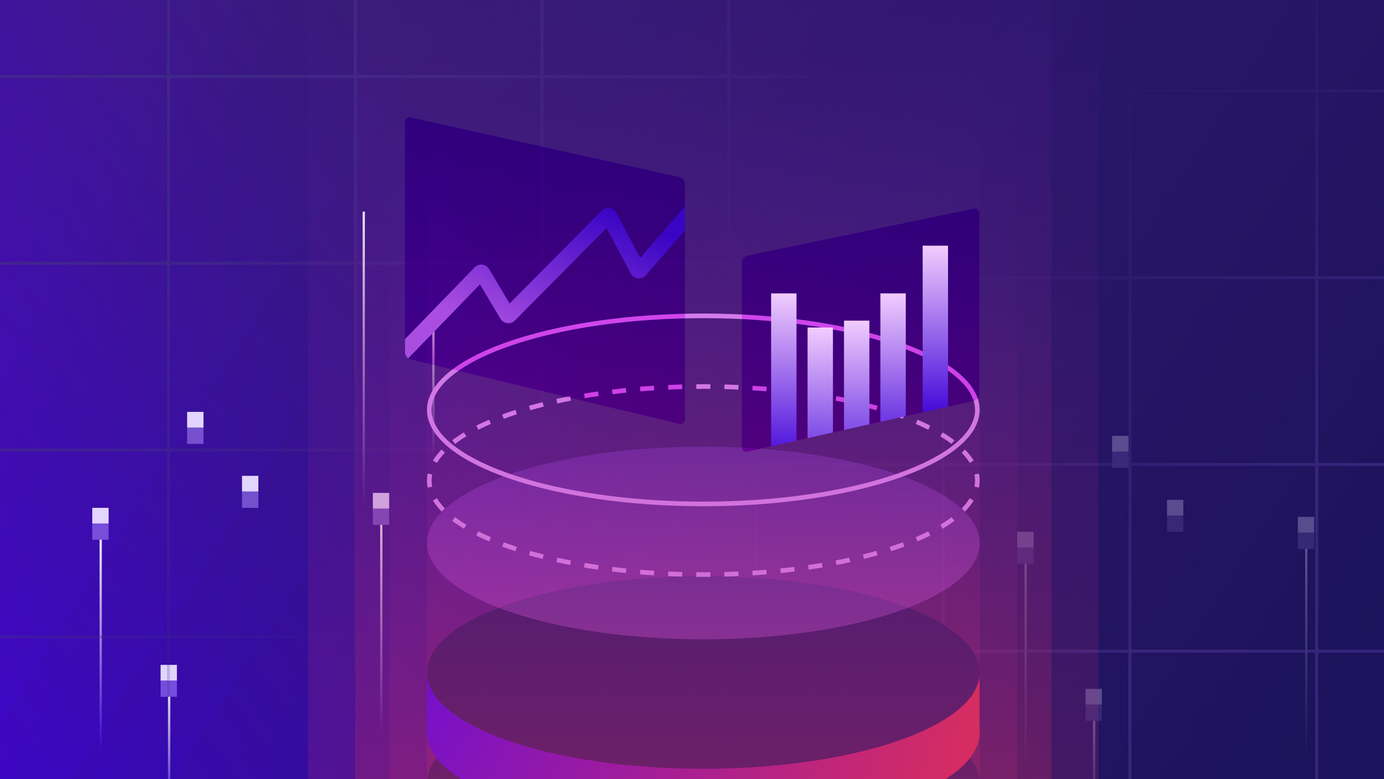
What Is the Modern Data Stack: A Practical Guide for Decision Makers
Explore the modern data stack: cloud-native tools, benefits, and architecture transforming analytics and business decisions.
Imagine you're a business leader swimming in data—customer feedback comments, web analytics, sales figures, support tickets—raw data everywhere, but actionable insights are hard to come by. Many organizations today know data-driven decision making in theory, yet in practice their legacy systems can’t keep up with the speed and complexity needed for great customer experience.
Legacy data stacks were built for a slower era, not for today’s always-on world of big data and real-time demands. In fact, only about 10% of businesses surveyed say they have a truly modern data stack, while nearly half are actively upgrading their data infrastructure. Clearly, there's a huge gap between the data potential and the reality.
The solution? A modern approach that’s modular, cloud-native, and agile enough to turn all that data into value.
So what exactly is the modern data stack, and why is everyone talking about it?
Let's break it down in plain language and explore how it can transform your decision-making.
What Is the Modern Data Stack?
The modern data stack (MDS) is a cloud-native, modular ecosystem of tools and technologies designed to handle the entire data flow from collection to insights.
Unlike old all-in-one systems, the modern stack is like a set of building blocks: you have specialized components for storing, processing, and analyzing data, all working together. It’s essentially a modern data platform that takes your disparate data sources (like databases, applications, and even text feedback) and moves them through a data pipeline toward meaningful analysis.
In simpler terms, the MDS lets you
- ingest and integrate data from anywhere,
- store it efficiently in the cloud,
- transform and clean it,
- then analyze and visualize it to inform decisions.
This spans everything from extraction tools that grab data, to warehouses that act as the data storage layer, to BI dashboards that provide data visualization. As such, decision-makers get timely, trustworthy insights instead of waiting weeks for reports.
How Modern Data Stacks Came Into Being
Crucially, the modern data stack evolved because traditional, monolithic data systems just weren’t cutting it.
Older on-premise setups couldn’t scale for big data volumes, and they often forced companies to do batch processing (meaning insights came with a lag). In contrast, the modern stack embraces real-time processing and streaming data, which enables up-to-the-minute analytics. The rise of streaming events, IoT, and the need for instant analytics (think of dashboards updating in real time) pushed this evolution.
Companies also realized they needed to be more data-driven in every decision, and that meant building infrastructure that can handle both structured and unstructured data quickly.
We’ll dive deeper into this evolution in the next section.
For now, know that the MDS is all about agility and flexibility: pick the right tool for each job and integrate them via the cloud. It stands in stark contrast to legacy data stacks, which were often inflexible, closed-off systems.
From Legacy to Modern: The Story of the Data Stack's Evolution
Businesses used to operate in a very different data world.
Imagine a company in the early 2000s: the IT team is hunched over racks of servers humming in a cold, fluorescent-lit data center. Each department—marketing, sales, finance—has its own isolated system. Data is stored on-premises, with massive upfront costs for hardware that might soon become obsolete. Need more storage? Get ready to order new servers and wait weeks for installation.
This was the era of the legacy data stack. It was rigid, heavy, and expensive.
- Every new system or upgrade came with months of lead time and heaps of manual work.
- Reports were generated in batches—maybe weekly, maybe monthly—meaning insights arrived long after opportunities had passed.
- Siloes were everywhere: marketing's CRM didn’t talk to finance's billing system, which didn’t talk to customer support’s ticketing tool.
- Stitching data together meant messy spreadsheets, late nights, and countless headaches.
And when it came to scaling? That was a nightmare. Companies either overpaid for capacity they might never use or hit limits that stifled growth. As new data sources emerged—social media, mobile apps, IoT devices—these old systems simply couldn’t keep up.
The world had changed, but legacy stacks stayed stuck in the past.
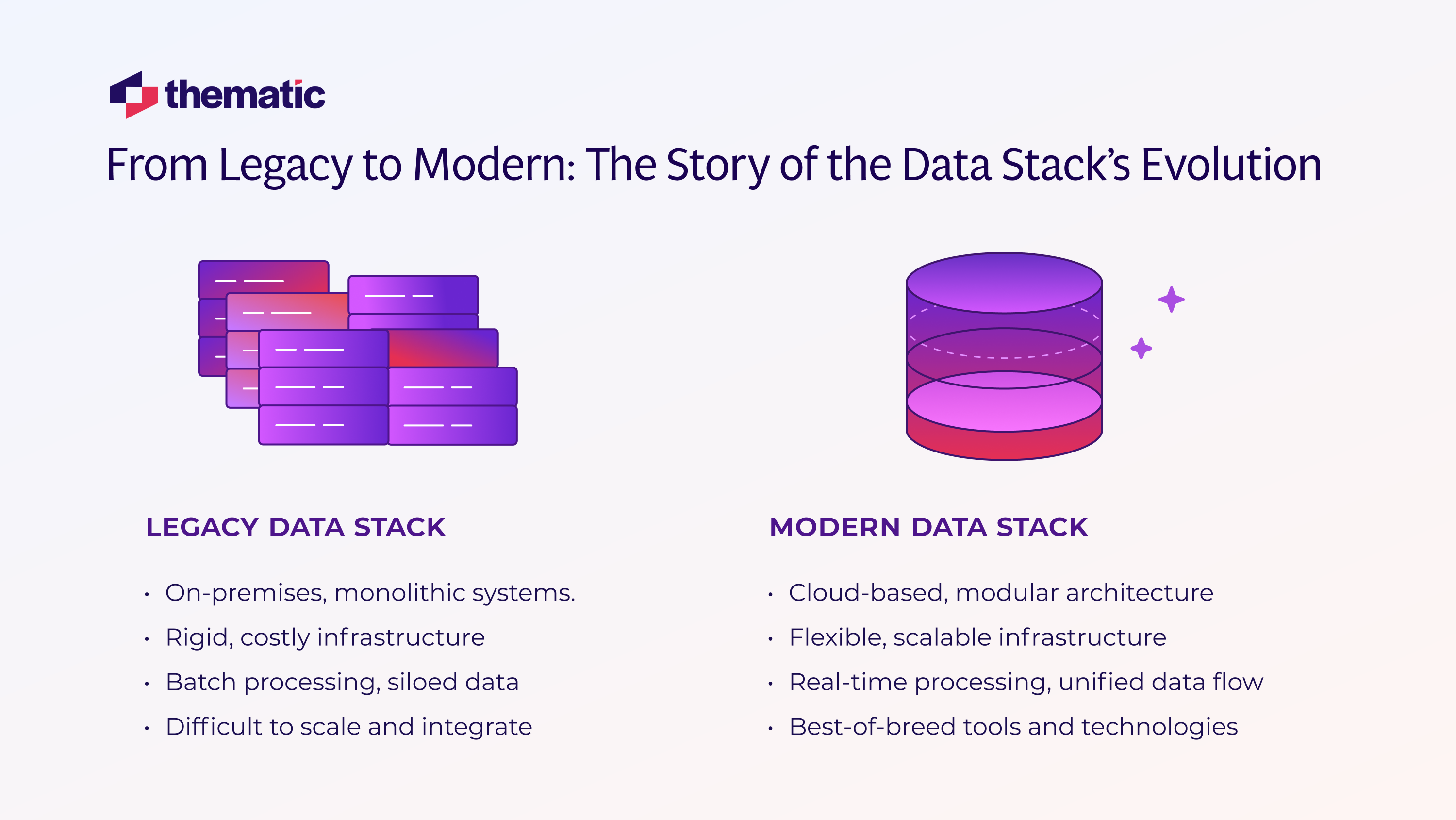
Then Came a Shift: Modern Data Stacks
Enter the modern data stack—a breath of fresh air for data teams everywhere.
Instead of tying companies to physical servers and rigid monoliths, the modern approach embraces the cloud.
- Infrastructure became flexible, modular, and scalable on demand.
- Tools like Snowflake, BigQuery, and Redshift made it possible to store virtually unlimited data without buying a single piece of hardware.
- Ingestion tools like Fivetran and Airbyte automated the tedious process of moving data.
- Transformation tools like dbt empowered teams to clean and model data directly inside the warehouse.
Now, businesses could process and analyze data in near real-time.
- Instead of waiting days for reports, managers could watch dashboards update live.
- Instead of patching together siloed systems they could connect everything through APIs and pipelines, creating a unified data platform that powered smarter, faster decisions.
And the stack was modular by design. Companies could pick and choose the best-in-class tools and technologies for each layer—whether for data storage, data integration, or data visualization. No more one-size-fits-all vendor lock-in. And when a better tool came along? Swapping it out was easier than ever.
Even qualitative data like customer feedback entered the stack more elegantly. Companies began using platforms like Thematic to perform qualitative data analysis, turning messy survey comments into structured insights that flowed into their central data store—giving decision-makers a 360° view of customer sentiment alongside sales figures and behavior trends.
In essence, businesses moved from survival mode—just keeping the lights on—to growth mode—using real-time, trusted insights to stay agile and proactive.
The journey from legacy to modern was a reinvention of how organizations think about, interact with, and act on their data.
Quick Comparison: Legacy vs. Modern Data Stacks
Core Components of the Modern Data Stack
To really understand the power of the modern data stack, it helps to think about it not as a pile of tools, but as a journey your data takes. Each component plays a specific role, helping your data move from raw input to real business insight.
Here’s how the journey unfolds:
1. Data Sources: Where It All Begins
Every journey needs a starting point. In the modern stack, it starts with your data sources—places where information is created.
Think: CRM systems, web analytics platforms, IoT devices, customer feedback forms, or social media streams.
This raw data is full of potential—you just need a way to harness it.
2. Data Ingestion and Integration: Moving Data Seamlessly
Next, we need to bring all that scattered data into one central place.
Tools like Fivetran, Airbyte, and Stitch make this possible through general-purpose data ingestion and integration. They connect to your various sources, extract the data, and load it into your warehouse automatically—keeping your data flow continuous and up-to-date.
For customer feedback specifically, Thematic offer specialized data gathering capabilities. Thematic connects directly to surveys, support platforms, and review channels to ingest unstructured feedback data, centralizing it for further analysis and insight generation.
3. Data Storage: Building a Safe Home
Now that data is flowing in, where does it go?
It lands in your data storage layer—typically a modern cloud warehouse like Snowflake, BigQuery, or Amazon Redshift.
This data store can scale infinitely, hold structured and unstructured information, and let your team access it quickly when they need it.
4. Data Transformation: Cleaning and Preparing for Action
Raw data isn’t always ready for analysis.
That’s where data transformation comes in.
Using tools like dbt or Apache Spark, teams clean, normalize, and shape the data into useful, analysis-ready formats, ensuring that reports and dashboards are built on consistent, reliable information.
When it comes to qualitative data like open-text survey responses or support tickets, Thematic plays a key role in transformation. It uses AI to identify themes, extract sentiment, and turn messy text into structured, analytics-ready data, making it possible to include feedback insights alongside numerical performance metrics.
5. Data Analytics and Visualization: Bringing Data to Life
With clean data in place, it's time to uncover insights.
This is the realm of data analytics and visualization.
Platforms like Looker, Tableau, Power BI, and specialized feedback tools like Thematic turn data into dashboards, reports, and explorations—helping teams discover patterns, spot opportunities, and make data-driven decisions.
6. Data Orchestration: Keeping the System in Sync
Behind the scenes, data orchestration tools like Apache Airflow or Prefect make sure all the moving parts work together.
They schedule workflows, monitor for errors, and automate repetitive tasks—ensuring your entire stack operates like a well-oiled machine.
7. Data Governance and Quality: Protecting Trust
Finally, none of this matters without trustworthy data.
Data governance and quality tools like Atlan or Great Expectations provide data catalogs, security policies, quality checks, and compliance monitoring.
They ensure your data is accurate, your users have the right access, and your business stays compliant with regulations like GDPR.
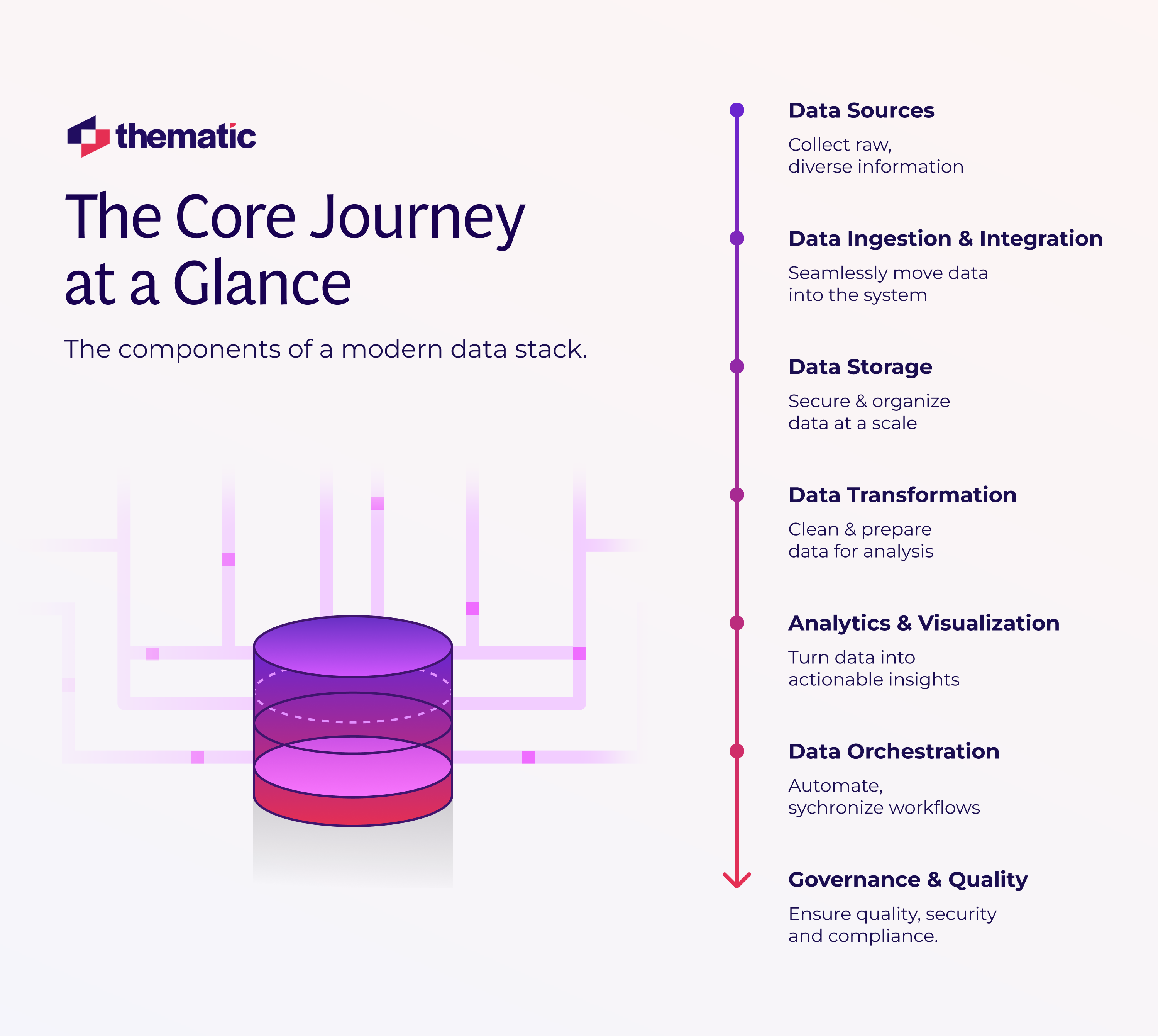
Every one of these components plays a critical part in transforming disconnected bits of information into a powerful engine for growth. Together, they make up the true magic of the modern data stack.
Why Adopting Modern Data Stacks for Business Makes Sense
So, what are the benefits of using a modern data stack for your business? Why go through the trouble of updating tools and retraining teams? Simply put, a modern data stack can give your organization superpowers when it comes to using data.
Here are some of the big advantages decision-makers care about:
Scalability Without Limits
Imagine your company triples its customer base overnight. In a legacy system, that would be a nightmare—servers crashing, engineers scrambling to expand storage, delayed insights everywhere.
But with a modern stack? You barely blink.
Cloud-based storage solutions grow with you. Processing power scales on demand. Whether you're handling ten thousand transactions or ten million, your systems keep pace without needing a massive infrastructure overhaul.
No more choosing between keeping all your valuable raw data or deleting chunks to make room. With the MDS, you get to keep everything—and keep moving forward.
Flexibility to Evolve and Adapt
The market changes fast. New tools pop up. New needs emerge. The beauty of the modern data stack is that it's modular by design.
Want to plug in a cutting-edge analytics tool? Need to swap out your cloud provider? No problem.
You're not locked into a single vendor or trapped by outdated platforms. You can build the best mix of tools and technologies for your needs—today and tomorrow.
And it’s not just about structured tables anymore. Whether it's transaction data, text feedback, sensor logs, or images, the modern stack supports all kinds of data sources—including the ability to automate processes like coding qualitative data—giving you the freedom to innovate without technical handcuffs.
Cost-Effectiveness That Makes CFOs Smile
Traditional IT setups weren’t just slow—they were expensive. Big upfront investments. Over-provisioned systems "just in case" of growth that might never come.
In contrast, the modern stack runs on pay-as-you-go models.
You only pay for what you actually use, scaling up or down as needed. Plus, better automation and integration mean your teams can manage more with less manual work—freeing up valuable time and resources.
The result? Lower infrastructure costs and faster time to insight. That’s not just an IT win—that's a competitive advantage that drives real revenue growth.
Real-Time Insights, Real-Time Action
Data doesn’t wait anymore. Neither should you.
Modern data stacks are built for real-time or near-real-time insights. Instead of getting yesterday’s sales figures at noon today, you can see how today's sales are trending by the hour. Instead of finding out about customer complaints after they snowball into churn, you can spot sentiment shifts as they happen.
For example, a finance company using a modern stack detected fraudulent transactions in real time—not hours later after batch reports rolled in. That kind of speed isn’t just nice—it’s a competitive weapon.
Collaboration Across the Organization
The modern stack doesn’t just empower analysts.
It empowers everyone.
VoC teams, for instance, can use modern data stacks to combine structured data like NPS scores with unstructured feedback, gaining a richer view of customer experience trends.
Because data is centralized and tools are easier to use, people across marketing, sales, product, and operations can explore insights on their own—without filing endless requests with the BI team.
Data democratization breaks down silos. Instead of isolated teams guarding their spreadsheets, everyone taps into the same single source of truth.
Modern data visualization tools even let teams comment, collaborate, and build shared dashboards, making insights a living conversation rather than a static report.
When decisions are based on shared, trusted data, your entire company moves faster—and moves together.
Voice of Customer Made Easy:
The Professionals’ VoC Handbook
Written by a CX expert, this handbook simplifies VoC strategies and helps you get the most from customer feedback.
What's inside:
- Easy-to-follow VoC strategies
- Tips for analyzing feedback
- Plus: Bonus VoC checklist
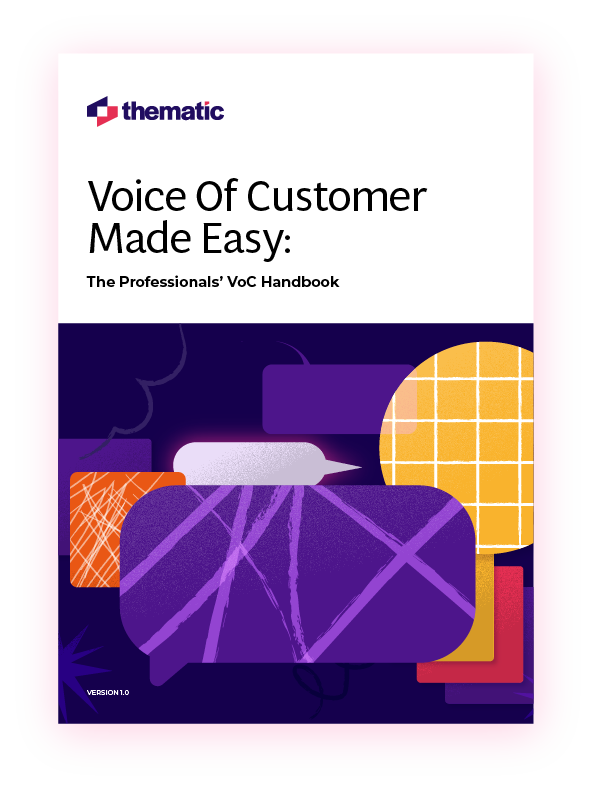
Trust Built on Data Quality
Of course, none of this matters if the data can't be trusted.
One of the biggest hidden advantages of the modern data stack is the way it bakes in data governance and quality.
- Automated validation checks catch errors before they pollute your reports.
- Clear data lineage shows exactly where numbers came from.
- Access controls ensure sensitive information stays protected.
Remember those meetings where people argued over whose spreadsheet was "more correct"?
With a modern stack, those debates disappear—replaced by shared confidence in a single version of the truth.
And when people trust the data, they use it more—which creates a virtuous cycle where insights deepen, decisions get sharper, and business outcomes improve.
How Companies Are Winning With the Modern Data Stack
The modern data stack (MDS) might sound abstract until you see it in action. So, how are organizations leveraging MDS for tangible results? Let's explore its applications across different industries.
Retail: Real-Time Inventory and Personalized Marketing
Retailers like Walmart are transforming operations with MDS by integrating data from point-of-sale systems, online transactions, and customer feedback. This integration enables real-time inventory management, ensuring popular items are restocked promptly, and facilitates personalized marketing campaigns that resonate with individual customer preferences.
Instacart, a leading grocery delivery platform, employs a modern data stack to unify diverse data sources, including customer feedback analyzed through Thematic. This approach provides a holistic view of the customer experience, allowing Instacart to identify and address issues across its consumer base, personal shoppers, retailers, and advertisers. By integrating qualitative feedback into their data ecosystem, Instacart can make informed decisions to enhance service quality and customer satisfaction.
Healthcare: Unified Patient Data for Better Care Coordination
Healthcare providers deal with vast amounts of data from electronic health records, lab results, imaging, and more. Traditionally, this data was siloed, making it challenging to get a comprehensive view of a patient's history.
With a modern data stack, healthcare organizations can unify these disparate data sources, providing clinicians with real-time access to critical patient information. For instance, when a patient arrives at the emergency room, an integrated MDS can immediately retrieve their medical history, recent lab tests, and data from wearables, alerting the care team to vital information like blood pressure trends or medication allergies.
Beyond individual care, MDS facilitates broader public health initiatives. Healthcare networks can analyze aggregated patient data to identify trends and improve services. For example, by compiling data from multiple clinics into a cloud data warehouse, a provider might discover that a specific demographic is underutilizing preventive services, prompting targeted outreach programs. Additionally, integrating data might reveal that patients using mobile apps for symptom reporting experience fewer hospital readmissions, supporting further investment in telehealth tools.
Implementing MDS also supports compliance with regulations like HIPAA by enabling strict data governance, access controls, and anonymization where necessary.
Finance: Real-Time Insights and Customer-Centric Strategies
In the financial sector, real-time data processing is crucial. Banks and financial institutions employ MDS to monitor transactions as they happen, swiftly identifying and preventing fraudulent activities by analyzing patterns and anomalies in real-time, thus safeguarding both the institution and its customers.
Similarly, LendingTree, a prominent online loan marketplace, leverages MDS to enhance customer experience and operational efficiency. By integrating Thematic into their data ecosystem, LendingTree automated the analysis of vast amounts of unstructured customer feedback. This integration enabled real-time insights into customer needs and preferences, allowing for automated theme detection and sentiment analysis.
Thematic's dashboards and reports provided product and customer experience teams with a high-level view of Net Promoter Score (NPS) drivers, facilitating swift responses to emerging customer issues and informing product development strategies.
This centralized approach to data allowed various departments, including product, marketing, and customer service, to collaborate more effectively, enhancing the overall customer experience.
Technology: Real-Time Product Insights and Smarter Development
Tech companies, especially SaaS providers, generate massive amounts of product usage data every day. Modern data stacks allow them to capture and analyze this firehose of information to guide smarter product decisions.
By funneling user events into a cloud data warehouse, companies can identify which features are popular, which ones are underused, and where friction points exist. For example, SolidWorks used a modern data stack to analyze over one terabyte of usage data daily, clustering users into behavior segments and tailoring feature improvements.
Companies like Netflix and Airbnb are renowned for their data-driven product decisions, running numerous A/B tests and experiments. Their modern data infrastructure allows product managers to get experiment results in real time and decide quickly whether a change made things better or worse.
The result: faster iteration cycles, better product-market fit, and development strategies that are guided by real-time insights, not guesswork.
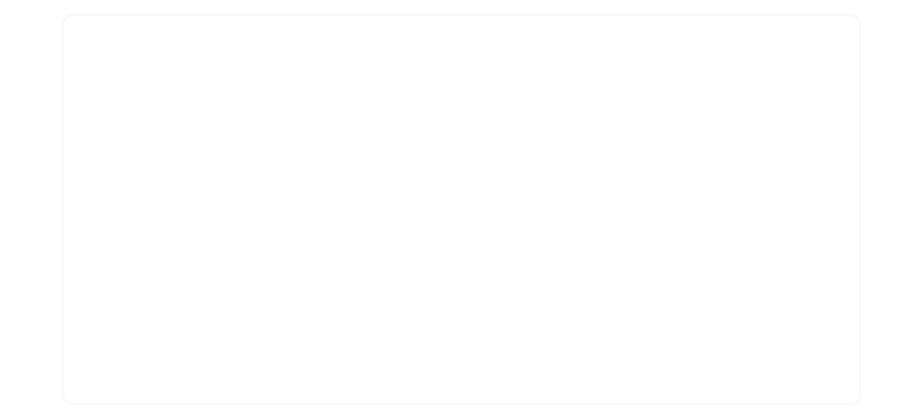
Thematic
AI-powered software to transform qualitative data into powerful insights that drive decision making.
What Could Go Wrong? (And How to Get It Right)
Adopting a modern data stack isn’t without its challenges. It’s important to be aware of potential pitfalls so you can navigate them effectively.
Here are some common hurdles companies face with MDS, and tips on how to get it right:
The Mirage of Eliminated Silos
Wait, didn't we say the modern stack breaks down silos? It does, but only if implemented properly. One pitfall is when departments adopt different tools that aren't well integrated (e.g., marketing sets up their own cloud database separate from the central warehouse). You can end up with mini-silos in the cloud.
How to get it right:
- Establish a company-wide data architecture plan.
- Involve all stakeholders when designing the stack so that sources are feeding into a single data platform where appropriate.
- Encourage a culture of sharing data—break down not just technical silos, but cultural ones too.
- Make sure there's executive buy-in to promote cross-department integration.
- Use data catalog tools to increase visibility into what data exists.
- Don’t forget unstructured data (text, voice, etc.)—have a plan to integrate it using NLP (or the more advanced models like LLM) in tools like Thematic, using AI to theme qualitative data and transform qualitative feedback into quantifiable insights.
The Skills Gap Chasm
A modern stack can introduce new technologies that your team isn’t familiar with. Tools like dbt or Spark, writing transformation in SQL or Python, managing a cloud warehouse – these might be new skills for an existing BI team or IT staff. There’s also a need for data engineering and sometimes data science skills to fully leverage the stack.
How to get it right:
- Invest in training and/or hiring.
- Create a “Data Center of Excellence” or a similar initiative to upskill your people.
- Start small: roll out the stack to a focused slice of the business first (like marketing analytics) to build team confidence before expanding.
- Pair software engineers with analysts for cross-training and faster learning.
- Bring in a consultant temporarily if needed to bridge the expertise gap.
- Focus on people as much as tech—mastering these tools makes teams more productive and happy in the long run.
The Overabundance of Tools
Paradoxically, with so many great tools available, some companies go overboard and try to adopt too many.
If your stack has dozens of different services, it can become complex to manage. You might have overlapping functionality or data flowing in convoluted ways.
How to get it right:
Simplicity is key.
- Design the minimal viable architecture first.
- Choose one ingestion tool, one primary warehouse, one transformation framework, and one main BI tool—keep it simple and integrated.
- Regularly audit your toolset: if two tools do the same job, consolidate.
- Standardize where possible to avoid needless complexity.
- Leverage managed services instead of self-hosted solutions if overhead becomes a burden.
- Always ask: "What value does this tool add, and is it worth the complexity?"
The Security and Compliance Tightrope
Moving to a modern data stack often means storing critical data in the cloud and making it widely accessible within the company. Without proper controls, you risk data breaches or misuse. Also, regulations (GDPR, CCPA, etc.) require careful handling of personal data.
How to get it right:
- Bake security and governance into your design from the start.
- Use security features from your cloud providers—encryption at rest and in transit, identity and access management, row-level security for sensitive data.
- Set up clear data governance policies: define who can access what explicitly.
- Implement monitoring and alerting for unusual access patterns.
- Conduct regular audits: know where personal data is stored and who has viewed it.
- Build deletion/anonymization processes to comply with "right to be forgotten" requests.
- Assign a data steward or governance lead to actively oversee security and compliance efforts.
- Treat security as a first-class requirement, not an afterthought—trust with your customers depends on it.
- The companies that thrive with a modern data stack don’t just upgrade their tools—they upgrade their approach.
- They pair technology with the right policies, training, and culture to stay agile.
- Start with clear goals ("What do we want to improve with our data?") and use them as your north star.
- Modernize with purpose, not just for the sake of it.
- Do that, and your MDS journey is far more likely to become a success story—not a cautionary tale.
What's Next for the Modern Data Stack Revolution
The modern data stack as we know it today isn’t standing still. Just as it evolved to this point, it continues to grow and adapt to new technology trends. Decision-makers should keep an eye on emerging developments that could shape the future of data strategy.
Here are a few key trends and what's on the horizon:
AI and Machine Learning Integration
We’re now in the era of artificial intelligence, and modern data stacks are increasingly incorporating AI/ML capabilities natively. This means not just bolting on an ML tool, but having ML woven into the stack – from automated anomaly detection in data quality (using AI to flag “this data point looks out of the ordinary”) to AutoML systems that can churn through data and surface patterns on their own.
In the near future, expect automated insights where the data stack doesn’t just show you a dashboard, but also tells you interesting findings (“Hey, these three factors are driving your customer churn, identified via a machine learning model”).
Predictive analytics will become more plug-and-play. Cloud warehouses are already adding ML functions inside them, and BI tools are offering AI-driven explanations for trends. The line between data stack and AI stack is blurring. For example, rather than exporting data to a separate environment for machine learning, companies are doing it directly where the data lives.
This trend means companies will be able to leverage AI even if they don’t have a huge data science team, as the capability is built into the tools.
Data Mesh Architecture
Traditionally, even a modern data stack is often centralized – one big team manages one big warehouse for the whole company.
Data Mesh is an emerging paradigm that decentralizes data ownership to domain teams (e.g., the marketing team manages their part of the data stack, the product team manages theirs) under a federated governance model. It’s like applying microservices thinking to analytics. Each domain provides data as a product for others to use, and there are standards to make it interoperable.
This could be the next step for companies that struggle with one-size-fits-all centralization. With a data mesh, you might have multiple warehouses or lakes, but a unified way of discovering and accessing data. The modern tools would still be in use, just deployed in a more distributed way.
For decision-makers, if your organization is very large or siloed, a data mesh approach might improve scalability of both tech and team structure.
Keep an eye on this if your single data platform is starting to groan under the weight of many teams’ needs. It’s an evolving area, but some big organizations are already experimenting with it to enable more autonomy while maintaining overall data governance.
Serverless and Low-Code Data Processing
As part of simplifying infrastructure, there’s a push toward serverless architectures in the data world. We see more serverless data warehouses and serverless data pipelines where you don’t even think about servers or clusters—just send your query or data and get results. This will likely continue, making the stack easier to manage and more cost-efficient (auto-scaling to zero when not in use, for instance).
Alongside this, low-code or no-code interfaces for data workflows are gaining traction. Imagine building a data pipeline by dragging and dropping components rather than writing a bunch of code. This isn’t to say coding will disappear (SQL and Python aren’t going anywhere soon for advanced use cases), but routine tasks may become automated or simplified.
For a manager, this means your analysts or even savvy business users can do more without heavy IT involvement, accelerating the time to insight even further. The end state might be that interacting with the data stack feels more like using a SaaS application than doing software engineering.
Greater Focus on Data Privacy and Ethics
With regulations tightening and public awareness of data privacy at an all-time high, future data stacks will bake in privacy by design. Techniques like data anonymization, differential privacy, and strict consent management will become standard parts of data pipelines. If your company deals in personal data, expect tools to offer more features that help with compliance automatically (like tagging sensitive data and ensuring it’s handled appropriately).
There’s also a growing ethical dimension: using data and AI responsibly. Future best practices may involve bias audits of datasets and models, transparency logs (where data came from, how it’s been used), and more.
Modern data stacks might integrate “privacy vaults” or similar concepts where sensitive data is kept separate, and only aggregated insights leave the vault. For global companies, keeping data region-specific (to comply with data residency laws) could also influence architecture (multi-region data stacks).
For a decision maker, staying ahead on privacy isn’t just about avoiding fines; it’s about maintaining customer trust. The tools will likely evolve to make this easier, but it will require conscious design decisions on your part, too.
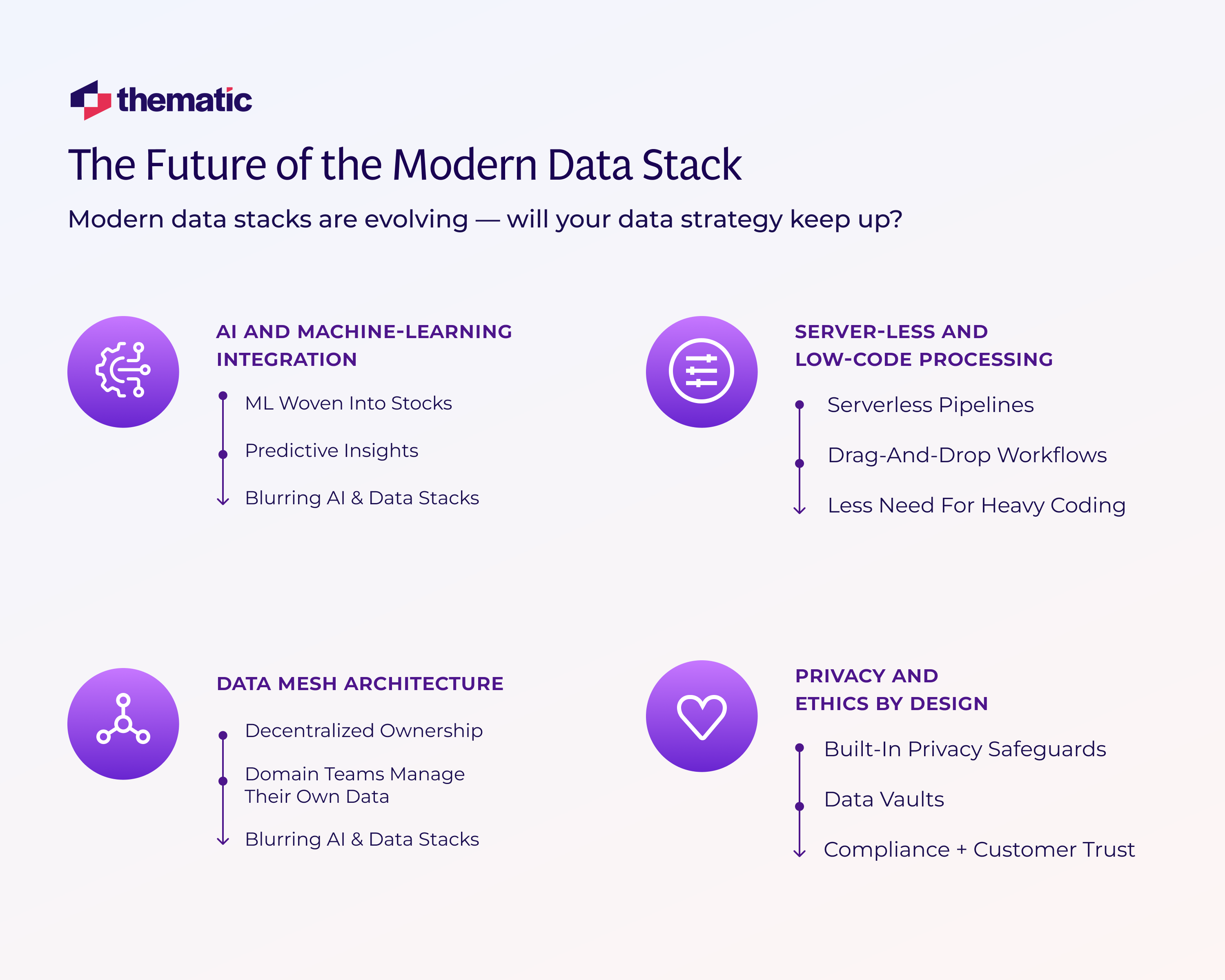
Embracing the Modern Data Stack Advantage
The modern data stack (MDS) is redefining how organizations harness data, transforming it from a static asset into a dynamic engine for innovation. With integrated modular, cloud-native tools, businesses can now ingest, store, transform, and analyze data with unprecedented speed and flexibility.
Teams are now empowered, able to make real-time, data-driven decisions, and foster agility and resilience in a rapidly changing landscape.
As we've explored, adopting an MDS is a strategic shift towards a more responsive and insightful organization. To truly capitalize on this transformation, it's important to manage quantitative data while understanding the nuances of customer feedback.
Ready to unlock deeper insights? Experience how Thematic can seamlessly integrate into your modern data stack, turning unstructured feedback into actionable intelligence. Request a demo of Thematic now and start your journey towards smarter, customer-centric decisions today.
Frequently Asked Questions
1. What exactly makes a data stack “modern”?
A modern data stack is cloud-native, modular, and scalable. Instead of relying on one rigid, all-in-one system, a modern stack uses a combination of specialized tools for different stages of the data journey—like collection, storage, transformation, and analysis. This modular approach makes it easier to handle real-time data, scale quickly, and adapt to changing business needs, compared to the slower, batch-oriented legacy systems.
2. Do I need to replace all my old systems at once to adopt a modern data stack?
Not at all. Many companies evolve gradually toward a modern data stack. You can start by modernizing one part of the process—like moving storage to the cloud or automating data ingestion—and build from there. The modular nature of the modern stack means you can upgrade components over time, at a pace that fits your business goals and resources.
3. Is a modern data stack only for big companies with huge amounts of data?
No—businesses of all sizes can benefit from a modern data stack. While companies like Netflix and Airbnb pioneered its use at scale, today’s cloud tools are highly accessible. Small and mid-sized businesses can use lighter, cost-effective versions of modern stack tools to gain faster insights, improve customer experiences, and scale their operations more easily when growth comes.
4. How does a modern data stack improve customer experience?
By connecting different data sources—like customer feedback, sales data, and product usage—into a single, unified view, a modern data stack lets you spot customer needs, frustrations, and opportunities in real time. Instead of reacting to problems after the fact, you can proactively improve services, personalize experiences, and solve issues before they escalate, all based on trustworthy, up-to-date insights.
Stay up to date with the latest
Join the newsletter to receive the latest updates in your inbox.