The Primary Methods of Qualitative Data Analysis
In academic research as well as in the business landscape, qualitative data analysis plays a crucial role in understanding and interpreting non-numerical data.
Qualitative data analysis helps us make sense of the stories and personal narratives. In the business context, qualitative data analysis turns customer feedback into an in-depth understanding of what matters to customers. Sharing the insights from this analysis with decision-makers helps them drive initiatives that improve customer experiences.
While quantitative data analysis focuses on numerical measurement and statistical analysis, qualitative data analysis delves into the rich and complex nature of human experiences and perceptions. When analyzed effectively, customer feedback can be transformed into actionable insights for every team across the company.
This guide will provide an in-depth exploration of the different methods employed in qualitative data analysis, as well as the steps involved and challenges encountered. We’ll also have a look at what QDA means in the business context and how to turn it into a high-powered tool for CX and product teams.
Understanding Qualitative Data Analysis Methods
Definition and Importance of Qualitative Data Analysis
Qualitative data analysis refers to the systematic process of examining and interpreting non-numerical data to gain meaningful insights and generate new knowledge. It’s what happens when you put a year’s worth of Amazon reviews into a thematic analysis engine, and end up with a thorough understanding of how users interact with your product (and half a dozen actionable insights to boot).
It involves dissecting text, images, videos, and other forms of qualitative data to identify patterns, themes, and relationships.
By capturing the nuances and depth of human experiences, the qualitative data analysis approach allows researchers to explore complex social phenomena that quantitative approaches cannot fully capture. It provides a rich and detailed understanding of social contexts, individual perspectives, and subjective experiences.
Qualitative data analysis methods offer an in-depth exploration of the hows and whys behind social phenomena, enabling researchers to gain a comprehensive understanding of complex social issues. It is incredibly valuable in fields such as sociology, anthropology, psychology, and education, where human behavior and social interactions are studied.
In these fields, researchers often seek to understand the intricacies of human experiences, and qualitative data analysis allows them to capture the complexity of these phenomena.
In the world of business & product development, qualitative data analysis methods can work to improve user experiences. Suddenly, you’ve got the opportunity to reach a comprehensive understanding of just what your products mean on the social landscape.
User feedback gets transformed into big-picture knowledge that offers a 360-degree view of how a product performs in the real world. Product teams get a solid, reliable basis on which to make decisions, and guesswork becomes a thing of the past.
Key Principles of Qualitative Data Analysis
Before delving into the various methods of qualitative data analysis, let’s look at the key principles that underpin these analysis techniques. Qualitative data analysis is guided by the following principles:
- Inductive Reasoning: Qualitative research focuses on specific observations and gradually develops broader interpretations and theories. It allows for the discovery of new patterns and relationships through an iterative process of data investigation.
- Contextual Understanding: Qualitative data analysis emphasizes the importance of understanding the research context and the social, cultural, and historical factors that shape it. Context provides meaning and helps researchers identify themes as well as interpret and make sense of the data.
- Subjectivity and Reflexivity: When research is human-led, the researchers acknowledge and critically reflect upon their own beliefs, biases, and experiences throughout the qualitative data analysis process. Where research is AI-driven, humans get a chance to view the actual data each insight is based on and check to see if it makes objective sense.
- Active Engagement: A qualitative data analysis method is an active and dynamic process that involves constant engagement with the data. Thematic analysis works most effectively as an ongoing process, thoroughly examining and interpreting all available data, while continually questioning and refining the research questions and analysis as new data points are added.
Inductive reasoning is a fundamental principle of qualitative data analysis. It allows researchers to start with specific observations and gradually develop broader interpretations and theories. Through this iterative process of data investigation, new patterns and relationships can be discovered. When you’ve got AI-driven data analysis software, this inductive reasoning is going on under the hood.
Contextual understanding is another key principle of the qualitative analysis process. It emphasizes the importance of understanding the research context and the social, cultural, and historical factors that shape it.
By considering the context when analyzing qualitative data, researchers can gain a deeper understanding of the data and interpret it more accurately. Well-designed thematic analysis software has this built in.
Subjectivity and reflexivity are essential principles in qualitative data analysis. Qualitative data analysis research must be repeatable if it is to be relied on, and there should always be ways to check just what qualitative feedback particular trends and insights come from. When qualitative data analysis is done right, transparency and rigor can be maintained throughout the process, from the initial selection of research questions and gathering of raw data to final analysis techniques.
Active engagement is a crucial aspect of qualitative feedback interpretation. It involves constant engagement with the data, as researchers thoroughly examine and interpret it. This active and dynamic process allows researchers to continually question and refine their qualitative analysis, ensuring a comprehensive understanding of the data.
Different Qualitative Data Analysis Methods
Just how does qualitative analysis work out in practice? In this article, we will explore five commonly used qualitative analysis methods: content analysis, narrative analysis, discourse analysis, grounded theory, and thematic analysis.
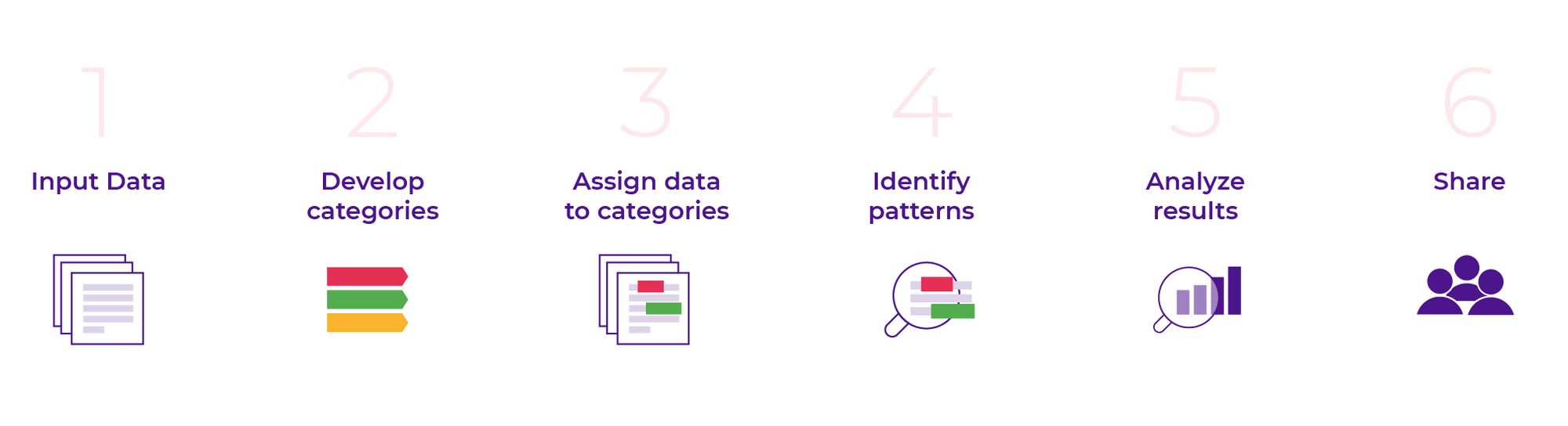
Content Analysis
Content analysis is a systematic and objective approach to analyzing data by categorizing, coding, and quantifying specific words, themes, or concepts within a text. It involves identifying patterns, frequencies, and relationships in the content, which can be textual, visual, or auditory.
Researchers can employ content analysis techniques to examine interviews, focus group discussions, newspaper articles, social media posts, and other forms of textual data. By assigning codes to different segments of the text, researchers can identify recurring themes, sentiments, or messages.
This same qualitative data analysis approach can be used by CX and product teams to analyze customer feedback or support tickets.
For example, in an analysis of public response to a new product, a PX team might use content analysis to analyze social media posts discussing the topic.
By categorizing the posts based on their stance (e.g., positive, negative, neutral) and identifying recurring themes (e.g., user experience, look and feel), a company could gain insights into the dominant narratives and public perceptions surrounding the product launch.
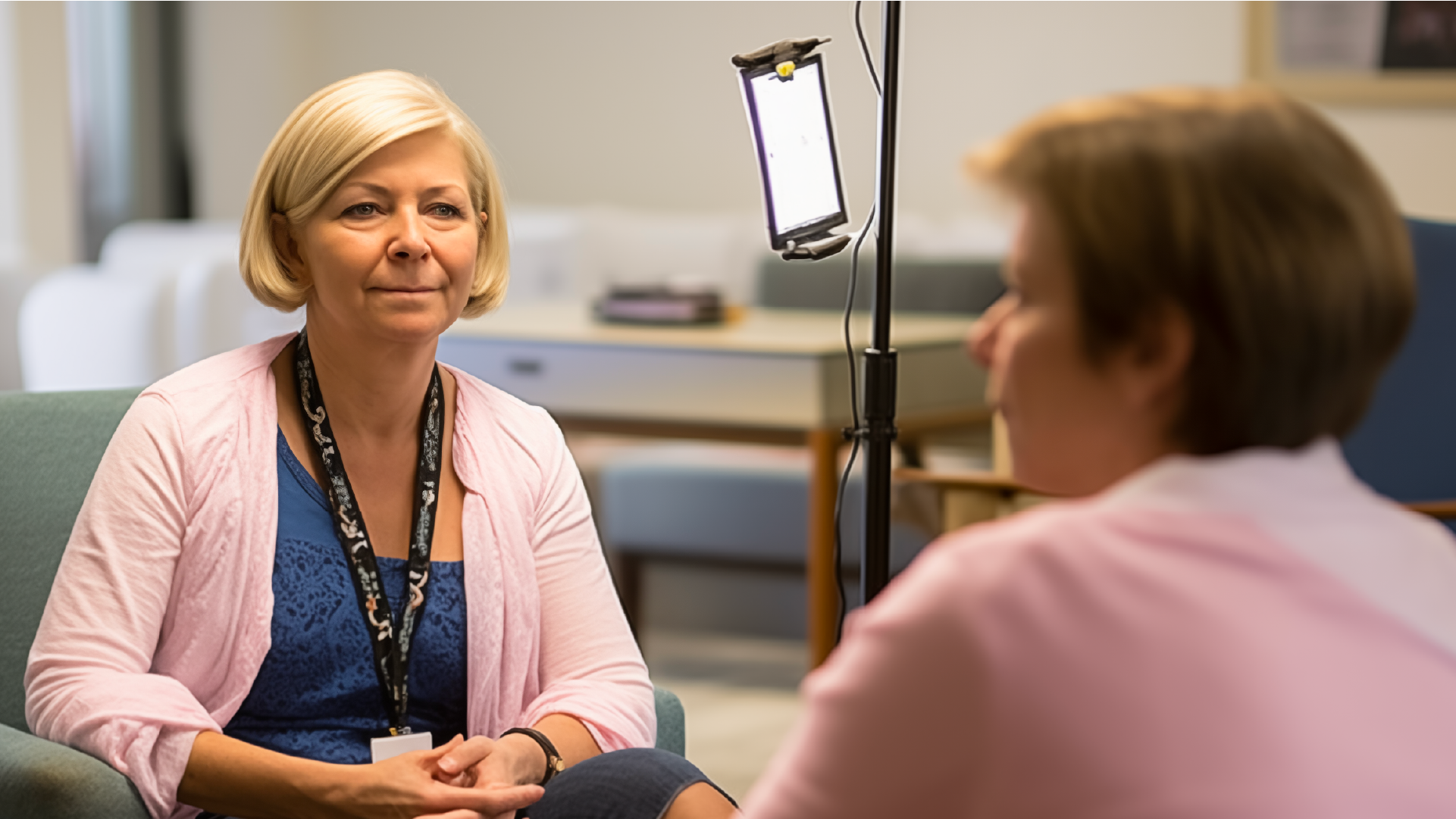
Narrative Analysis
Narrative analysis focuses on interpreting and understanding the stories and personal narratives shared by individuals. Researchers analyze the structure, content, and meaning of these narratives to gain insights into how individuals make sense of their experiences, construct identities, and communicate their perspectives.
Through narrative analysis techniques, qualitative researchers explore the plot, characters, setting, and themes within a narrative. They examine how the narrator constructs meaning, conveys emotions, and positions themselves within the story.
This same narrative analysis method is often used in psychology, sociology, and anthropology to understand identity formation, life histories, and personal narratives. It can be used in a business setting to analyze long-form responses and user interviews or descriptions of user behavior.
For instance, in a study on the experiences of cancer survivors, researchers may conduct narrative analysis on interviews with survivors. By examining the narratives, researchers can identify common themes such as coping strategies, support systems, and personal growth.
This qualitative analysis process can provide valuable insights into the lived experiences of cancer survivors and inform interventions and support programs.
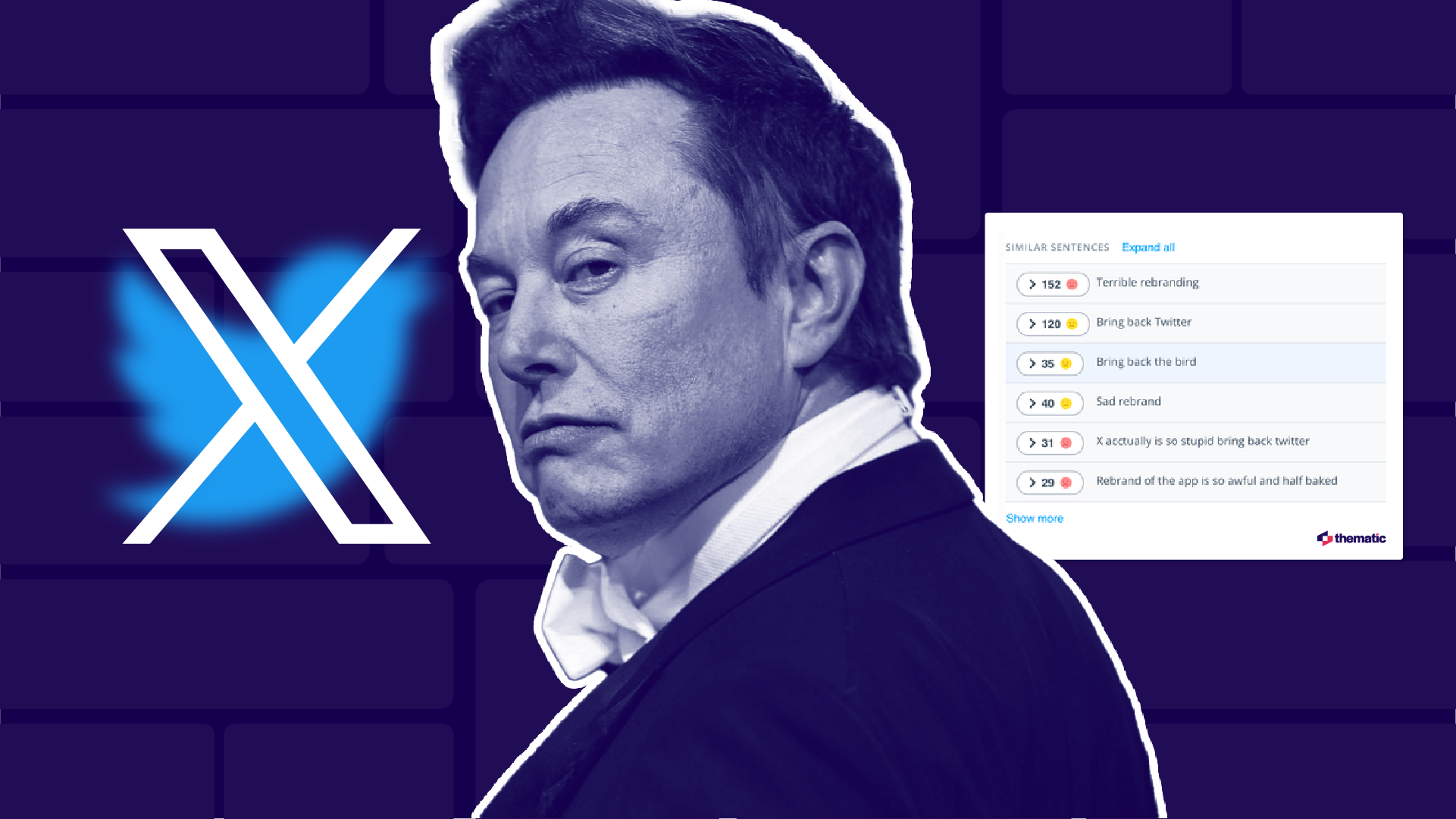
Discourse Analysis
Discourse analysis examines the social, cultural, and power relations that shape language use in different contexts. It focuses on the ways in which language constructs and reflects social reality, identities, and ideologies.
Researchers employing discourse analysis analyze data that includes spoken or written language, including interviews, speeches, media articles, and conversations.
They examine linguistic features such as metaphors, power dynamics, framing, and silences to uncover underlying social structures and processes.
For example, in a study on gender representation in media, researchers may use discourse analysis to analyze television advertisements. By examining the language, visual cues, and narratives used in the advertisements, researchers can identify how gender roles and stereotypes are constructed and reinforced.
It can shed light on the ways in which media perpetuates or challenges societal norms and expectations.
Another example might be using discourse analysis to analyze Tik Tok and YouTube videos to understand the societal responses to a rebranding; for instance, that from Twitter to X. Customer interviews are another good source for this analysis method.
Grounded Theory
Grounded theory is an approach to qualitative analysis that aims to develop theories and concepts grounded in data. It involves iterative data collection and analysis to develop an inductive theory that emerges from the unstructured data itself.
Researchers using grounded theory analyze interviews, observations, and textual data to generate concepts and categories.
These concepts are continually refined and developed through theoretical sampling and constant comparison. Grounded theory analysis is particularly useful when exploring complex social phenomena where existing theories may be limited.
For instance, in a study on the experiences of individuals living with chronic pain, researchers may use grounded theory to analyze interviews with participants. Through iterative analysis, researchers can identify key concepts such as pain management strategies, social support networks, and psychological coping mechanisms.
These concepts can then be used to develop a theoretical framework within grounded theory that captures the multidimensional nature of living with chronic pain.
Although historically grounded theory analysis has been primarily used in the social sciences, grounded theory has also been used successfully for business inquiry.
Thematic Analysis
Thematic analysis is a widely used method in qualitative data analysis that involves identifying, analyzing, and reporting patterns or themes within data. It is a flexible approach that can be applied across a variety of qualitative data, such as interview transcripts, survey responses, and observational notes.
When thematic analysis is done manually, researchers initially familiarize themselves with the raw data, reading through the material multiple times to gain a deep understanding.
Following this, they begin manual coding. The first step is to generate initial codes, which are tags or labels that identify important features of the data relevant to the research question.
These codes are then collated into potential themes, which are broader patterns that emerge across the data set.
Each theme is then reviewed and refined to ensure it accurately represents the coded data and the overall data set. The final step involves defining and naming the themes, during which researchers provide detailed analysis, including how themes relate to each other and to the research question.
Sound complicated? The great news is that advances in artificial intelligence mean we no longer have to do all that by hand.
Thematic analysis software can process thousands of pieces of consumer feedback in a matter of minutes, providing a user-friendly view of the themes and trends in the customer data pool.
What’s more, this type of software can be programmed to do content analysis, discourse analysis, and narrative analysis at the same time. The best comprehensive business solution for thematic analysis today is Thematic; a comprehensive feedback analysis that is designed for customer-centric businesses. It makes qualitative user analysis accessible to anyone, and is able to process feedback at scale.
Across disciplines, thematic analysis is particularly valued for its ability to provide a rich and detailed, yet complex account of data. It's a method that is accessible to researchers across different levels of qualitative research experience and can be applied to a variety of theoretical and epistemological approaches, making it a versatile tool in qualitative work.
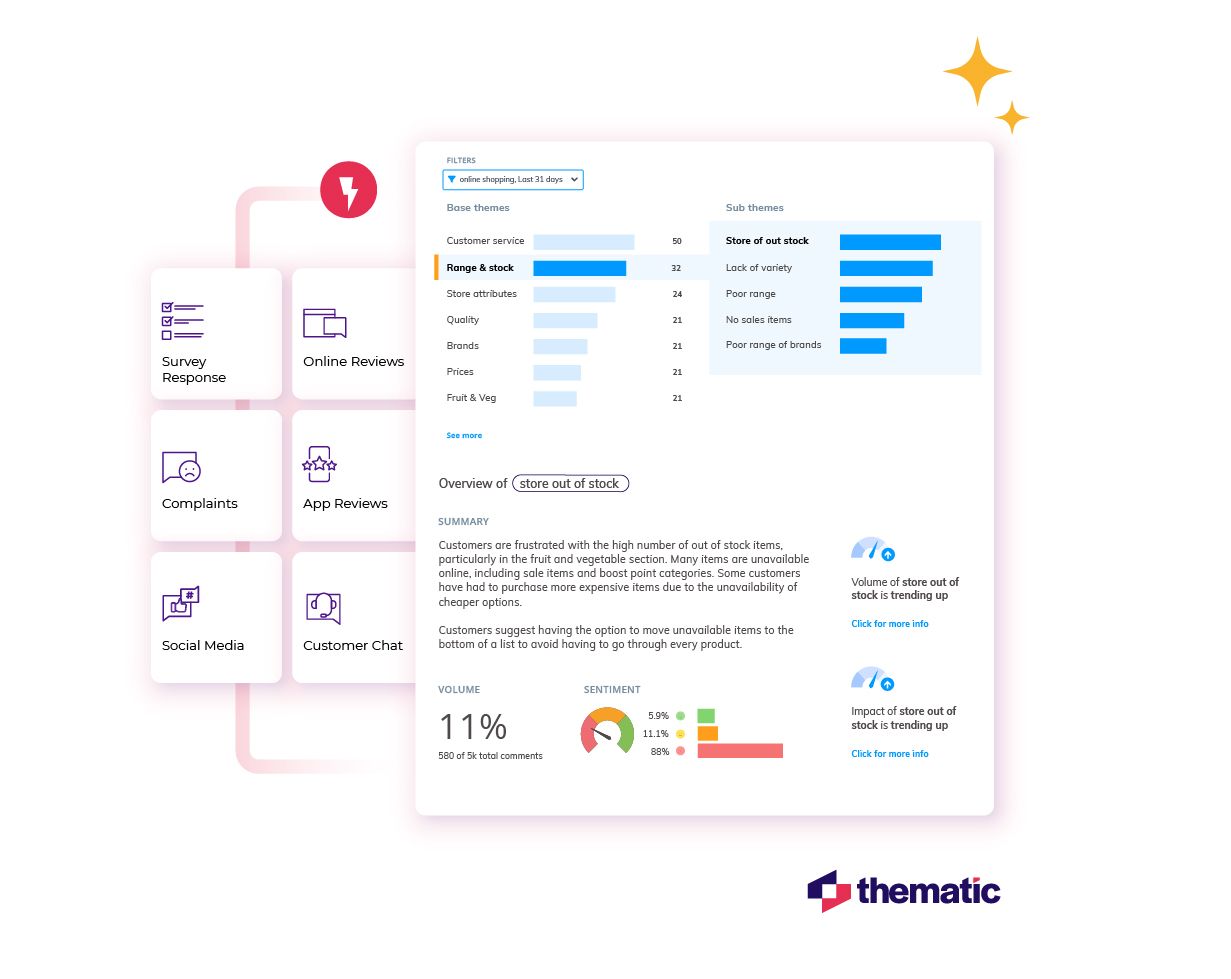
Steps in Qualitative Data Analysis
Data Collection
Data collection is the initial phase of qualitative research and data analysis. It involves selecting appropriate methods to gather data such as interviews, observations, focus groups, or archival research.
Researchers may employ various techniques to collect data. These can include developing interview protocols, conducting observations, or collecting data using audio-visual recording devices.
They may need to consider ethical considerations, ensure informed consent, and establish rapport with participants to obtain rich and reliable data. The goal is to gather qualitative data that is relevant, comprehensive, and representative of the research topic.
Qualitative research questions can be more open-ended than those used for gathering quantitative data, and the research findings have the potential to be far more extensive.
In a business context, much of the work is done for you by customers who provide feedback in reviews, on support tickets, and on social media. Customer interviews are another possible source of rich data.
Data Coding
Data coding is the process of categorizing and organizing qualitative data into meaningful segments. When this is done manually, researchers assign codes to different parts of the data based on the emerging patterns, themes, or concepts identified during analysis. This coding process helps researchers manage and make sense of large amounts of qualitative data.
There are different types of codes used in analyzing raw data, including descriptive codes, interpretive codes, and conceptual codes.
Descriptive codes capture the content and surface-level meaning of all the data, while interpretive codes delve deeper into the underlying meanings and interpretations. A conceptual coding system further abstracts the research data by identifying broader concepts or theories.
Data Interpretation
Data interpretation involves making sense of the coded data and exploring the relationships, themes, and patterns that emerge from the analysis. Researchers critically examine the data, compare different codes, and then identify themes and connections between categories and concepts.
During data interpretation, researchers may engage in constant comparison, where they continually compare new data to existing codes and categories. This iterative process helps refine the analysis and identify theoretical insights.
It involves synthesizing the findings of qualitative and quantitative data and crafting a narrative that presents a comprehensive understanding of the research phenomenon.
Both data coding and data interpretation can be done by your qualitative analytics software, either in a research or business setting. In a corporate setting, CX /PX teams and customer service can then use information gained through the data interpretation step to drive favourable outcomes.
Performing Qualitative Data Analysis with Generative AI and LLM
Running manual grounded theory analysis or content analysis on a large amount of consumer feedback has never been a practical option. But that doesn’t mean qualitative research doesn’t make sense in a business context.
Generative AI, based on large language models (LLMs) can work with qualitative data at scale, analyze it, and derive the themes, connections and insights that can inform business decisions.
An LLM is a powerful machine learning model, based on deep learning and neural networks. It’s able to process and identify the complex relationships in natural language, and it can also understand user questions and moods and even generate text.
A natural languague processing LLM, trained on huge amounts of text data, could do all the work of a QDA researcher with the added benefits of easily verifiable, repeatable results.
Companies with extensive in-house talent may be able to build an in-house AI engine to analyze customer feedback and make sense of it— on a small scale. Those who are serious about getting real insights, though, will want to go with professional tools that have been trained on massive amounts of data and give reliable, dependable results.
Thematic is probably the best example of such a tool. Built to make sense of any amount of feedback data, it works in a highly transparent way that will leave you confident in every insight you derive.
It’s also incredibly user-friendly, with helpful visualizations and an easy-to-use dashboard that enables you to keep constant tabs on exactly what your users feel about the company. It’s never been easier to transform your user experience.
Modern Methods of Qualitative Data Analysis in Action: A Case Study
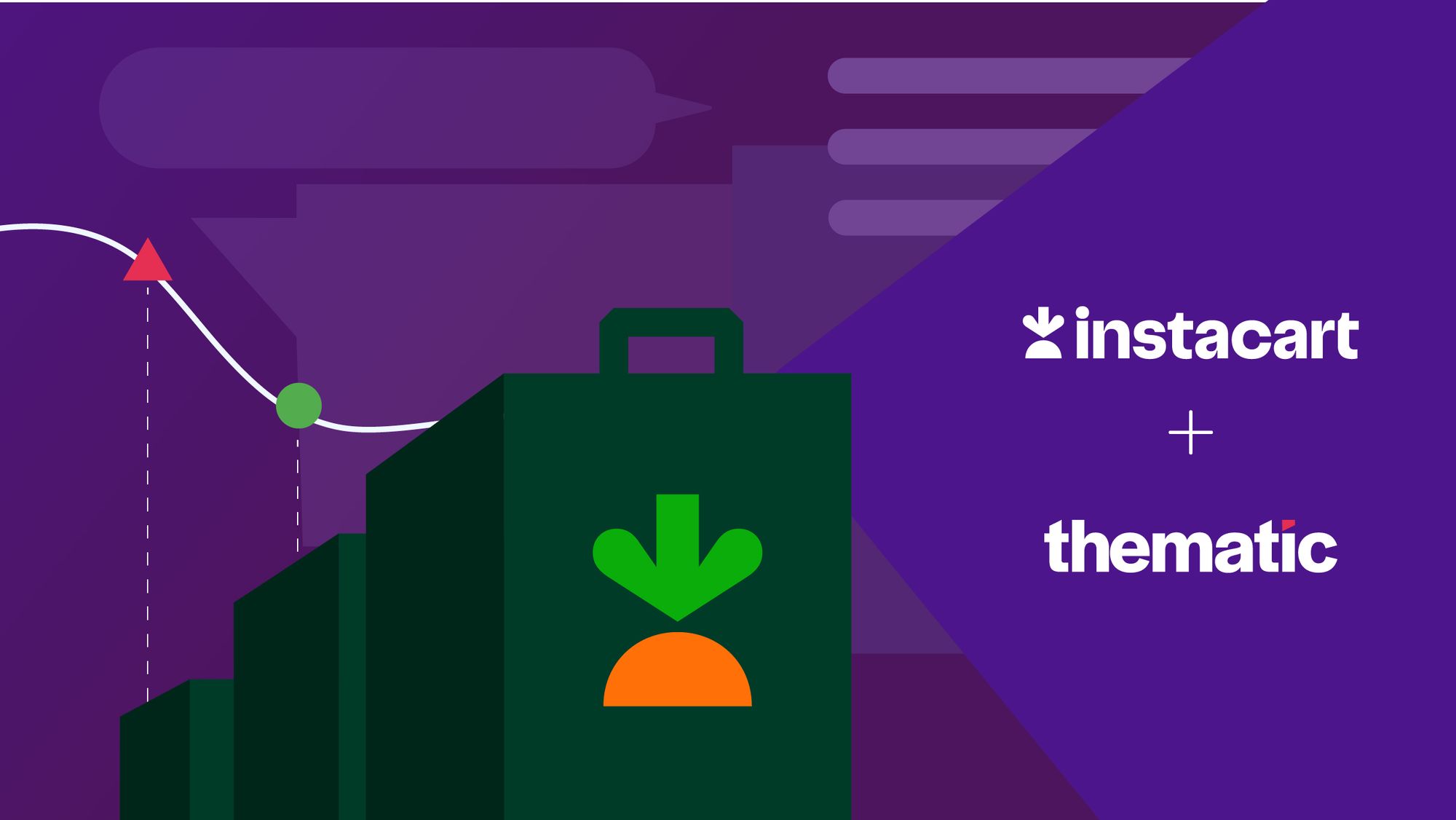
Instacart is one example of a company that discovered the power of qualitative data analysis. This company has 10 million end users, 500,000 personal shoppers, and more than 40,000 retailers. Processing all this qualitative data the traditional way would have been impossible, but Ant Marty, product operations team manager, found a method that worked.
Plugging data from the app into Thematic, she got real time information on everything happening among those millions of users: trends, themes, and deep understanding of what mattered to the people who made the company run.
Data collection is easy when you have an app with numerous feedback collection options. Data coding is automated by Thematic. And Thematic makes the first move in interpretation as well, providing insights that can be transformed by product teams into action plans and even a long-term vision.
Challenges Facing Qualitative Data Analysis Methods
Ensuring Data Validity and Reliability
One of the main challenges to a qualitative approach is ensuring the validity and reliability of the findings. Validity refers to the accuracy, truthfulness, and credibility of the data collected and analysis, while reliability refers to the consistency and replicability of the research process and findings.
Researchers address these challenges by employing rigorous data collection methods, ensuring data saturation, conducting member checks, and establishing inter-rater reliability. They also maintain reflexivity by critically reflecting on their assumptions, biases, and interpretations throughout the analysis process.
If you are a business using software to conduct qualitative research, your data validation check may be somewhat different, but it’s just as important. Some software, like Thematic, has validation built in, and the whole process is so transparent you can easily check and double-check where each insight comes from.
With other software options, you may have to run manual checks to ensure every piece of information provided has a firm basis.
Dealing with Subjectivity and Bias
Subjectivity and bias used to be considered inherent to qualitative research methods due to the interpretive nature of the process. Researchers bring their own perspectives, beliefs, and experiences, which can influence the analysis and interpretations.
To mitigate subjectivity and bias, researchers maintain transparency in their analytical processes by documenting their decision-making, providing detailed justifications for their interpretations, and engaging in peer debriefing and member checking. Using multiple researchers or an expert panel can also increase the credibility and reliability of the analysis.
Another way to decrease subjectivity is through thematic analysis software, which produces results that are repeatable and verifiable.
When it is all said and done, qualitative analysis offers a powerful and nuanced examination of human experiences and social phenomena. By employing diverse methods, adhering to key principles, and addressing potential limitations, researchers can harness the full potential of qualitative data to uncover rich insights and contribute to the advancement of knowledge.
Benefits of Qualitative Data Analysis Methods
Rich, In-Depth Insights
A primary benefit of qualitative research techniques is their ability to provide rich, in-depth insights into complex phenomena. These methods delve deeply into human experiences, emotions, beliefs, and behaviors, offering a comprehensive understanding that is often unattainable through quantitative methods.
By exploring the nuances and subtleties of social interactions and personal experiences, qualitative analysis can uncover the layers of meaning that underpin human behavior. This depth of understanding is particularly valuable in fields like psychology, sociology, and anthropology, where the intricacies of human experience are central to the research question.
It is even more important for customer-focused businesses and enables them to create a product and a CX that meets their customer’s needs and desires. Quantitative analysis can provide a one-dimensional understanding of user behavior based on quantitative data, but when analysing qualitative data you get the why to every what.
Flexibility and Contextual Understanding
Another significant advantage of these analysis techniques is their inherent flexibility and capacity to provide contextual understanding. Unlike quantitative research, which relies on rigid structures and predefined hypotheses, qualitative research is adaptable to the evolving nature of the study.
This flexibility allows researchers to explore unexpected themes and patterns that emerge during the data collection process. Qualitative analysis is how businesses like Atlassian have created infinite customer feedback loops and powered their own infinitely evolving products.
Additionally, qualitative methods are sensitive to the context in which the data is collected, acknowledging and incorporating the environmental, cultural, and social factors that influence the data. The context-rich approach used to collect qualitative data ensures a more holistic understanding of the subject matter, making it particularly useful in cross-cultural studies, community research, and exploratory investigations.
Your product may have global reach, and users in different areas may interact with it in different ways– but qualitative techniques can take all that into account.
This considered, it should be no surprise that qualitative analysis techniques have become powerful tools for researchers seeking to understand the complexities of human behavior and social phenomena. Their ability to provide depth, context, and rich narrative data makes them indispensable tools in the arsenal of social science research, and there’s no better way to gain solid information to guide your business decisions.
Whether you’re a researcher keen on analyzing and interpreting qualitative data or an entrepreneur keen on making your business more customer-centric, this research method is likely to become your next best friend.
If you’re in academia, you may want to do it all manually, and that’s totally okay. But if it’s business intelligence you’re after— try out Thematic. Your future self will thank you, as will everyone else who views the end-of-year reports.
FAQ
What are the five methods to analyze qualitative data?
The five chief methods of qualitative data analysis are:
- Content Analysis
- Narrative Analysis
- Discourse Analysis
- Grounded Theory
- Thematic Analysis
The right analysis method for your use case will depend on what context, your research questions, and the form of data available to you.
What are good sources of data for qualitative data analysis?
In a business context user reviews, support tickets, customer surveys and social media posts are all great sources of data for qualitative analysis. In a research project, gathering qualitative data may mean conducting interviews, surveys, or focus groups.
What are the benefits of qualitative data analysis?
Two big benefits of qualitative data analysis include:
- Rich, in-depth insights
- Flexibility and contextual understanding
In a business context, this translates into a loyal, well-satisfied user base, a successful product, and an upwards-ticking revenue curve. Research objectives for social sciences may include a better understanding of social dynamics or human relations.
What are the challenges of qualitative data analysis?
The two prime challenges of qualitative data analysis techniques are:
- Ensuring data validity and reliability
- Dealing with subjectivity and bias
What is the best tool for qualitative data analysis?
While a number of other options do exist, the best comprehensive software for qualitative data analysis in a business context today is Thematic.