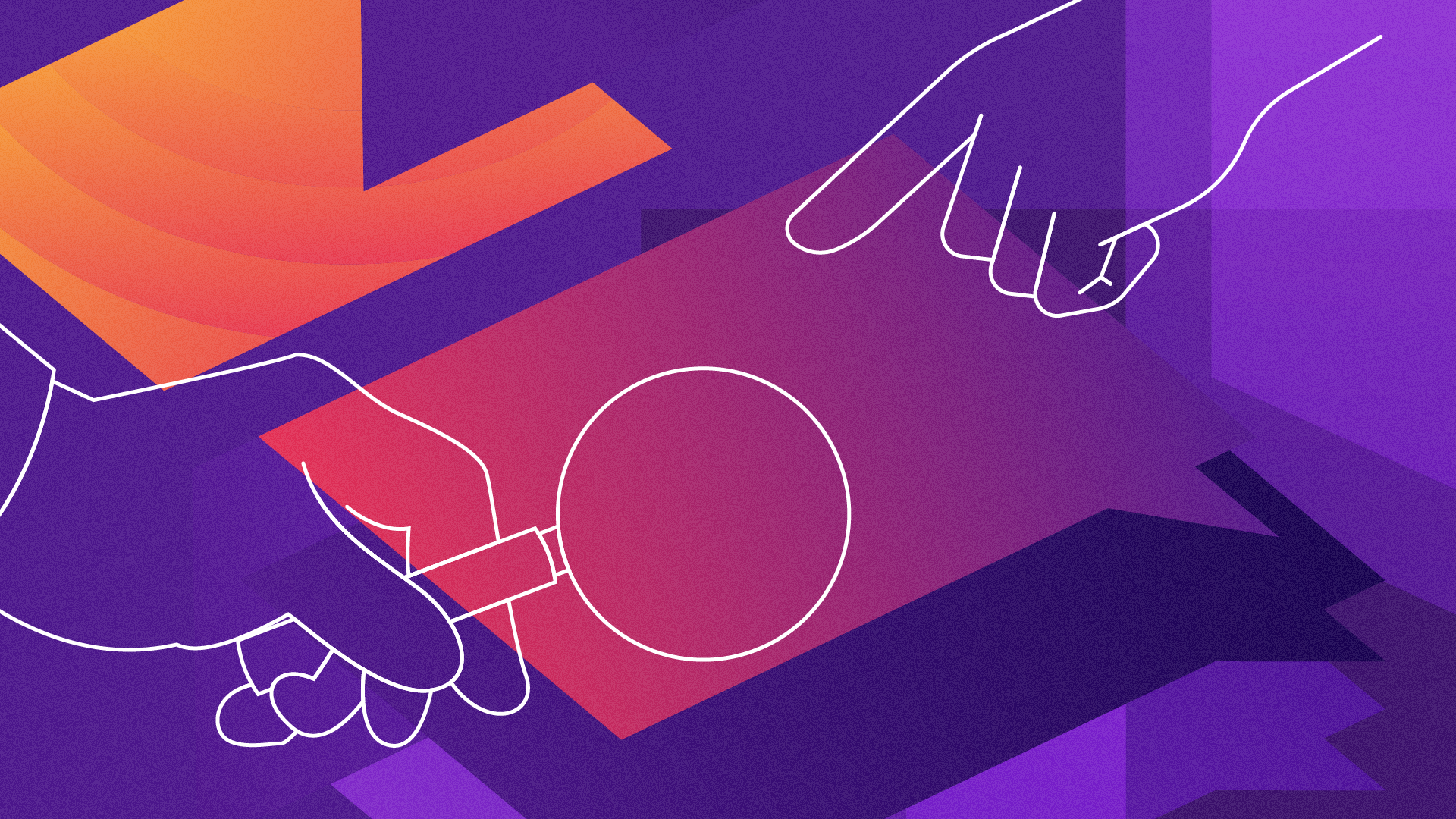
Content Analysis vs. Thematic Analysis: A Comprehensive Guide
Uncover the differences between content analysis and thematic analysis, learn which method is right for your research, and discover powerful tools to streamline your analysis.
On the surface, content analysis and thematic analysis sound the same. They both use terms like "coding" and "themes," and both share the goal of extracting meaning. For many, content analysis and thematic analysis feel interchangeable.
But they're not.
While they both start with qualitative data, content analysis and thematic analysis are different in the way they analyze qualitative data and arrive at their results.
So, what's the difference? What makes content analysis different from thematic analysis? This is where we find out.
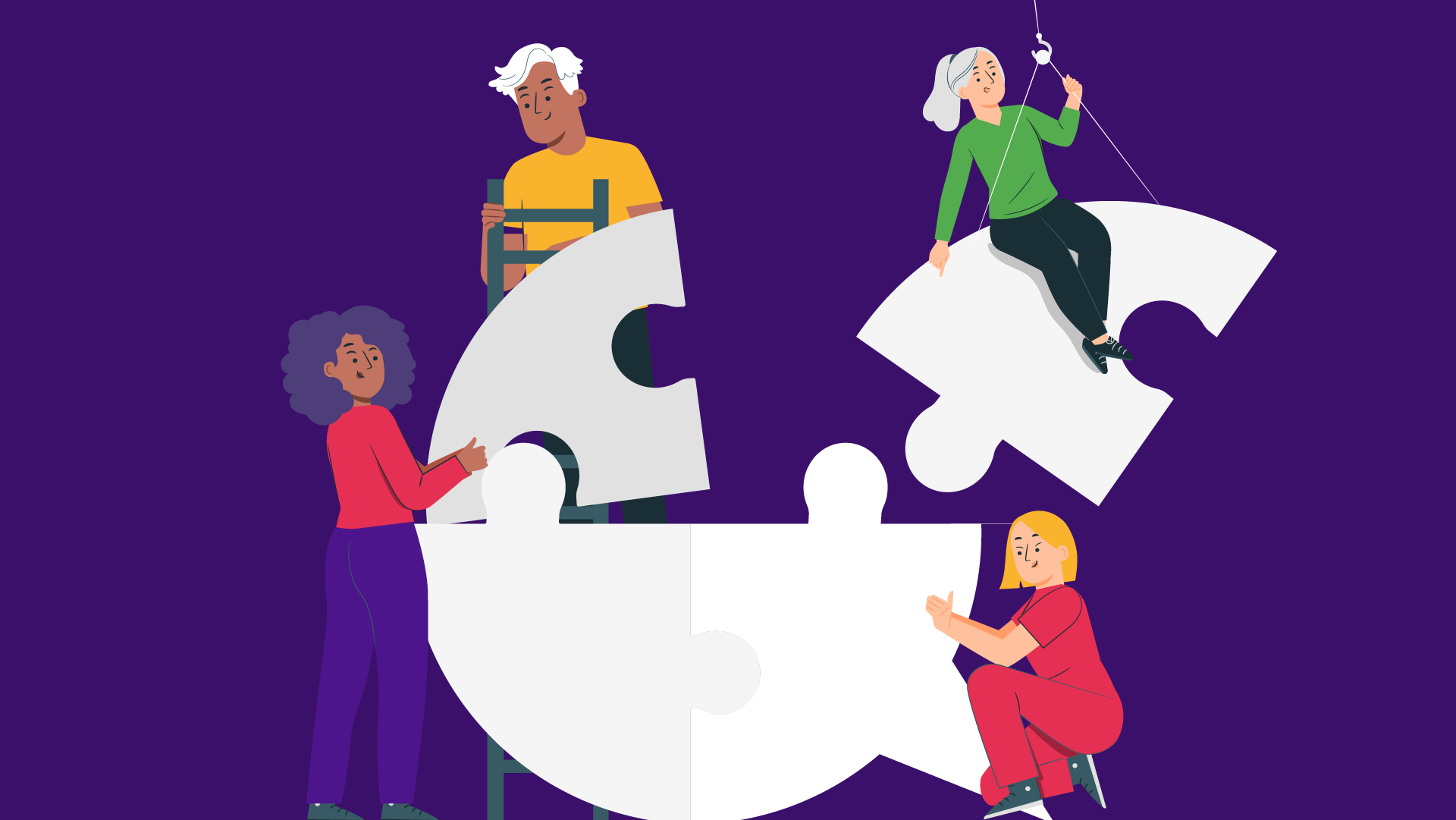
What is content analysis?
Content analysis is a research method used to identify, quantify, and analyze patterns. It's a systematic approach that often involves identifying and analyzing data, breaking down data into smaller units, categorizing them, and then counting the frequency of these categories.
Why do we need to do this?
By counting frequency, the researcher can draw conclusions about the content's meaning, its potential impact, and even the intentions behind it.
How does qualitative data frequency allow the researcher to draw conclusions?
In content analysis, frequency is a key factor for drawing meaningful conclusions, because it reveals patterns and trends within the entire data set. Frequency can reveal important factors, like dominant themes or key messages. Ommission can also reveal biases in the one conducting content analysis, and changes in frequency over time can indicate shifts in attitudes, opinions, and trends.
Examples of research questions for content analysis
- "What are the dominant themes in news coverage of a particular event?"
- "How do social media users express their opinions about a specific product?"
- "What are the most common keywords used in customer reviews?"
- "How has the portrayal of gender roles changed in advertising over time?"
Researchers frequently use similar research questions when employing complementary qualitative techniques within content analysis. This particular phenomenon is because the method aims to identify and measure recurring patterns or trends in communication. They focus on the surface-level information that is directly observable in the data, rather than trying to interpret hidden meanings or intentions.
What is a thematic analysis?
In contrast to content analysis, thematic analysis goes beyond counting words or phrases, which is precisely what content analysis does. Thematic analysis focuses on identifying and interpreting patterns of meaning (i.e., themes) that emerge from the data.
These themes represent the underlying ideas, concepts, or experiences that are expressed by the participants or conveyed in the text.
Differences between Thematic Analysis & Content Analysis
While both content and thematic analysis deal with turning qualitative data into something measurable, how they get there and the focus level of interpretation are what differentiate them.
Here's a quick rundown of the differences.
What kind of questions does thematic analysis ask?
- "What are the experiences of people living with a chronic illness?"
- "How do employees perceive their workplace culture?"
- "What are the factors that influence consumer behavior?"
- "How do different social groups represent themselves in the media?
These questions share a common characteristic—they seek to identify themes and interpret patterns of meaning (themes) reporting patterns that emerge from the data. They focus on understanding the underlying reasons, motivations, or experiences for complex phenomena expressed in the data rather than simply quantifying the frequency of specific words or phrases.
But wait! There's more.
5 Key Differences between Content Analysis and Thematic Analysis
Here are five key differences between content analysis and thematic analysis.
1. Research Focus
As we pointed out earlier, content analysis focuses on the manifest content (which is the surface-level information that is directly observable in data). For example, content analysis will look for specific words, phrases, or visual elements. It works on an 'exact match' rather than context based (which is more thematic analysis).
In contrast, thematic analysis focuses on latent content, which is more aligned with uncovering the meaning and deeper significance of data. It looks at implicit messages, ideas, or concepts rather than just taking everything at face value.
2. Data Analysis Approach
Content analysis is primarily a quantitative method, which, in a nutshell, involves counting and measuring the frequency of specific elements in the data. From this, researchers can identify patterns and trends to make comparisons between different datasets.
For example, demographic A liked the product (identified through the frequency of positive ratings), while demographic B did not (through the binary opposite of negative ratings).
Thematic analysis, however, is a qualitative method that is more focused on identifying and interpreting patterns of meaning in the data. This is achieved by employing a more subjective and interpretive approach and digging into the nuances of language itself to figure out the real reasons behind the experiences and decisions that people make.
For example, demographic A liked the product not because of the product itself but because it's currently a trend on social media. In contrast, demographic B did not like the product because of demographic A's overhyping of it.
A popular energy drink company recently launched a new flavor targeting younger consumers (Demographic A). Initial sales figures were high, but online reviews were mixed.
👉 Content analysis of online reviews revealed a high frequency of positive words like "delicious" and "refreshing" from Demographic A, suggesting they enjoyed the taste. However, there was also a significant number of negative reviews from Demographic B (older consumers) using words like "too sweet" and "artificial."
👉 Thematic analysis delved deeper into the reviews, uncovering that Demographic A's positive reviews often mentioned the drink's association with popular influencers and viral social media challenges. This suggested that their purchase was driven more by social trends than actual taste. Conversely, Demographic B's negative reviews often expressed disappointment that the drink didn't align with their preferences for less sugary and more natural beverages, and some even mentioned feeling excluded by the marketing tactics aimed at a younger audience.
3. Level of Interpretation
Content analysis is more descriptive because it focuses on what is being explicitly stated or depicted in the data. While it can be handy, it does present a problem: it only provides a surface-level understanding of the content. Thematic analysis takes it further through its interpretive nature, and it's more interested in figuring out the underlying meanings and significance than the frequency of things.
4. Flexibility
When it comes to content analysis, categories (or codes) are predetermined. They also don't typically change during the analysis process. While this can be beneficial in ensuring consistency and reliability in the analysis, it can also limit the ability to discover unexpected themes and patterns in the data.
Thematic analysis, on the other hand, is more flexible and iterative by allowing for the emergence of themes from the data itself. Codes and themes are developed and refined throughout the analysis process. This flexibility allows for a more open-ended and exploratory approach, but it can also make it more challenging to ensure consistency and reliability in the analysis.
Thematic analysis can make it more challenging to ensure consistency and reliability because it relies on the researcher's interpretation of the data (especially when done manually), which can introduce subjectivity and potential bias. Different researchers might identify different themes or interpret the same theme differently, leading to inconsistencies in the analysis.
AI-driven thematic analysis tools, like Thematic, leverage machine learning algorithms to automate and enhance the process of identifying and interpreting themes in qualitative data. These tools can significantly reduce the time and effort required for manual coding, allowing researchers to analyze larger datasets more efficiently.
How does it work?
Thematic can automatically cluster similar pieces of text together, suggesting potential themes based on patterns in the data. This can help researchers quickly identify recurring ideas or concepts that might not be immediately apparent through manual coding.
5. Research Application
Content analysis is a go-to method for researchers looking to test existing theories. Its structured, number-focused approach is perfect for checking if communication data matches specific predictions.
Thematic analysis, however, is the tool of choice for those aiming to break new ground. Its adaptable and interpretive nature makes it ideal for diving into complex issues and uncovering fresh insights.
Content Analysis vs. Thematic Analysis: Key Similarities (and Why It Matters)
Here are the top 5 reasons:
- Shared Terminology: Both methods use terms like "coding" and "themes," leading to confusion about their distinct approaches.
- Common Goal: Both methods aim to extract meaning from qualitative data, further blurring the lines between them.
- Data Source: Both methods can be applied to various types of qualitative data, such as text, images, audio, and video.
- Coding Process: Both methods involve a systematic process of coding or categorizing data, although the focus and interpretation of codes differ.
- Research Applications: Both methods can be used in a variety of research fields, including social sciences, marketing, media studies, and healthcare.
5 Tools to Help You with Content Analysis and Thematic Analysis
1. Thematic
An AI-powered tool specifically designed for thematic analysis, Thematic automatically identifies and organizes themes in your qualitative research data, saving you time and effort. Its user-friendly interface and intuitive visualizations make it easy to understand and share your qualitative research and findings anywhere.
Best for: thematic analysis of customer feedback, social media data, open-ended survey responses, and other types of text-based data.
Price: Available on request. A free guided demo is available.
2. NVivo
A popular choice for qualitative data analysis, NVivo offers a comprehensive suite of tools for coding, organizing, and visualizing data. It supports both content and thematic analysis, allowing you to quantify and interpret patterns in your data. NVivo's advanced features, such as mixed-methods analysis and project collaboration, make it a powerful tool for research teams.
Best for: experienced researchers who need a versatile research tool used for in-depth, qualitative research method and analysis, including both the quantitative or qualitative method methods, content analysis and thematic analysis.
Price: Paid software with various licensing options.
3. MAXQDA
Another comprehensive qualitative data analysis software, MAXQDA, offers similar features to NVivo, including coding, memoing, and visualization. It's known for its user-friendly interface and flexibility, making it a good option for both beginners and experienced researchers. MAXQDA also supports mixed-methods analysis, allowing you to combine both your qualitative data analysis, qualitative methods, and quantitative data.
Best for: Researchers who want a user-friendly tool for both content and thematic analysis, with the option to integrate quantitative and descriptive qualitative approaches, analysis methods and data sources.
Price: Paid software with various licensing options.
4. ATLAS.ti
ATLAS.ti is a powerful tool for qualitative data analysis that offers a wide range of features, including coding, linking, and visualization. It's particularly well-suited for large and often complex data projects, allowing you to manage and analyze vast amounts of data. ATLAS.ti also supports multimedia data, such as images and videos, making it a versatile tool for visual data in various research projects.
Best for: Researchers working on large-scale projects with diverse data types, including both content and thematic analysis.
Price: Paid software with various licensing options.
5. QDA Miner Lite
A free and open-source software for qualitative data to conduct thematic analysis, QDA Miner Lite offers basic features for coding and conduct thematic data analysis with. It's a good option for beginners or those on a budget who are looking for a simple and easy-to-use tool. While it may not have all the advanced features of other tools, it's a great starting point for learning the basics of content and thematic analysis.
Best for: beginners or budget-conscious researchers who need a simple tool for content or thematic analysis.
Price: Free
Which method is right for you? Content analysis or thematic analysis?
Choosing between content analysis and thematic analysis depends on your specific, research question, goals, research objectives, the nature of your data, and the resources available to you. Here's a breakdown of the key factors to help you make your decision on the most suitable method and research question.
Start with the requirements.
Before diving into which method is right for you, it's essential to outline your project requirements. This will help you determine which theme development method aligns best with your goals and resources.
1. Research Questions
What are you trying to find out? Are you looking to quantify specific elements in your own data set, or are you interested in uncovering deeper meanings and key themes?
2. Data Type
What kind of data are you working with? Is it structured data, like survey responses with predefined categories, or unstructured qualitative data sources, like open-ended interviews or social media comments?
3. Methodology
Based on your research questions and data type, what methodology will you use? Will you employ quantitative methods like descriptive statistics or inferential statistics, or will you opt for qualitative methods like thematic analysis or content analysis?
3. Resources
What resources do you have available to research question? Consider your budget, time constraints, and the expertise of your research team.
What kind of data have you got?
Structured vs. unstructured data
Content analysis is well-suited for structured data, such as survey responses with predefined categories or social media posts with hashtags. Thematic analysis is more appropriate for unstructured data, such as open-ended survey responses, interview transcripts, or social media comments, where you need to identify and interpret underlying themes.
Large vs. Small Datasets
Content analysis can efficiently handle large datasets due to its quantitative nature. Thematic analysis, being more qualitative and interpretive, is often better suited for smaller datasets where you can delve deeper into the nuances of the data.
Traditionally, thematic analysis was a go-to for smaller datasets, allowing for deeper dives into the details. With AI powered thematic analysis, you can now tackle even the biggest datasets with ease.
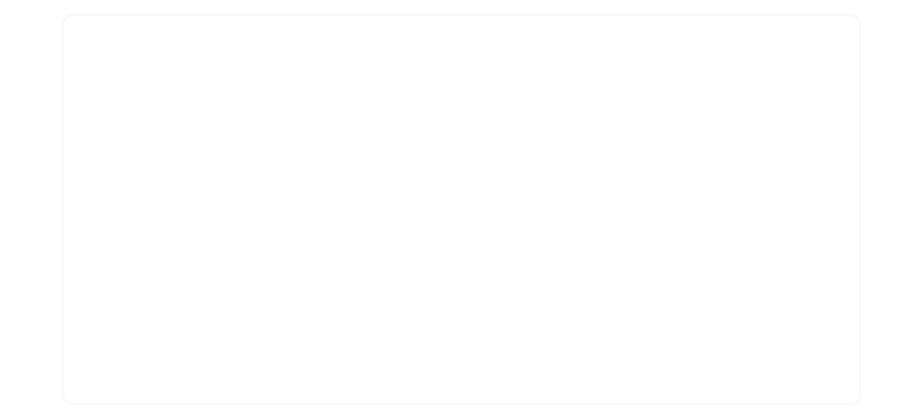
Thematic
AI-powered thematic analysis software to transform qualitative data into useful & actionable insights.
Human Resources and Employee Expertise
What's your resource and capacity bandwidth?
Time Constraints
Content analysis can be faster, especially with the help of software tools that automate the coding process. Thematic analysis, with its focus on interpretation and nuance, can be more time-consuming.
Expertise
Content analysis requires a qualitative research method and less interpretive skill, making it accessible to researchers with varying experience levels. Thematic analysis demands a deeper understanding of qualitative research methods and interpretive skills.
Budget
Some content analysis software tools can be expensive, while both thematic analysis and content can often be done manually or with free or low-cost tools.
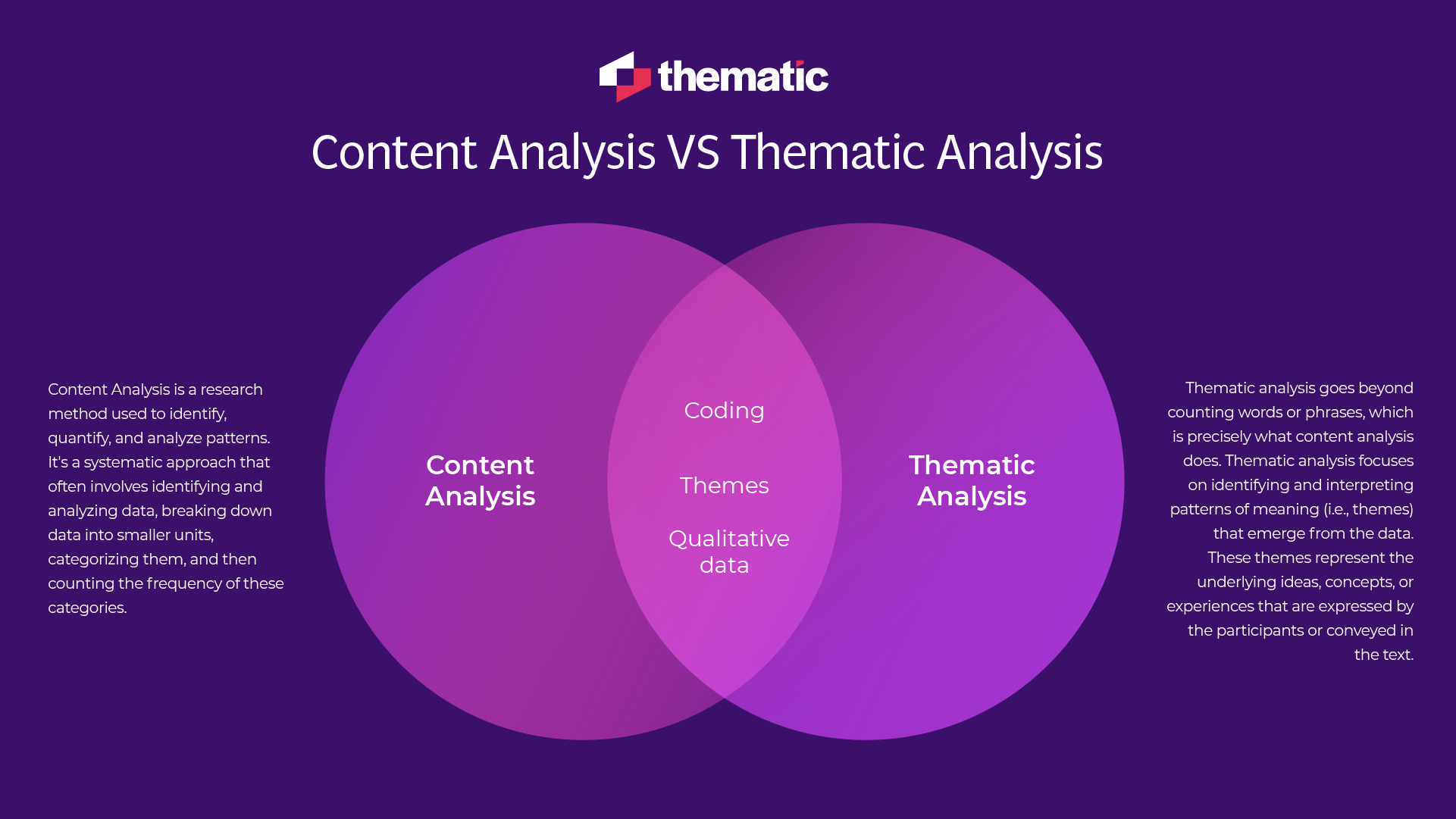
Where do I go from here?
The choice between the two isn't about one being "better" than the other, but rather about selecting the right tool for the job. Both content analysis and thematic analysis are valuable tools for extracting meaning from qualitative data, each with unique strengths and applications.
So, are you ready to embark on your qualitative research journey? Whether you're a seasoned explorer or a curious newcomer, Thematic is here to guide you. Don't miss out on the opportunity to experience the power of AI-driven thematic analysis firsthand. Sign up for our free guided demo today and unlock the hidden depths of your data.
Stay up to date with the latest
Join the newsletter to receive the latest updates in your inbox.