful. Does a solution that’s sufficiently accurate even exist??”
Both are valid reasons, and we are eager to provide an answer.
But let’s step back, when it comes to analyzing feedback, what does “accurate” really mean?
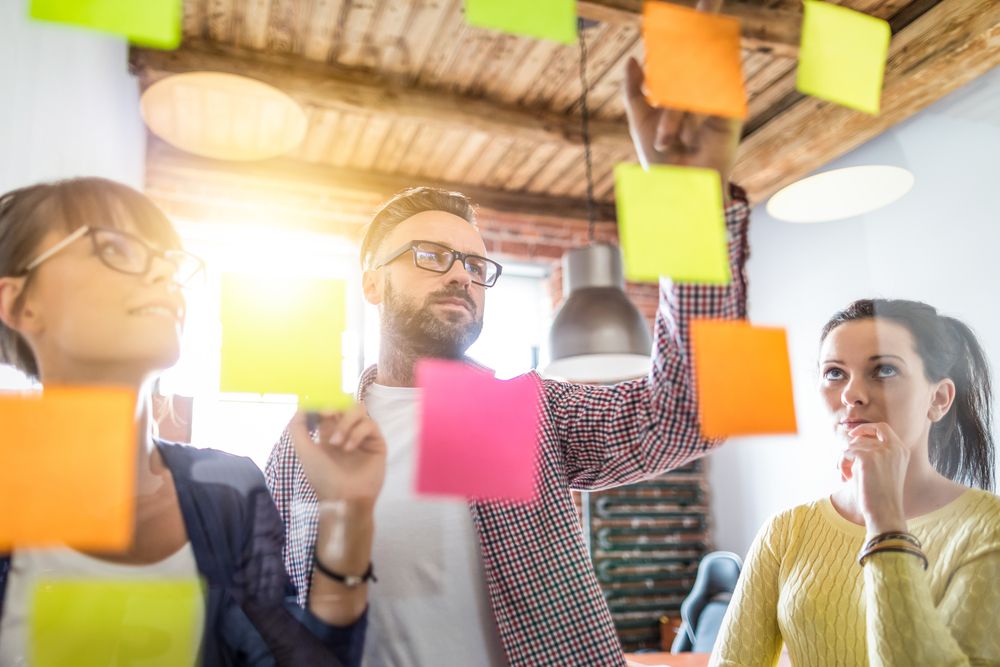
Why is accuracy alone meaningless?
Having published dozens of academic publications that evaluate accuracy, I can geek out on this topic for hours. But, in the context of customer feedback, these three key points matter the most:
100% accuracy is a myth – and it’s subjective anyway
Even people disagree when deciding how to code a piece of feedback.
The level of agreement between people is called consistency, and it usually ranges between 40 and 70% depending on the task.
So, don’t expect a generic number as an answer.
Usefulness trumps accuracy
Imagine this situation: All feedback theoretically falls into billing, price, or customer service.
It’s relatively easy to be highly accurate, because there are only 3 choices.
But is the analysis useful?
Unlikely, especially because you already knew these categories in advance.
A solution with 100% accuracy that doesn’t get more specific than billing is actually worse than a solution that has an 85% level accuracy, but tells you which comments are saying “billing date is inconvenient” vs. “billing terms have improved” vs. “billing isn’t accurate.”
Ultimately, it’s more important to get specific and actionable themes, rather than chasing an accuracy number. More on this later.
Ease and speed of refinement matters too
Refinement is a step that many people overlook.
No matter how “accurate” an approach might be, there are always human perspectives and tacit business knowledge that need to be incorporated in order to make the output actionable.
Oftentimes this knowledge and perspective only becomes apparent after a review of the first results.
Let’s say we have evaluated two solutions:
Solution A is 70% accurate in finding themes. Its decisions are transparent, and anyone can easily refine them to make the results more actionable and relevant. Within a couple of hours you can get to nearly perfect accuracy.
Solution B is 80% accurate, but it’s a black box. It takes weeks of data science labour to refine the output.
I know which one I’d rather choose!
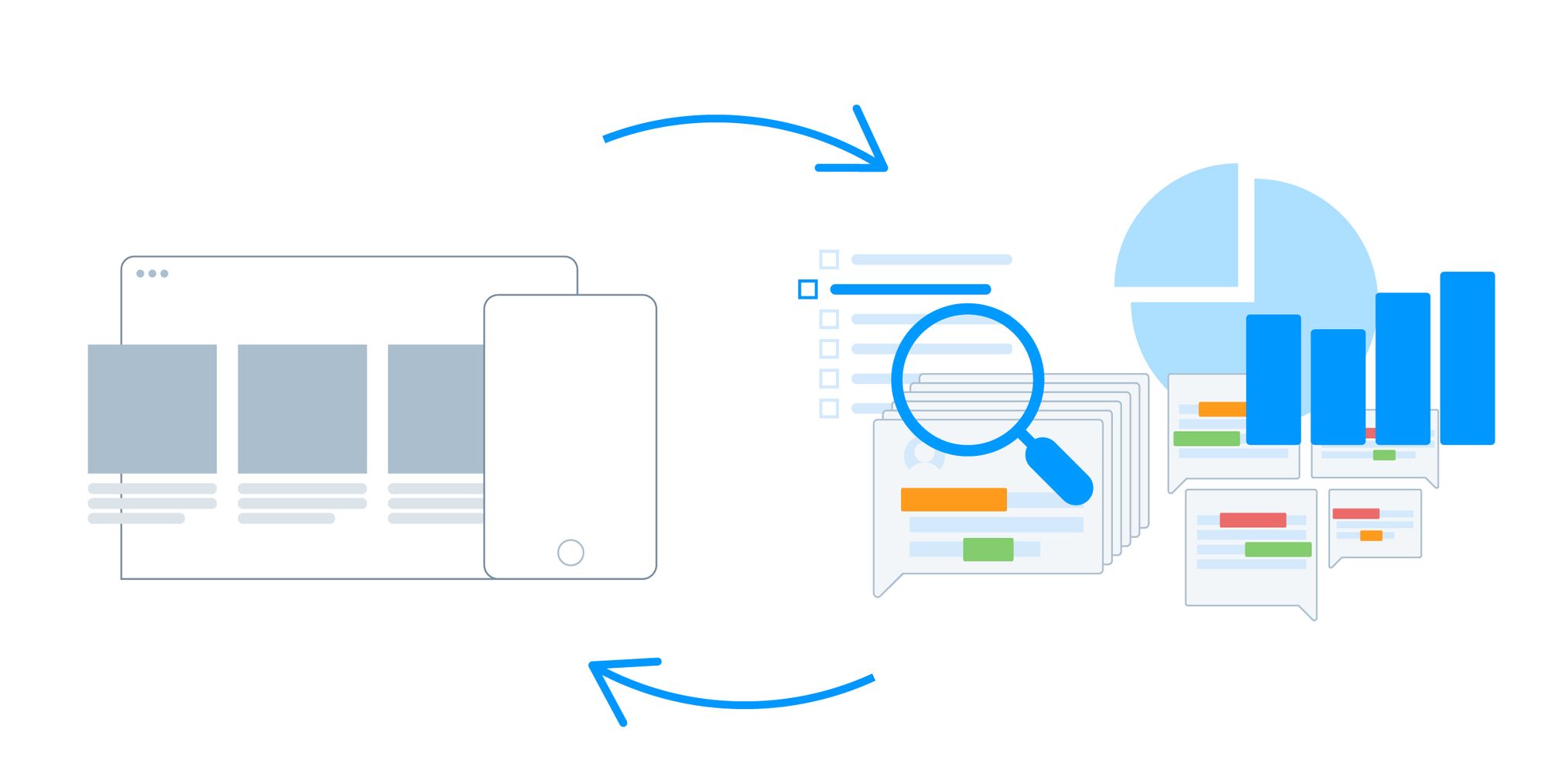
How accurate is Thematic?
At Thematic, we’ve designed an interface that makes it easy to quickly refine themes, and have built algorithms that learn from this process, delivering improved results in future projects.
Other solutions require extensive manual configuration, and with manual feedback analysis (which some might claim is the most accurate approach of all) refining themes can mean starting from scratch.
Previously, we’ve published a guide on how to evaluate analysis of customer feedback, and a white paper on the advanced evaluation of Thematic.
In the white paper we used two different customer feedback datasets. Each one had themes from 4 people.
We calculated people’s consistency with each other, and compared Thematic’s average consistency with them.
Our research shows that Thematic can easily be more accurate than people, whose analysis can suffer from personal bias or being tired.
Please note that Thematic offers an easy way of editing themes to ensure highest accuracy possible. This process takes between 30 minutes and 2 hours.
Try to quickly eyeball the themes that Thematic found on one of the datasets: “How can we improve things in our business school?”
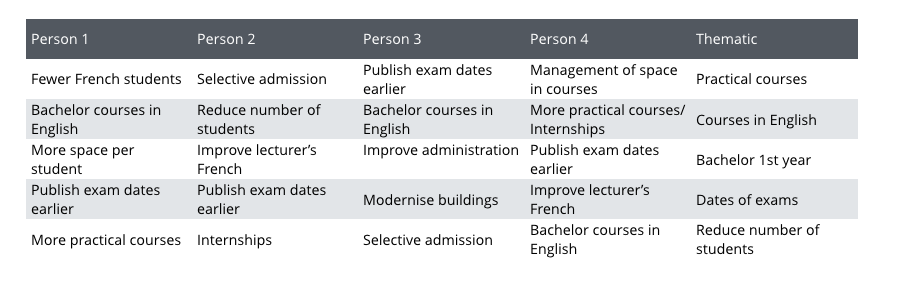
The advanced evaluation results were as follows:
- Pre-editing, Thematic was slightly less consistent than people. Post-editing, Thematic was better than 2 out of 4 people.
- Thematic’s results were more specific because not only it discovered themes, but also sub-themes. Where people just provided the general categories, Thematic found both themes and sub-themes.
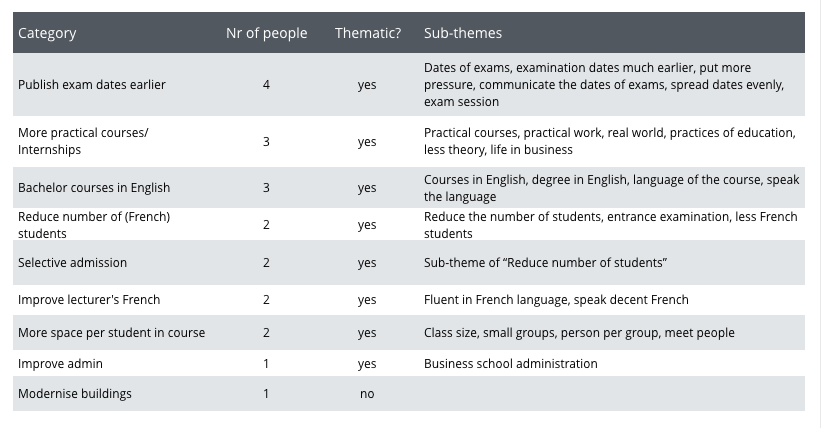
Why is Thematic so accurate?
Thematic’s proprietary solution was designed specifically for the analysis of customer feedback by the world’s leading experts in this field.
The research is lead by Thematic’s co-founder and CEO Dr. Alyona Medelyan, who has a PhD in keyword extraction and more than 2500 academic citations in Natural Language Processing.
Accuracy is also ensured by allowing a person to refine Thematic’s choices: themes can be regrouped, renamed and deleted.
This refinement is optional, because even without help Thematic gets 80-90% of the way.
Thematic has also other advantages: It learns the use of language not just from millions of pieces of feedback it collects, but also from all the human refinements it has captured over the past 5 years.
Best thing: It does not require pre-defined categories or annotated data.
Takeaways
- Solutions as accurate (if not more accurate) as people exist!
- You don’t need to compromise the quality of analysis when automating this task.
- Usefulness (specificity and actionability) matters more than accuracy alone.
- Ease and speed of refinement matters too
- Don’t just ask for accuracy, eyeball the results to get a sense of how useful and actionable the themes are.
At Thematic we care about accuracy a great deal! And we guarantee human-competitive results.
Thematic Newsletter
Join the newsletter to receive the latest updates in your inbox.